PerRNN: Personalized Recurrent Neural Networks for Acceleration-based Human Activity Recognition
IEEE International Conference on Communications(2019)
摘要
The ever-growing proliferation of mobile devices equipped with accelerometers has provided new opportunities to capture the semantic meanings of human activities and improve user experience with behavior-based recommendations, which heavily rely on the accuracy of the recognition of daily human activities. Acceleration-based human activity recognition (HAR) is a challenging problem because each accelerometer records multi-dimensional signals in both spatial and temporal domains that have different attributes for representing different activities or even the same activity. Thus we cannot directly compare these signals with each other, because they are embedded in a non-metric space. In this paper, we present a Personalized Recurrent Neural Network (PerRNN) to dynamically segment and recognize the human activities based on accelerometer data. Enlightened by the idea of spatiotemporal predictive learning, the proposed architecture is capable of memorizing different acceleration signals' appearances and temporal variations in a unified memory pool. We evaluate the performance of the proposed framework on a commonly used dataset, WISDM. Experiment results show that compared with state-of-the-art schemes, our proposed PerRNN system recognizes 6 different human activities with the highest overall accuracy of 96.44%.
更多查看译文
关键词
personalized recurrent neural networks,acceleration-based human activity recognition,behavior-based recommendations,daily human activities,accelerometer data,PerRNN,spatiotemporal predictive learning,acceleration signals
AI 理解论文
溯源树
样例
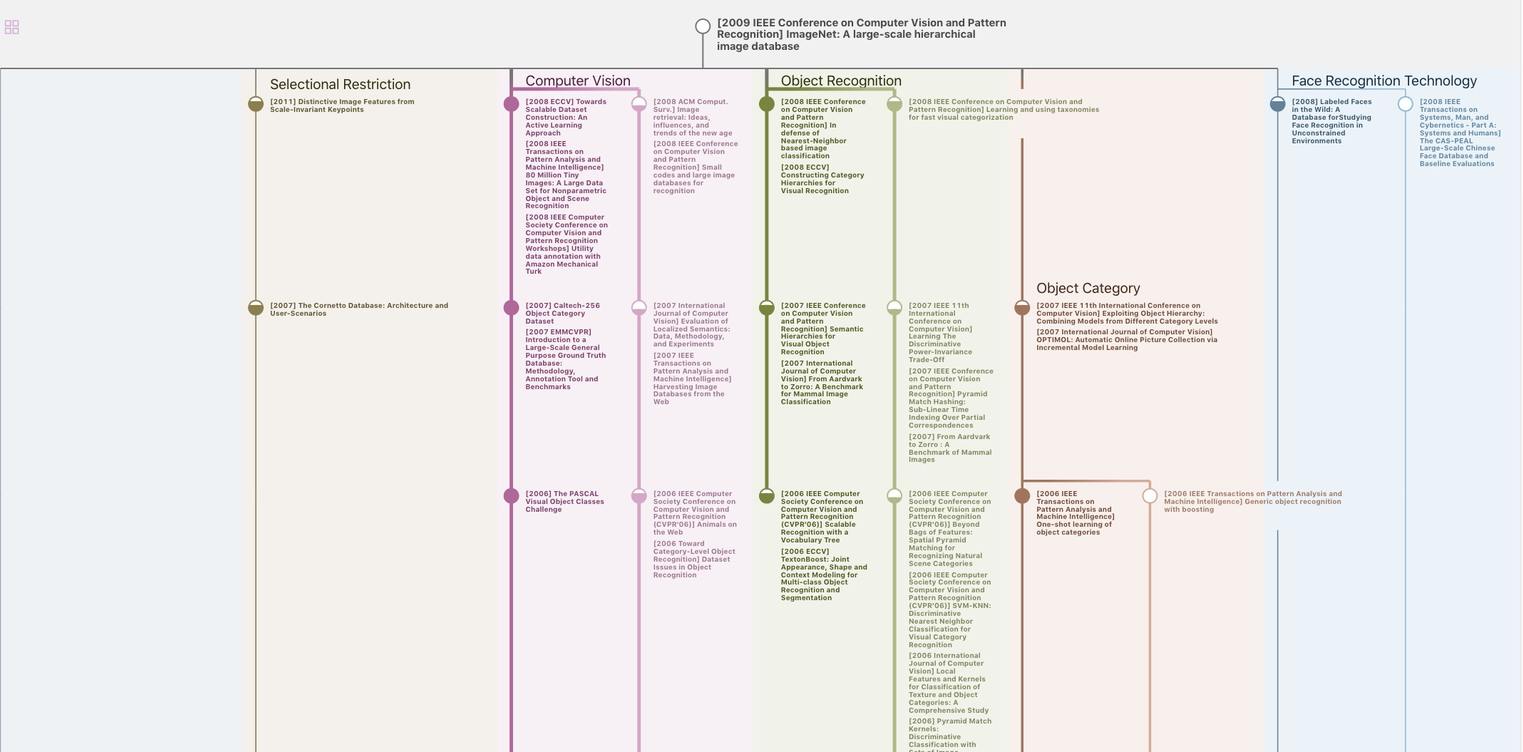
生成溯源树,研究论文发展脉络
Chat Paper
正在生成论文摘要