Rule Set Complexity in Mining Incomplete Data Using Global and Saturated Probabilistic Approximations.
Communications in Computer and Information Science(2019)
摘要
We discuss incomplete data sets with two interpretations of missing attribute values, lost values and "do not care" conditions. For data mining we use two probabilistic approximations, global and saturated. In our previous research, we compared four approaches, combining two interpretations of missing attribute values with two types of probabilistic approximations, using the error rate evaluated by ten-fold cross validation as the criterion of quality. Results of this research showed that though there are significant differences (with a 5% level of significance), however no approach is a winner. So in this paper the main objective is to compare complexity of rule sets induced by our four approaches. We show that there are significant differences between the four approaches; however, the difference between used probabilistic approximations is not significant. The only significant difference is between the two interpretations of missing attribute values. Our main result is that using the "do not care" condition interpretation of missing attribute values significantly reduces the complexity of induced rule sets.
更多查看译文
关键词
Incomplete data mining,Characteristic sets,Rough set theory,Probabilistic approximations
AI 理解论文
溯源树
样例
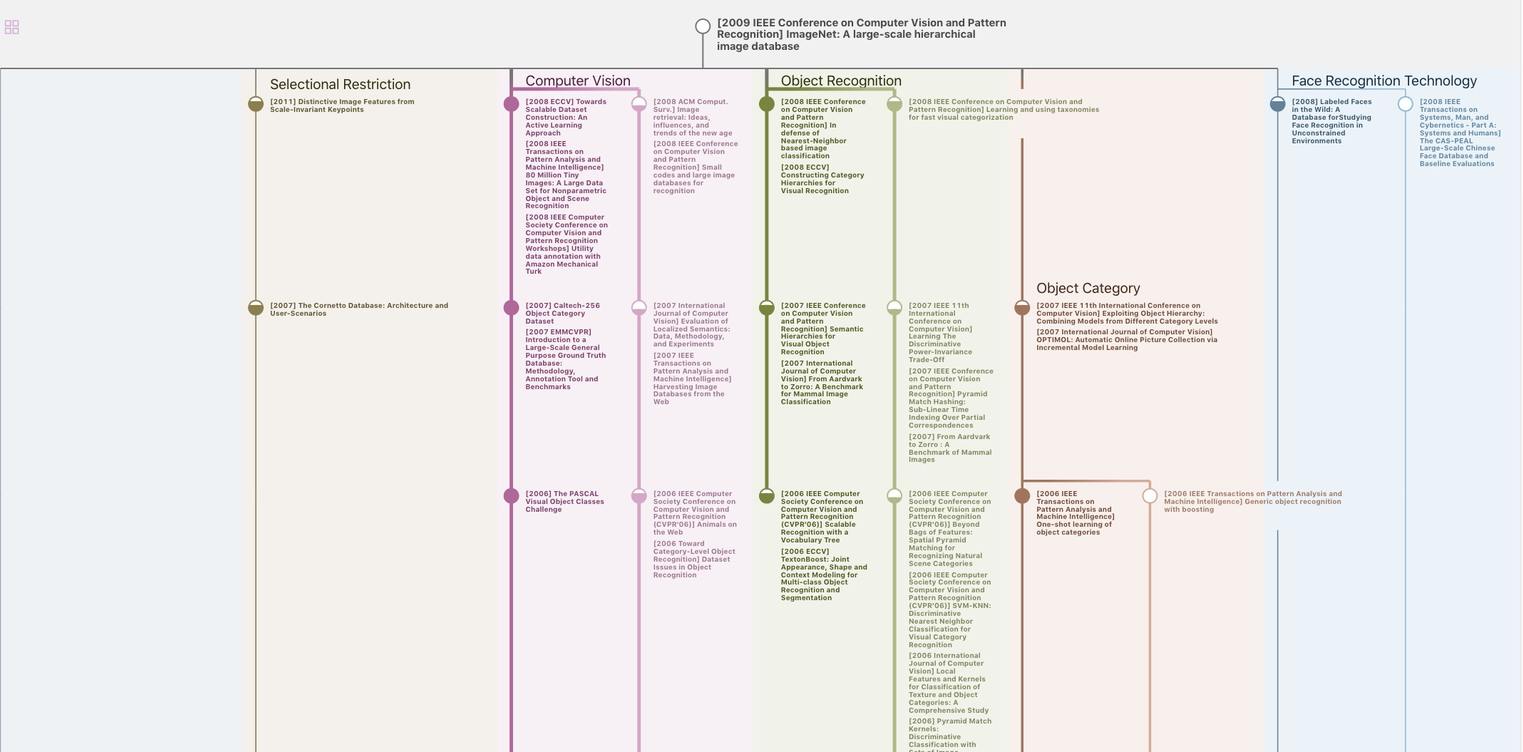
生成溯源树,研究论文发展脉络
Chat Paper
正在生成论文摘要