Self-Organizing Cellular Radio Access Network with Deep Learning
IEEE INFOCOM 2019 - IEEE Conference on Computer Communications Workshops (INFOCOM WKSHPS)(2019)
摘要
Network operators require a high level of performance and reliability for the cellular radio access network (RAN) to deliver high quality of service for mobile users. However, these network do experience some rare and hard-to-predict anomaly events, for example, hardware failures and high radio interference, which can significantly degrade performance and end-user experience. In this work, we propose SORA, a self-organizing cellular radio access network system enhanced with deep learning. SORA involves four core components: self-KPIs monitoring, self-anomaly prediction, self-root cause analysis, and self-healing. In particular, we design and implement the anomaly prediction and root cause analysis components with deep learning methods and evaluate the system performance with 6 months of real-world data from a top-tier US cellular network operator. We demonstrate that the proposed methods can achieve 86.9% accuracy in predicting anomalies and 99.5% accuracy for root cause analysis of network faults.
更多查看译文
关键词
self-organizing cellular radio access network,network operators,mobile users,anomaly events,hardware failures,high radio interference,end-user experience,SORA,cellular radio access network system,self-anomaly prediction,self-root cause analysis,deep learning methods,system performance,network faults,top-tier US cellular network operator
AI 理解论文
溯源树
样例
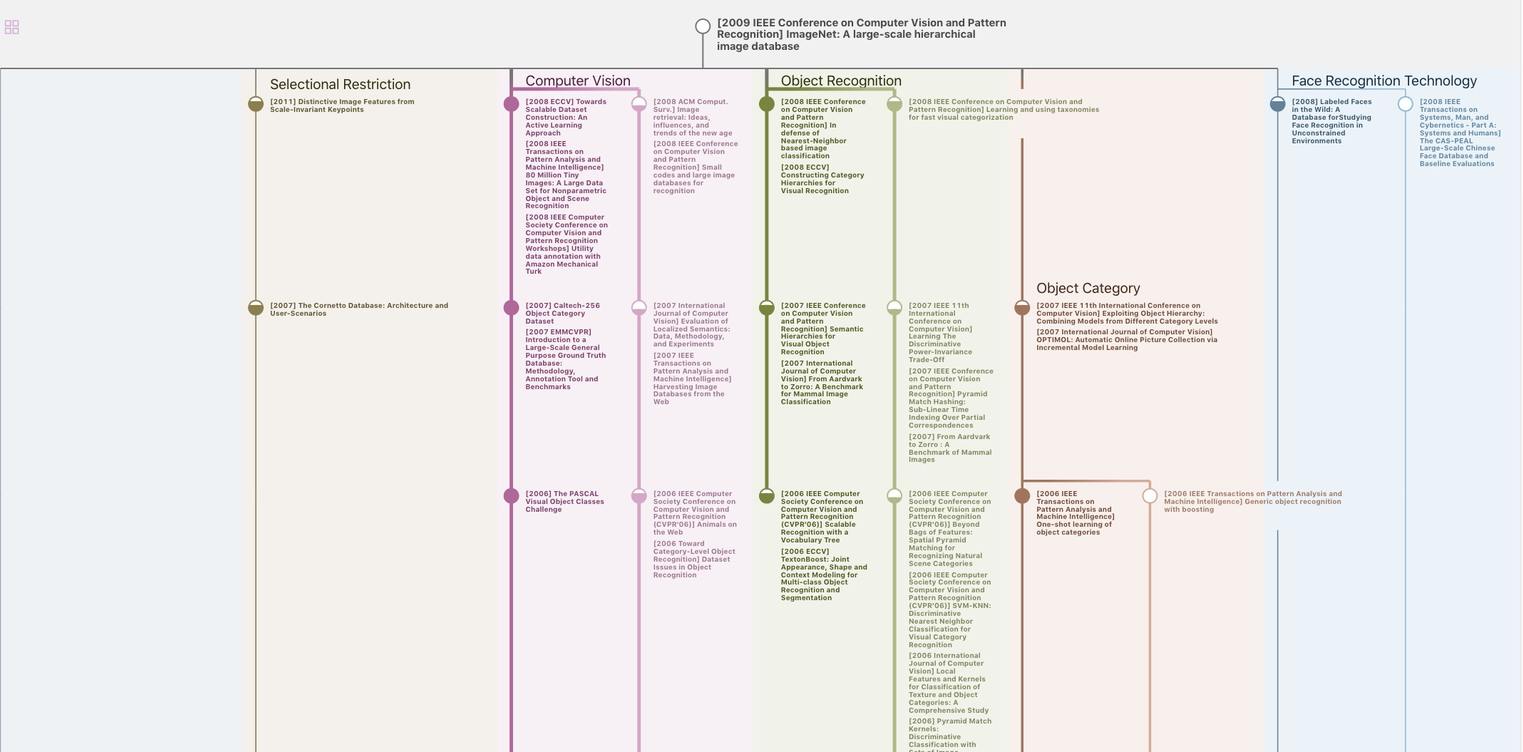
生成溯源树,研究论文发展脉络
Chat Paper
正在生成论文摘要