A Self-Attentional Neural Architecture for Code Completion with Multi-Task Learning
International Conference on Software Engineering(2020)
摘要
ABSTRACTCode completion, one of the most useful features in the Integrated Development Environments (IDEs), can accelerate software development by suggesting the libraries, APIs, and method names in real-time. Recent studies have shown that statistical language models can improve the performance of code completion tools through learning from large-scale software repositories. However, these models suffer from three major drawbacks: a) The hierarchical structural information of the programs is not fully utilized in the program's representation; b) In programs, the semantic relationships can be very long. Existing recurrent neural networks based language models are not sufficient to model the long-term dependency. c) Existing approaches perform a specific task in one model, which leads to the underuse of the information from related tasks. To address these challenges, in this paper, we propose a self-attentional neural architecture for code completion with multi-task learning. To utilize the hierarchical structural information of the programs, we present a novel method that considers the path from the predicting node to the root node. To capture the long-term dependency in the input programs, we adopt a self-attentional architecture based network as the base language model. To enable the knowledge sharing between related tasks, we creatively propose a Multi-Task Learning (MTL) framework to learn two related tasks in code completion jointly. Experiments on three real-world datasets demonstrate the effectiveness of our model when compared with state-of-the-art methods.
更多查看译文
关键词
Code completion,Hierarchical structure,Multi-task learning,Self-attention
AI 理解论文
溯源树
样例
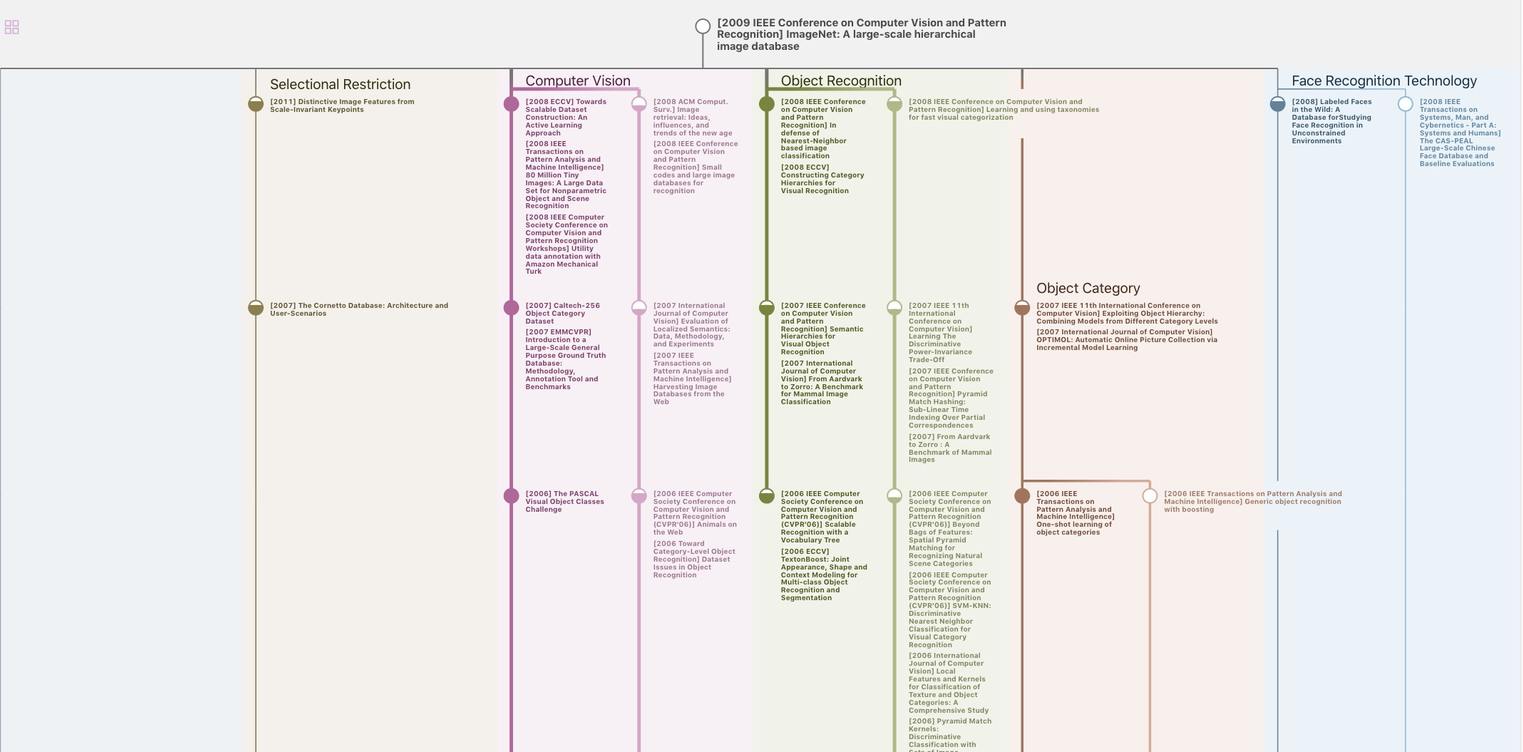
生成溯源树,研究论文发展脉络
Chat Paper
正在生成论文摘要