Semi-Supervised Methods for Explainable Legal Prediction
ICAIL '19: Proceedings of the Seventeenth International Conference on Artificial Intelligence and Law(2019)
摘要
Legal decision-support systems have the potential to improve access to justice, administrative efficiency, and judicial consistency, but broad adoption of such systems is contingent on development of technologies with low knowledge-engineering, validation, and maintenance costs. This paper describes two approaches to an important form of legal decision support---explainable outcome prediction---that obviate both annotation of an entire decision corpus and manual processing of new cases. The first approach, which uses an Attention Network for prediction and attention weights to highlight salient case text, was shown to be capable of predicting decisions, but attention-weight-based text highlighting did not demonstrably improve human decision speed or accuracy in an evaluation with 61 human subjects. The second approach, termed SCALE (Semi-supervised Case Annotation for Legal Explanations), exploits structural and semantic regularities in case corpora to identify textual patterns that have both predictable relationships to case decisions and explanatory value.
更多查看译文
关键词
Artificial Intelligence & Law, Computational Models of Argument, Human Language Technology, Legal Reasoning, Machine Learning
AI 理解论文
溯源树
样例
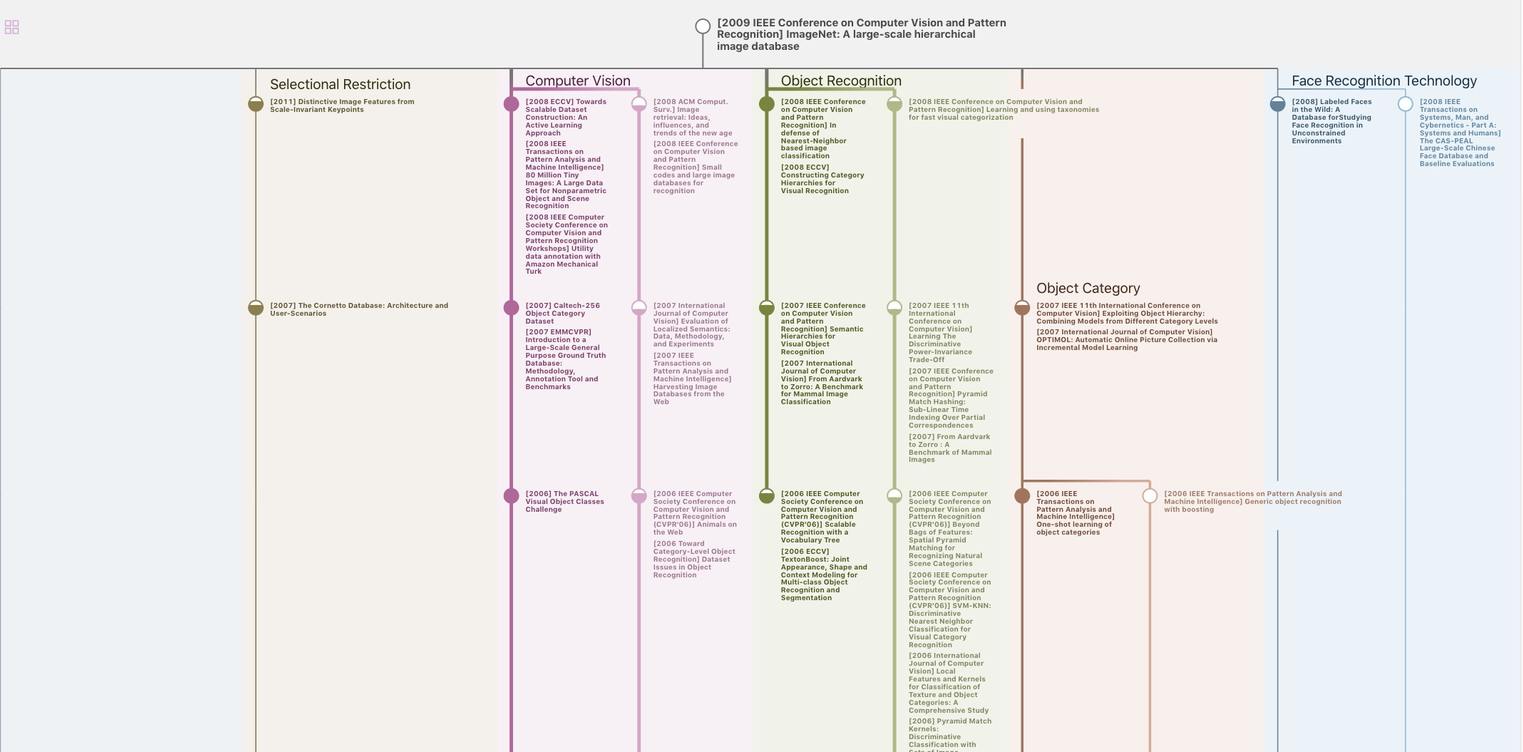
生成溯源树,研究论文发展脉络
Chat Paper
正在生成论文摘要