Impact of Non-Ideal Characteristics of Resistive Synaptic Devices on Implementing Convolutional Neural Networks
IEEE Journal on Emerging and Selected Topics in Circuits and Systems(2019)
摘要
Emerging non-volatile memory (eNVM) based resistive synaptic devices have shown great potential for implementing deep neural networks (DNNs). However, the eNVM devices typically suffer from various non-ideal effects which may degrade the performance of the system. Based on a representative convolutional neural network (CNN) model for CIFAR-10 dataset, this paper comprehensively investigates the impact of those non-ideal characteristics, such as nonlinearity and asymmetry of conductance tuning, variations, endurance and retention, on the training/inference accuracy. The compact models of the device non-ideal effects are incorporated into the TensorFlow framework. Our simulation results suggest that 1) the training accuracy is more sensitive to the asymmetry of conductance tuning than the nonlinearity; 2) the conductance range variation does not degrade the training accuracy, instead, a small variation can even reduce the accuracy loss introduced by asymmetry; 3) device-to-device variation can also remedy the accuracy loss due to asymmetry while cycle-to-cycle variation leads to significant accuracy degradation; 4) the accuracy degradation will not be noticeable if the endurance cycles are more than 7,000 cycles; and 5) different drifting modes affect the inference accuracy differently, and the best case is where the conductance is drifting up/down randomly.
更多查看译文
关键词
Training,Tuning,Random access memory,Degradation,Convolutional neural networks,System-on-chip
AI 理解论文
溯源树
样例
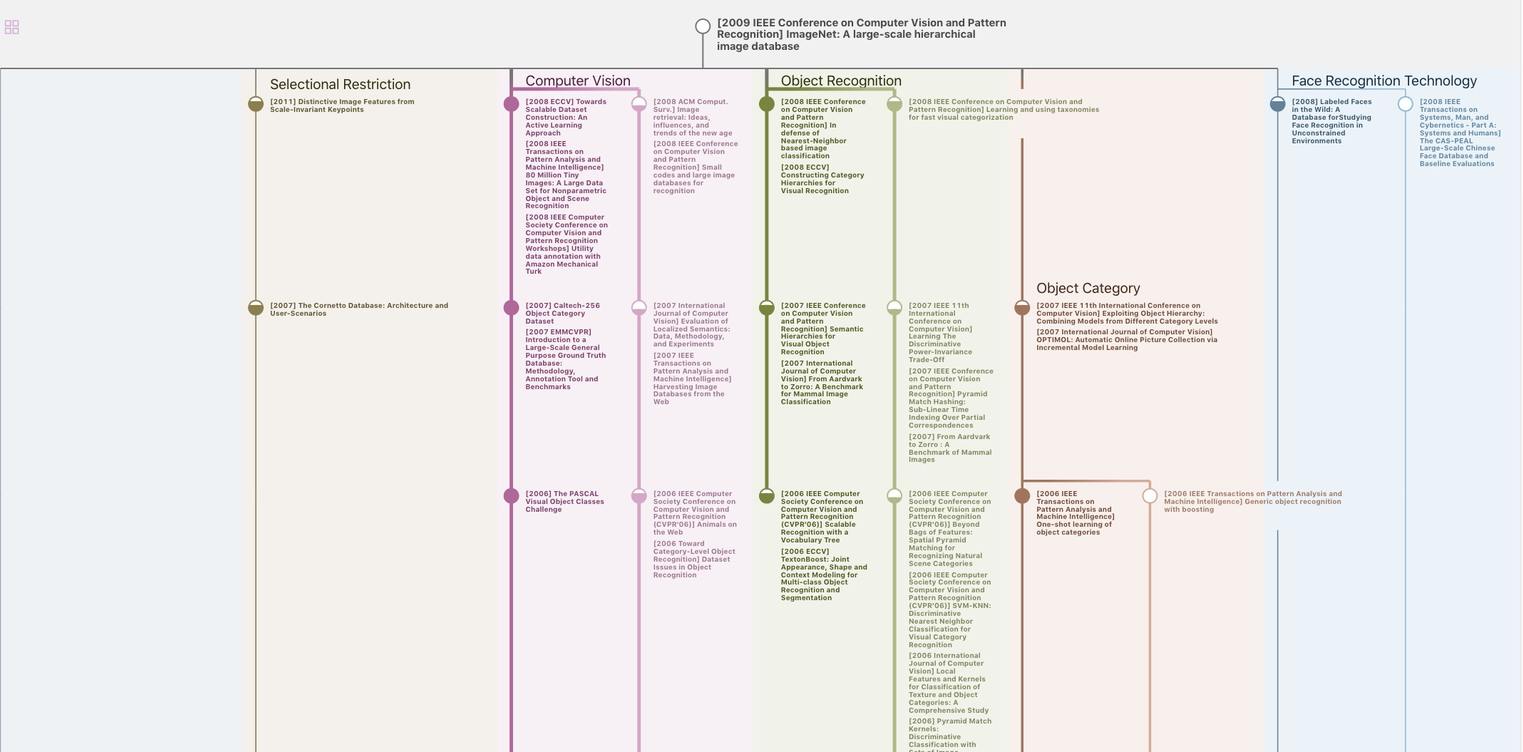
生成溯源树,研究论文发展脉络
Chat Paper
正在生成论文摘要