Latent Question Interpretation Through Variational Adaptation.
IEEE/ACM Trans. Audio, Speech & Language Processing(2019)
Abstract
Most artificial neural network models for question-answering rely on complex attention mechanisms. These techniques demonstrate high performance on existing datasets; however, they are limited in their ability to capture natural language variability, and to generate diverse relevant answers. To address this limitation, we propose a model that learns multiple interpretations of a given question. This diversity is ensured by our interpretation policy module which automatically adapts the parameters of a question-answering model with respect to a discrete latent variable. This variable follows the distribution of interpretations learned by the interpretation policy through a semi-supervised variational inference framework. To boost the performance further, the resulting policy is fine-tuned using the rewards from the answer accuracy with a policy gradient. We demonstrate the relevance and efficiency of our model through a large panel of experiments. Qualitative results, in particular, underline the ability of the proposed architecture to discover multiple interpretations of a question. When tested using the Stanford Question Answering Dataset 1.1, our model outperforms the baseline methods in finding multiple and diverse answers. To assess our strategy from a human standpoint, we also conduct a large-scale user study. This study highlights the ability of our network to produce diverse and coherent answers compared to existing approaches. Our Pytorch implementation is available as open source.11github.com/parshakova/APIP.
MoreTranslated text
Key words
Adaptation models,Training,Speech processing,Feature extraction,Neural networks,Knowledge discovery,Indexes
AI Read Science
Must-Reading Tree
Example
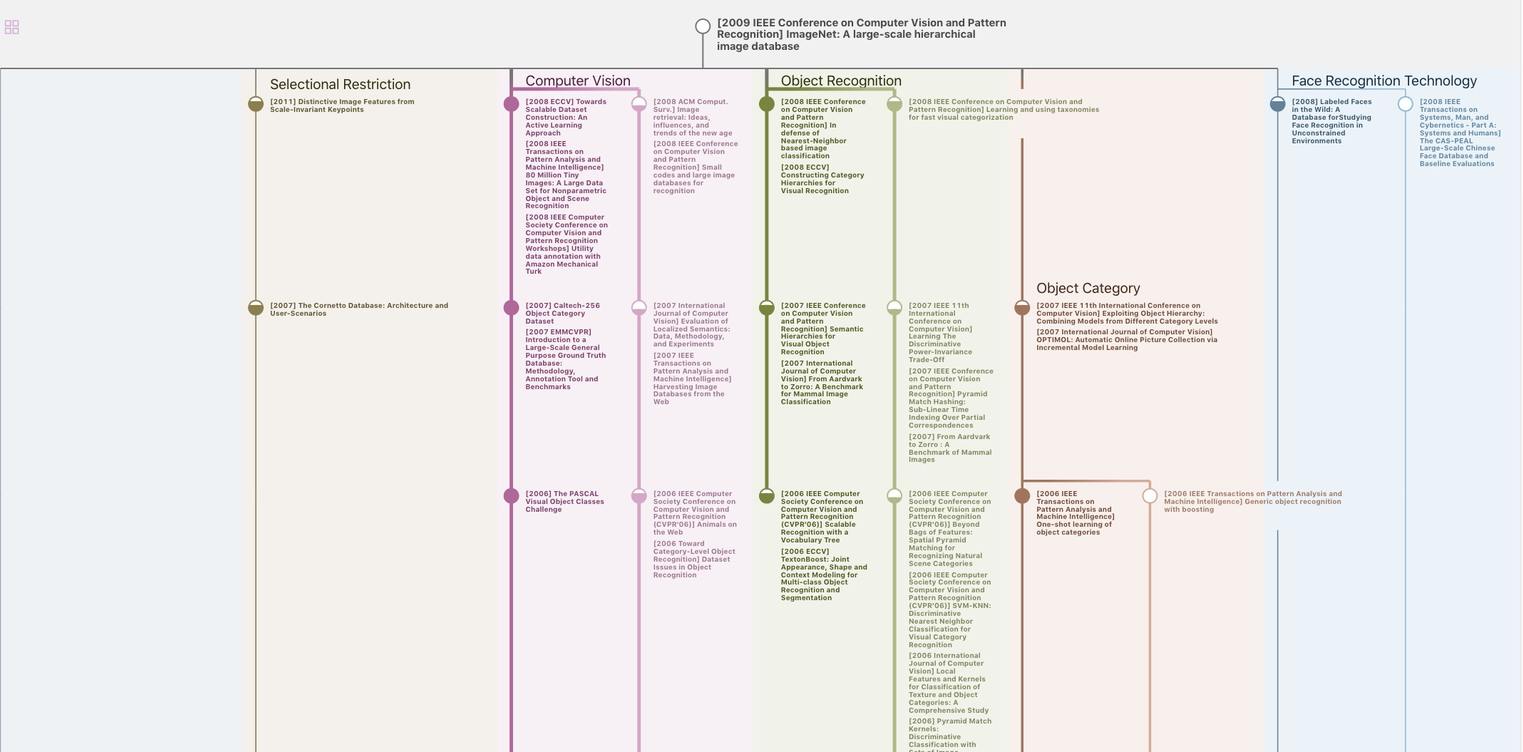
Generate MRT to find the research sequence of this paper
Chat Paper
Summary is being generated by the instructions you defined