Learning to Find Hydrological Corrections.
27TH ACM SIGSPATIAL INTERNATIONAL CONFERENCE ON ADVANCES IN GEOGRAPHIC INFORMATION SYSTEMS (ACM SIGSPATIAL GIS 2019)(2019)
摘要
High resolution Digital Elevation models, such as the grid terrain model of Denmark with more than 200 billion measurements, is a basic requirement for water flow modelling and flood risk analysis. However, a large number of modifications often need to be made to even very accurate terrain models, before they can be used in realistic flow modeling. This include removal of bridges, which otherwise act as dams in flow modeling, and inclusion of culverts that transport water underneath roads. For this reason, there is list of known hydrological corrections for the danish model. However, producing this list is a slow an expensive process, since it is to a large extent done manually, often with only local input. In this paper we propose a new algorithmic approach based on machine learning and convolutional neural networks for automatically detecting hydrological corrections on large terrain data. Our model is able to detect most known hydrological corrections and quite a few more that should have been included in the original list.
更多查看译文
关键词
geographic information systems, flow modelling, hydrological conditioning, neural networks
AI 理解论文
溯源树
样例
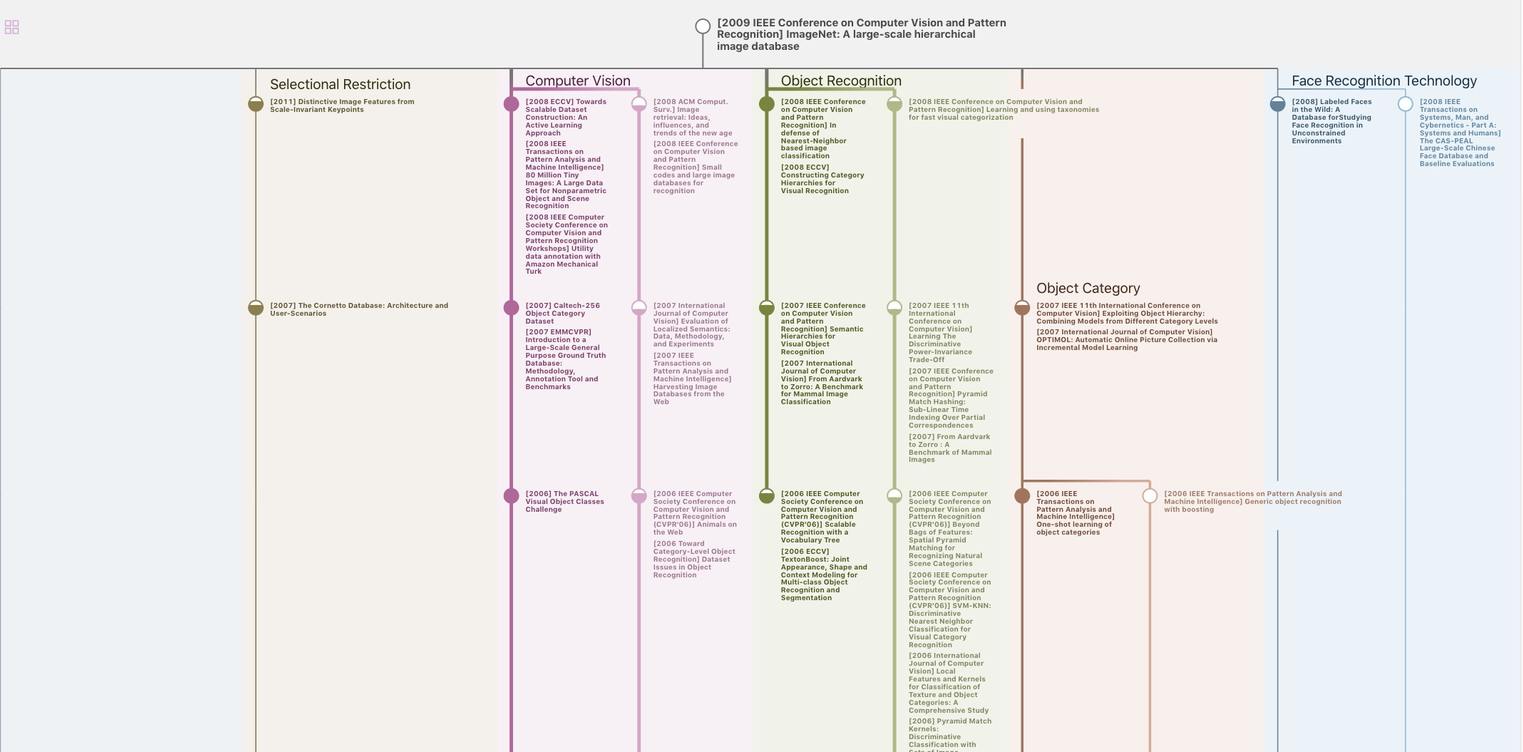
生成溯源树,研究论文发展脉络
Chat Paper
正在生成论文摘要