Hierarchical feature representation for unconstrained video analysis.
Neurocomputing(2019)
摘要
Complex video analysis is a challenging problem due to the long and sophisticated temporal structure of unconstrained videos. This paper introduces pooled-feature representation (PFR) which is derived from a double layer encoding framework (DLE) to address this problem. Considering that a complex video is composed of a sequence of simple frames, the first layer generates temporal sub-volumes from the video and represents them individually. The second layer constructs the pool of features by fusing the represented vectors from the first layer. The pool is compressed and then encoded to provide video-parts vector (VPV). This framework allows distilling the representation and extracting new information in a hierarchical way. Compared with recent video encoding approaches, VPV can preserve the higher-level information through typical encoding in the higher layer. Furthermore, the encoded vectors from both layers of DLE are fused along with a compression stage to develop PFR. The early and late fusion stages are adopted based on the priority of compression stage over concatenation of represented vectors. To validate the proposed framework, we conduct extensive experiments on four complex action datasets: UCF50, HMDB51, URADL, and Olympic. Experimental results demonstrate that PFR with early fusion achieves the state-of-the-art performance by capturing the most prominent features with minimum dimension.
更多查看译文
关键词
Video analysis,Visual learning,Data compression
AI 理解论文
溯源树
样例
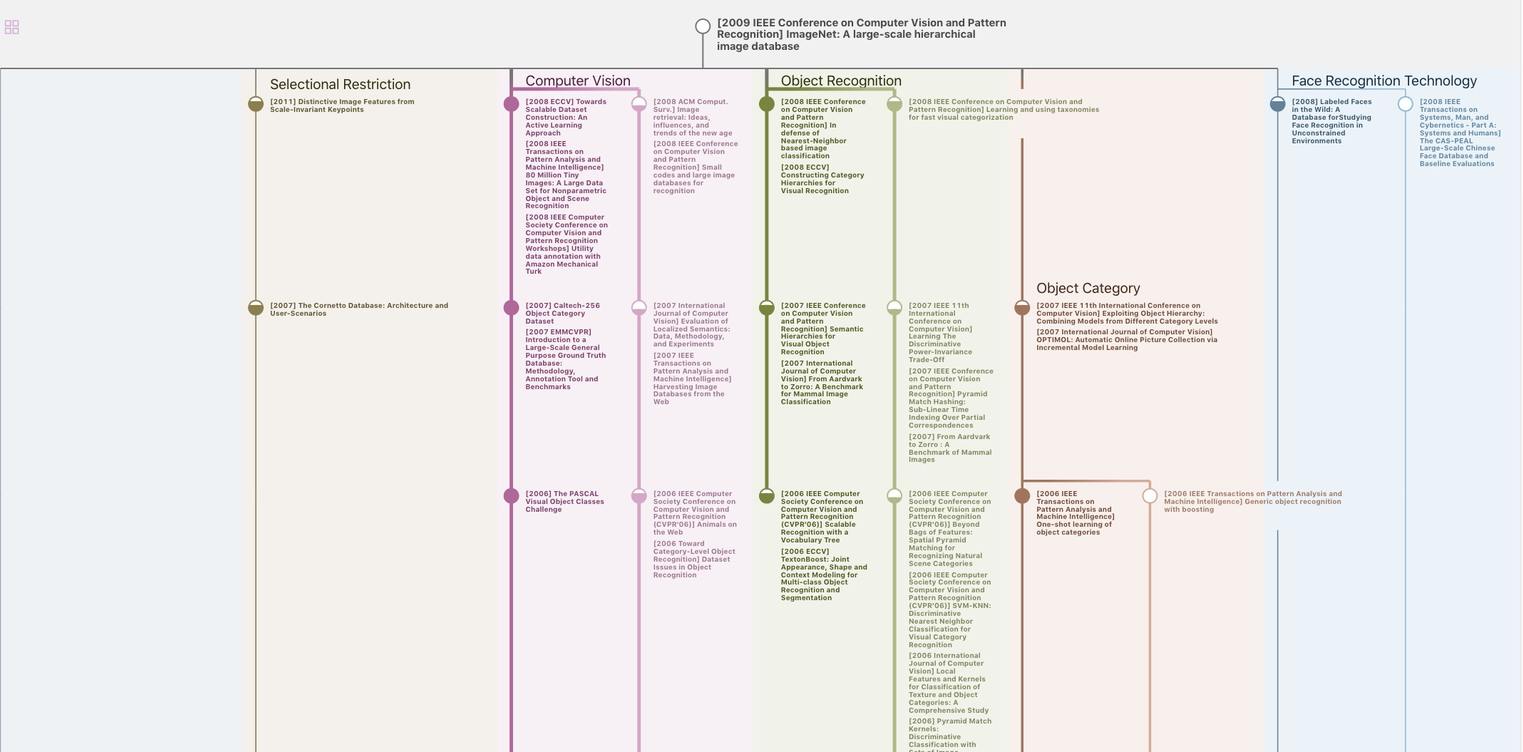
生成溯源树,研究论文发展脉络
Chat Paper
正在生成论文摘要