A spatio-temporal model for EEG-based person identification
Multimedia Tools and Applications(2019)
摘要
Biometric representation of humans often requires the tasks such as identification and verification. This can be done using various modalities such as fingerprint, face, retina, voice, etc. However, existing biometric systems are vulnerable to various security attacks. Recently, Electroencephalography (EEG) is considered to be one of the alternatives to develop a robust biometric system. Brain activities represented by EEG signals are more sensitive, secure, and difficult to copy and steal. In this paper, we propose a spatio-temporal dense architecture for EEG-based person identification. Firstly, raw EEG are processed to extract robust and informative spatial features using Convolutional Neural Networks (CNN) as they are known for automatic feature extraction from the raw data. Then, a Long short-term memory (LSTM) network is utilized to process temporal data and person identification is carried out. The experiment has been carried out on a publicly available dataset consisting of EEG of 109 subjects. The architecture is tested on two baseline situations, i.e., eye closed (EC) and the eye opened (EO). Person identification rates of 99.95% and 98% have been recorded for EC and EO states using the proposed scheme. Experimental results demonstrate the robustness of the proposed scheme in terms of person identification and outstrip existing works.
更多查看译文
关键词
Biometric, Electroencephalogram, CNN, LSTM
AI 理解论文
溯源树
样例
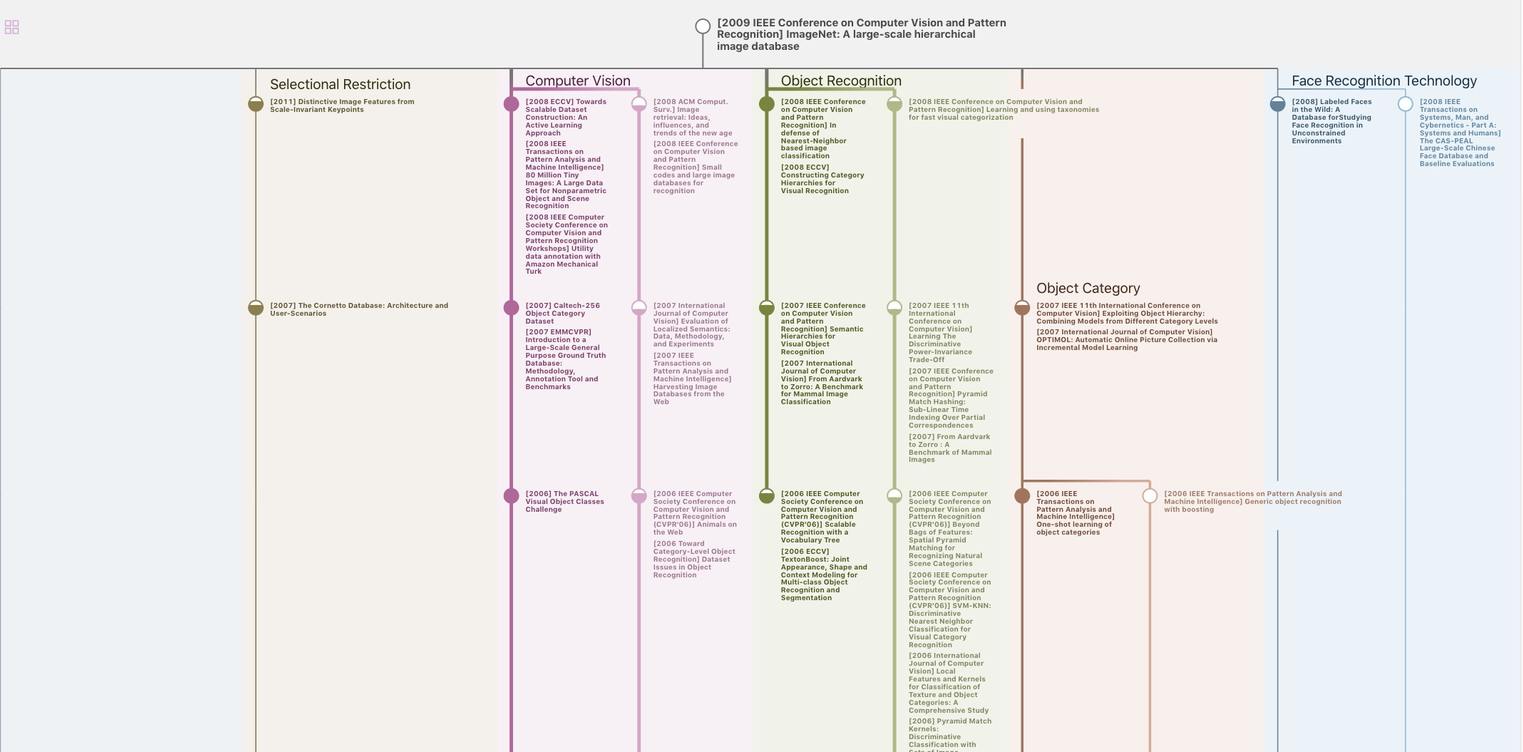
生成溯源树,研究论文发展脉络
Chat Paper
正在生成论文摘要