Warped Gaussian Processes and Derivative-Based Sequential Designs for Functions with Heterogeneous Variations.
SIAM-ASA JOURNAL ON UNCERTAINTY QUANTIFICATION(2018)
摘要
Gaussian process (GP) models have become popular for approximating and exploring nonlinear systems using scarce input/output samples and prior hypotheses done through mean and covariance functions. While it is common to make stationarity assumptions and use variance-based criteria for exploration, in realistic cases it is not rare that systems under study exhibit a heterogeneous behavior depending on regions of the parameter space. We consider a class of problems where high variations occur along unknown noncanonical directions and we tackle the problem of accommodating nonstationarity from two angles. First we define a novel class of covariances (WaMI-GP) that simultaneously generalizes kernels of multiple index and of tensorized warped GPs, and second, we introduce derivative-based sampling criteria dedicated to the exploration of high-variation regions. The novel GP class is investigated through both mathematical analysis and numerical experiments, and it is shown that it allows encoding much expressiveness while keeping the number of parameters to be inferred moderate. Criteria and models are compared on a mechanical test case from safety studies conducted by IRSN. On this application some of the proposed criteria outperform usual variance-based criteria in the case of a stationary GP model; however, variance-based criteria with WaMI-GP perform even better. Our method is also compared with the treed Gaussian processes (TGP) on this application and on a NASA test case. In the IRSN application, WaMI-GP dominates TGP in static and sequential settings. In the NASA application, while TGP clearly dominates in the static case, for small designs it is outperformed by WaMI-GP in the sequential setup.
更多查看译文
关键词
nonstationary kernels,infill sampling criteria,computer experiments
AI 理解论文
溯源树
样例
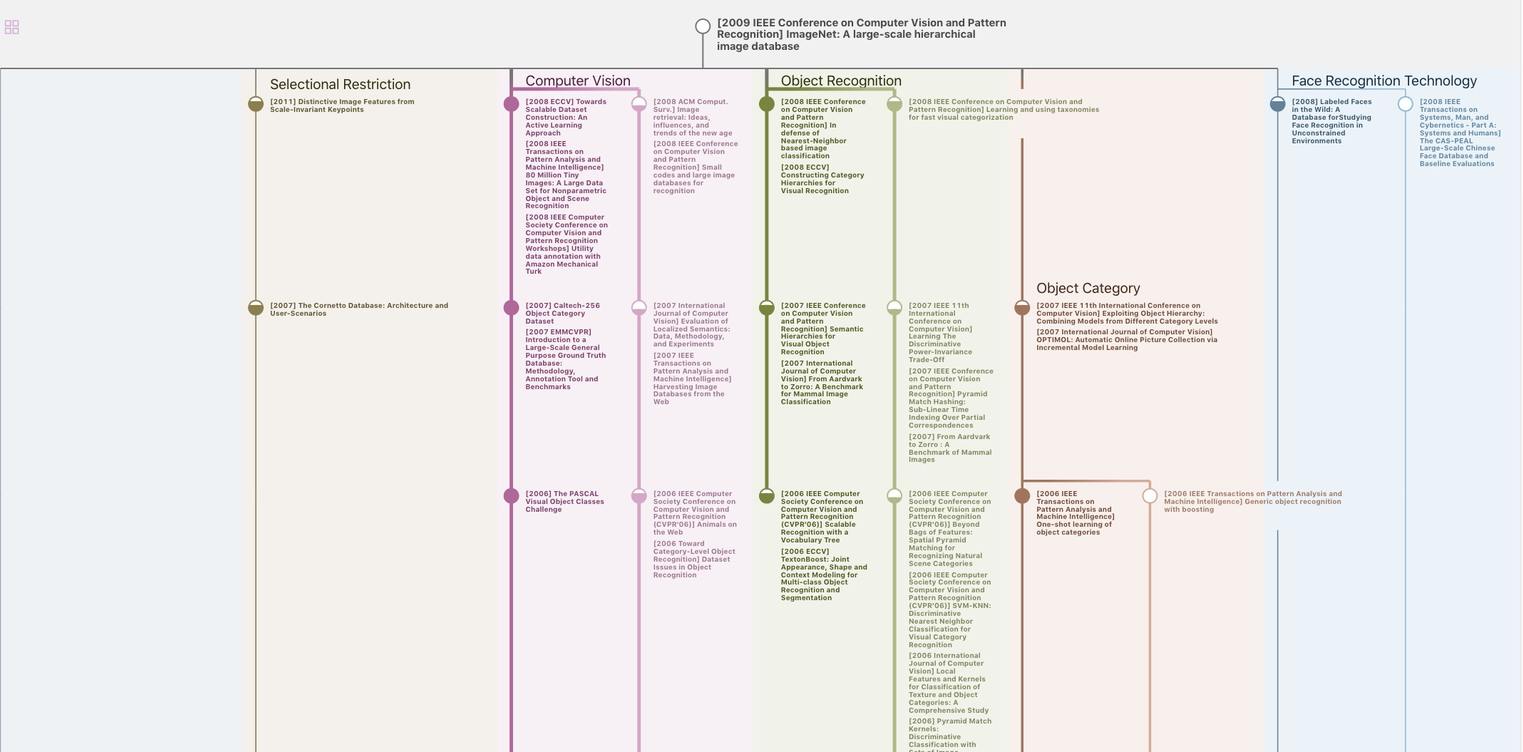
生成溯源树,研究论文发展脉络
Chat Paper
正在生成论文摘要