Adversarially Regularized U-Net-Based Gans For Facial Attribute Modification And Generation
IEEE ACCESS(2019)
摘要
Modifying and generating facial images with desired attributes are two important and highly related tasks in the field of computer vision. Some current methods can take advantage of their relationship and use a unified model to handle them simultaneously. However, producing high visual quality images on both tasks is still a challenge. To tackle this issue, we propose a novel model called adversarially regularized U-net (ARU-net)-based generative adversarial networks (ARU-GANs). The ARU-net is the major part of the ARU-GAN and is inspired by the design principle of U-net. It uses skip connections to pass different-level features from encoder to decoder, which preserves sufficient attribute-independent details for the modification task. Besides, this U-net-like architecture employs an adversarial regularization term to guide the distribution of latent representation to match the prior distribution, which guarantees to generate meaningful faces from this prior. We also propose a joint training technique for the ARU-GAN, which enables the facial attribute modification and generation tasks to learn together during training. We perform experiments on celebfaces attributes (CelebA) dataset and make visual analysis and quantitative evaluation on both tasks, which demonstrates that our model can successfully produce high visual quality facial images. Also, the results show that learning two tasks jointly can lead to performance improvement compared with learning them individually. At last, we further validate the effectiveness of our method by making an ablation study and experimenting on another dataset.
更多查看译文
关键词
Generative adversarial networks, facial images, skip connections, adversarial regularization
AI 理解论文
溯源树
样例
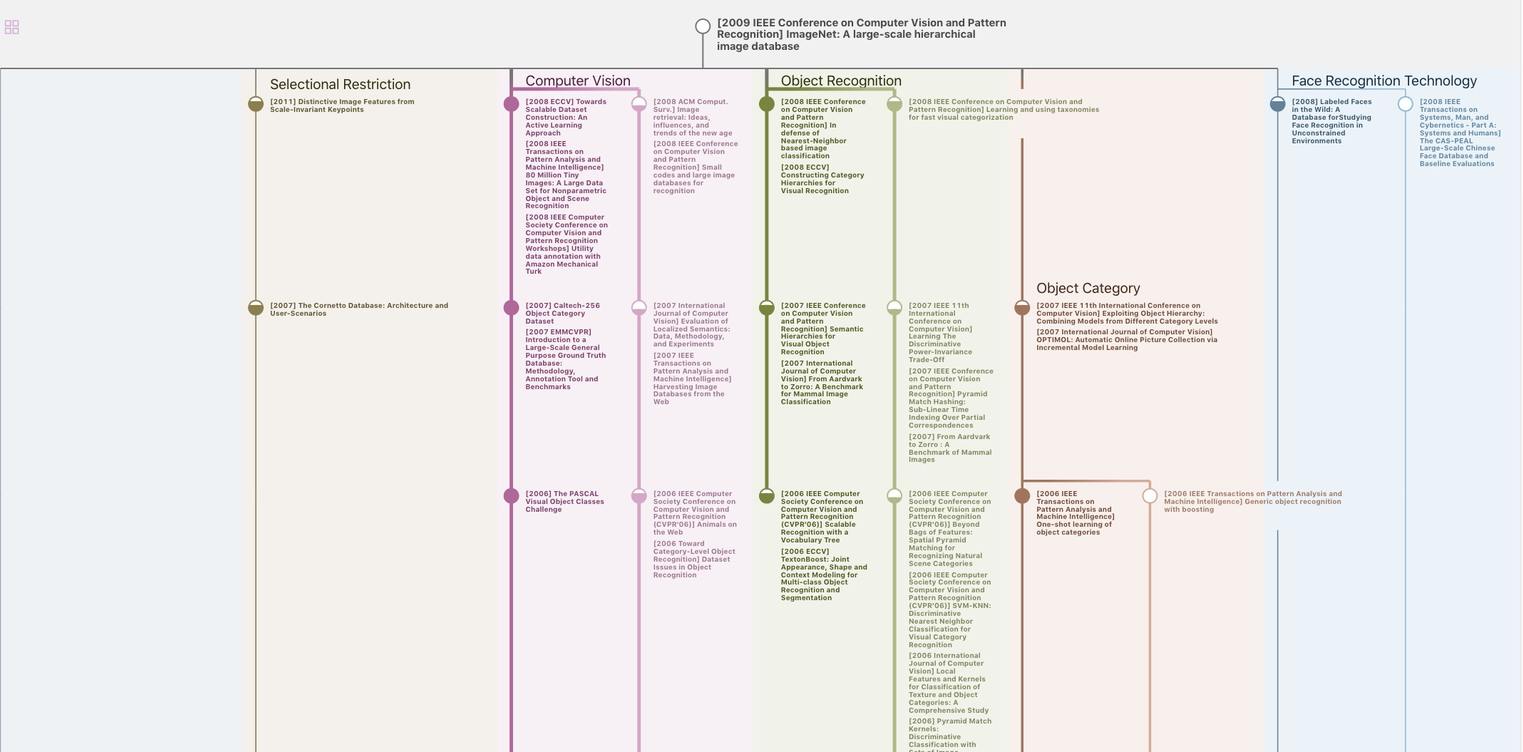
生成溯源树,研究论文发展脉络
Chat Paper
正在生成论文摘要