Rotation-equivariant convolutional neural network ensembles in image processing
Proceedings of the 2019 ACM International Joint Conference on Pervasive and Ubiquitous Computing and Proceedings of the 2019 ACM International Symposium on Wearable Computers(2019)
摘要
For the present engineering of neural networks, rotation invariant is hard to be obtained. Rotation symmetry is an important characteristic in our physical world. In image recognition, using rotated images would largely decrease the performance of neural networks. This situation seriously hindered the application of neural networks in the real-world, such as human tracking, self-driving cars, and intelligent surveillance. In this paper, we would like to present a rotation-equivariant design of convolutional neural network ensembles to counteract the problem of rotated image processing task. This convolutional neural network ensembles combine multiple convolutional neural networks trained by different ranges of rotation angles respectively. In our proposed theory, the model lowers the training difficulty by learning with smaller separations of random rotation angles instead of a huge one. Experiments are reported in this paper. The convolutional neural network ensembles could reach 96.35% on rotated MNIST datasets, 84.9% on rotated Fashion-MNIST datasets, and 91.35% on rotated KMNIST datasets. These results are comparable to current state-of-the-art performance.
更多查看译文
关键词
ensembling learning, neural networks, rotation equivariant
AI 理解论文
溯源树
样例
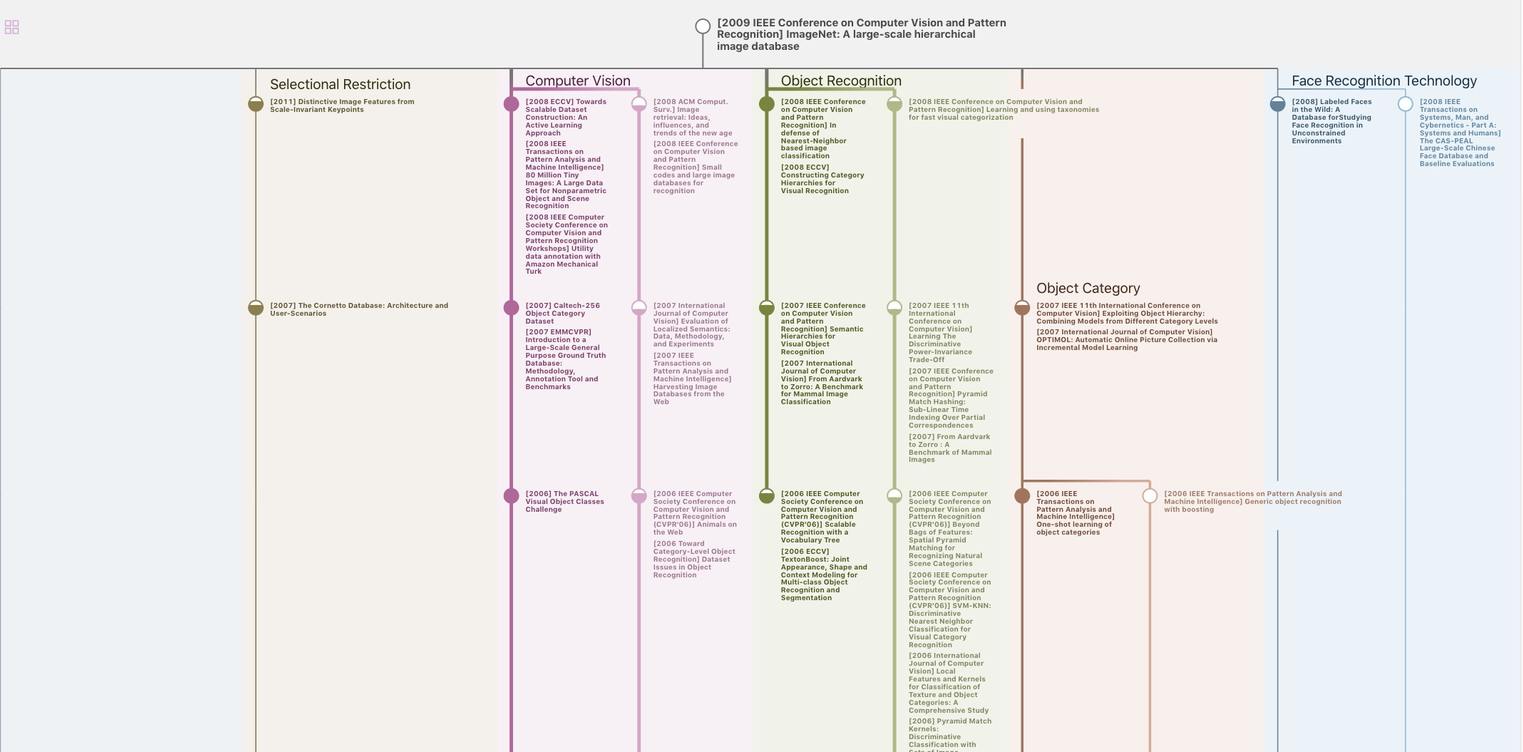
生成溯源树,研究论文发展脉络
Chat Paper
正在生成论文摘要