Geographical Correlation-Based Data Collection for Sensor-Augmented RFID Systems
IEEE Transactions on Mobile Computing(2020)
摘要
This paper studies the practically important problem of data collection for sensor-augmented RFID systems. However, existing RFID data collection protocols suffer from two common limitations: execution time is naturally in proportion to the number of tags, thus they cannot satisfy time-stringent application scenarios; none of them is complaint with the C1G2 standard, thus they cannot be implemented using Commercial-Off-The-Shelf (COTS) RFID tags. To overcome these two limitations, this paper proposes the Geographical correlation-based RF-data Collection (GRC) protocol. GRC is fast because it is able to approximately capture the sensing data of all tags by only actually gathering data from a small set of sampled tags. This is based on the observation from the real-world data set that sensing data has a strong geographical correlation, i.e., data gathered from nearby RFID tags has similar values. In GRC, we use a greedy approach to find the minimum sampling tag set to cover the whole monitoring region such that each un-sampled tag has at least one sampled tag nearby. Then, RFID reader runs the Framed Slotted Aloha (FSA) protocol specified in C1G2 standard to collect sensing data from the sampled tags. For each un-sampled tag, we approximate its sensing data by calculating weight-average of the data collected from its nearby sampled tags, where a faraway sampled tag should be given a small weight, and vice versa. Compared with existing RFID data collection schemes, the advantages of GRC are two-fold: (1) Extensive simulation results demonstrate that the time cost of our GRC scheme is only 1/28~1/3 of the state-of-the-art data collection scheme; (2) GRC is totally complaint with C1G2 standard, thus it can be easily deployed on the COTS RFID tags.
更多查看译文
关键词
Sensor-augmented RFID,sensing data collection,time-efficiency,geographical correlation
AI 理解论文
溯源树
样例
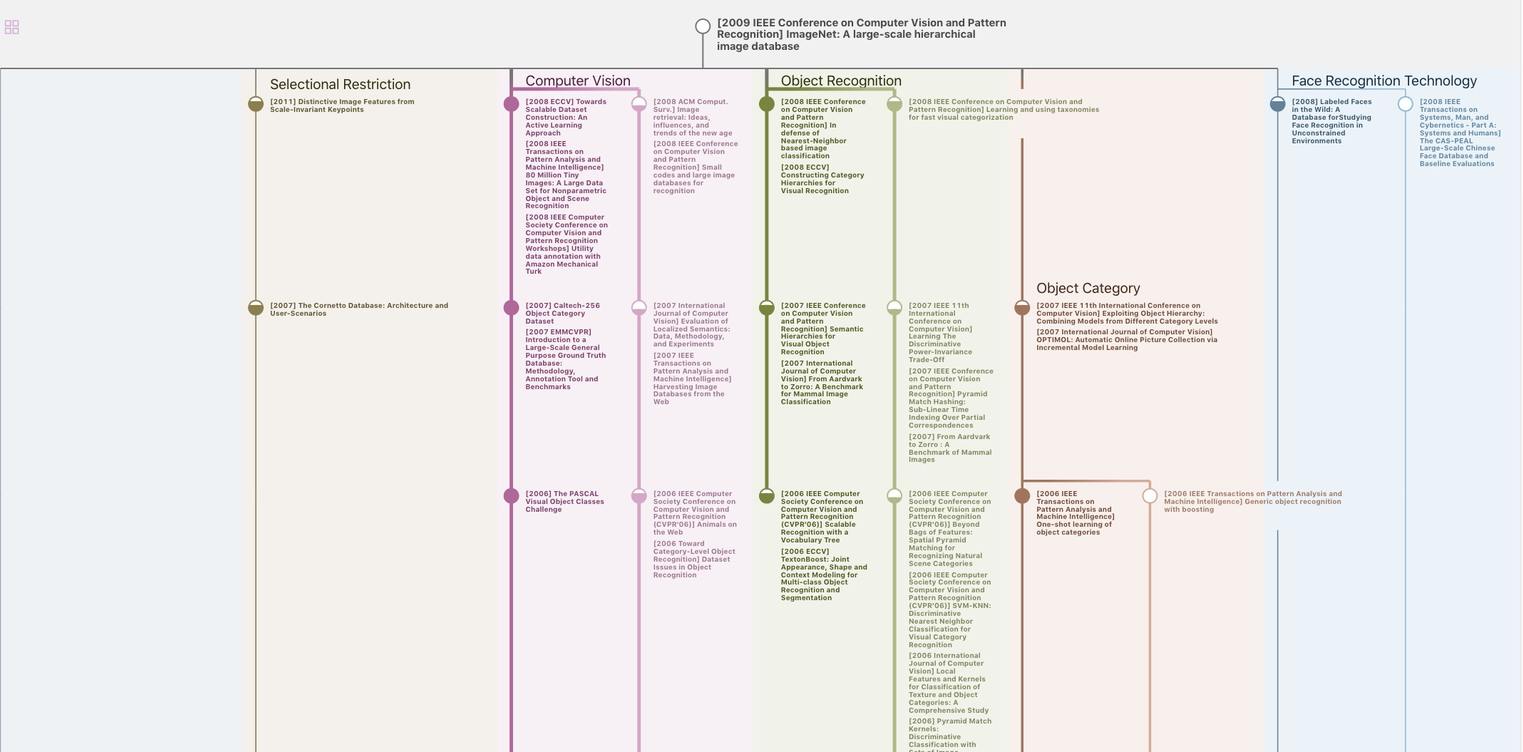
生成溯源树,研究论文发展脉络
Chat Paper
正在生成论文摘要