A Predictive Model for MicroRNA Expressions in Pediatric Multiple Sclerosis Detection.
MDAI(2019)
摘要
MicroRNAs (miRNAs) are a set of short non coding RNAs that play significant regulatory roles in cells. The study of miRNA data can be of valuable support for the early diagnosis of multifactorial diseases such as pediatric Multiple Sclerosis. However the analysis of miRNA expressions poses several challenges due to high dimensionality and imbalance of data. In this paper we present a data science workflow to develop a predictive model that is intended to support the clinicians in the diagnosis of Multiple Sclerosis starting from miRNA data produced by Next-Generation Sequencing. The goal is to create an effective model able to predict the pathological condition of a patient starting from his miRNA expression profile. Based on the proposed workflow, the miRNA dataset is firstly preprocessed in order to reduce its high dimensionality (from 1287 features to 40 features) and to mitigate class imbalance. Then a classification model is learnt from data via neural network training. Results show that the model defined by using the 40 data-driven selected features achieves an overall classification accuracy of 94% on test data and overcomes the model based on 42 features selected by the experts that achieves only 83% of overall accuracy.
更多查看译文
关键词
microRNA expression, Next-Generation Sequencing, Pediatric Multiple Sclerosis, Feature selection, Artificial neural networks, Classification.
AI 理解论文
溯源树
样例
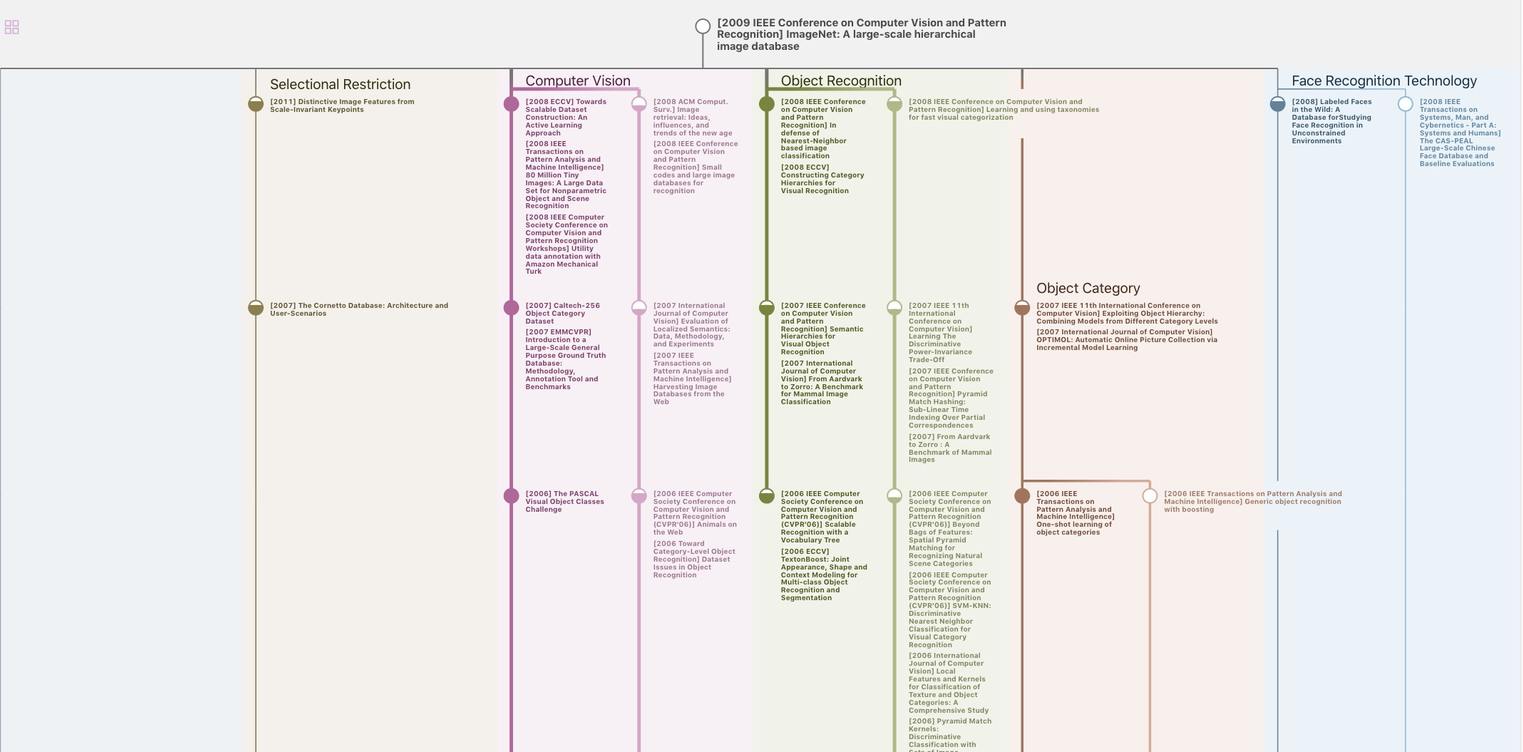
生成溯源树,研究论文发展脉络
Chat Paper
正在生成论文摘要