EdgeNet: Balancing Accuracy and Performance for Edge-based Convolutional Neural Network Object Detectors
Proceedings of the 13th International Conference on Distributed Smart Cameras(2019)
摘要
Visual intelligence at the edge is becoming a growing necessity for low latency applications and situations where real-time decision is vital. Object detection, the first step in visual data analytics, has enjoyed significant improvements in terms of state-of-the-art accuracy due to the emergence of Convolutional Neural Networks (CNNs) and Deep Learning. However, such complex paradigms intrude increasing computational demands and hence prevent their deployment on resource-constrained devices. In this work, we propose a hierarchical framework that enables to detect objects in high-resolution video frames, and maintain the accuracy of state-of-the-art CNN-based object detectors while outperforming existing works in terms of processing speed when targeting a low-power embedded processor using an intelligent data reduction mechanism. Moreover, a use-case for pedestrian detection from Unmanned-Areal-Vehicle (UAV) is presented showing the impact that the proposed approach has on sensitivity, average processing time and power consumption when is implemented on different platforms. Using the proposed selection process our framework manages to reduce the processed data by 100x leading to under 4W power consumption on different edge devices.
更多查看译文
关键词
Aerial Cameras, Convolutional Neural Networks, Object Detection, Pedestrian Detection
AI 理解论文
溯源树
样例
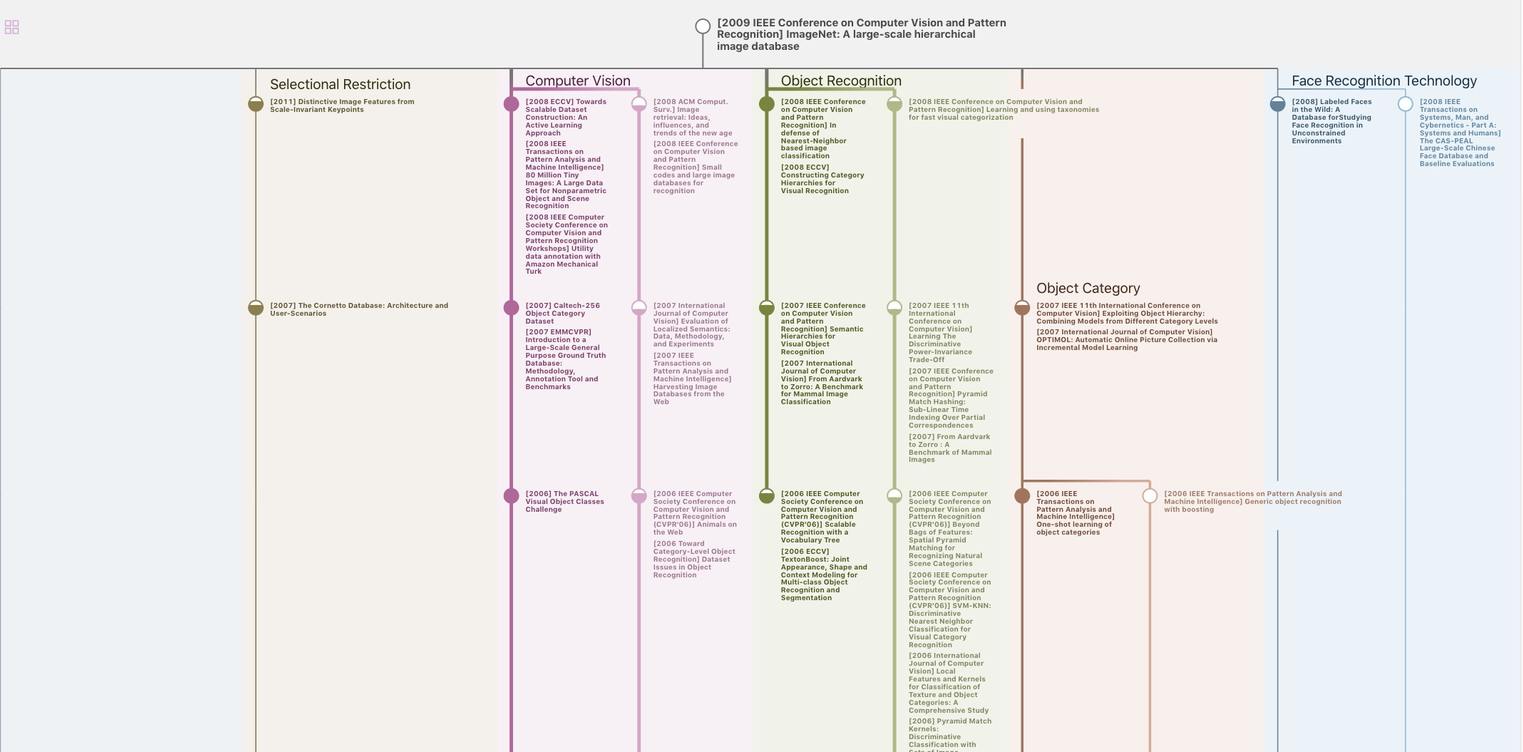
生成溯源树,研究论文发展脉络
Chat Paper
正在生成论文摘要