Proxy modelling for multi-well simulations: enabling identification of major input variables and reduced computation time over Monte Carlo sampling
The APPEA Journal(2019)
摘要
The petroleum industry uses high level dynamic simulations applied to geocellular models to guide forecasts of oil, gas and water production. Uncertainty in model choice and input variable selection is often addressed through large numbers of computationally slow Monte Carlo simulations designed around physics based models. Here, an alternate approach is proposed, which uses a relatively small amount of data and a reduced number of simulations of the high level physics model to train a fast (to evaluate) proxy or surrogate model based on a Polynomial Chaos Expansion. We give details of the theory and incorporated techniques, which significantly increase flexibility. Input variables (e.g. cell-by-cell variations in porosity and permeability) are sampled from unknown probability distributions and sensitivity analysis is based on low level proxy models. The theory is tested by developing proxy models to predict total gas production from a five-spot well configuration in the Hermitage area that taps into the Walloon Coal Measures of the Surat Basin in Queensland. Synthetic training data is simulated using commercial dynamic simulation software based on a high level physics model.
更多查看译文
关键词
simulations,monte carlo,major input variables,multi-well
AI 理解论文
溯源树
样例
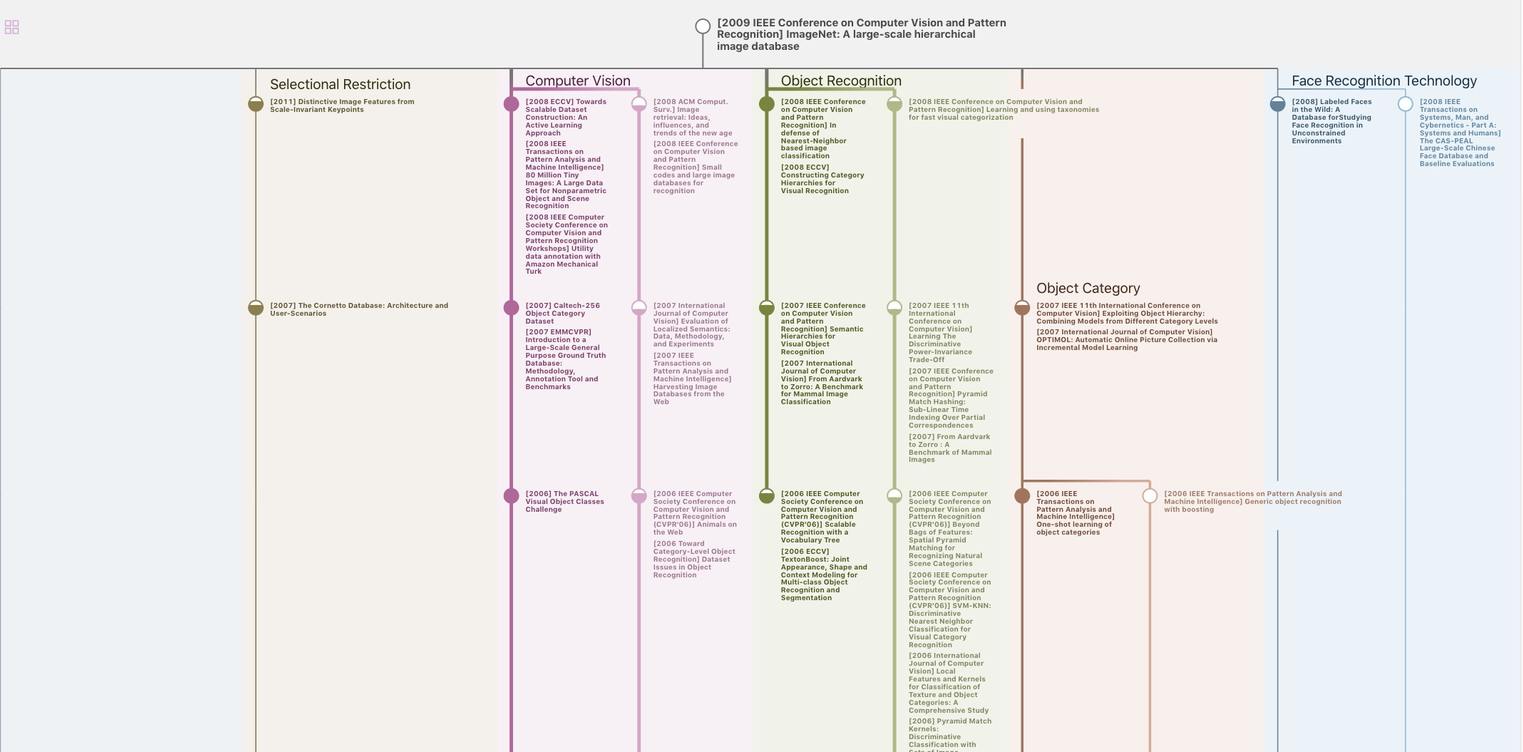
生成溯源树,研究论文发展脉络
Chat Paper
正在生成论文摘要