Rigorous Error Modeling for sUAS Acquired Image-Derived Point Clouds
ieee(2019)
摘要
The use of small unmanned aircraft systems to collect 3-D topographic data for a variety of geophysical applications has exploded in the last decade. These data are often produced from imagery using structure-from-motion (SfM) algorithms with or without ground control. While the accuracy of these data products has been assessed in a number of efforts, comparatively little focus has been placed on estimating spatially varying ground-space uncertainties in final data products using rigorous error propagation methodology and uncertainty estimation. Uncertainty estimates of the final 3-D products would provide users a more complete understanding of the quality of the products and therefore the applicability of the product. This paper illustrates an end-to-end workflow, using direct geopositioning and SfM techniques to create 3-D topographic point clouds with rigorously propagated accuracy estimates. Included is a method to quantify uncertainty in dense matching and incorporate those contributions into the final ground covariance. The uncertainty estimates are captured as metadata in the point cloud file using standard formats for exploitation. These estimates are critical to the downstream analysis, exploitation, and fusion of data sets; especially in scenarios where ground truth is not an option. The testing of this end-to-end process is described; data from a field campaign were processed without using mensurated control, and the resultant point clouds were compared to surveyed points to evaluate the predicted accuracy. Predicted accuracies bounded observed errors in the horizontal and vertical components. Future enhancements are also proposed.
更多查看译文
关键词
Dense matching,disparity,error propagation,photogrammetry,point cloud,unmanned aerial vehicle,unmanned aircraft system
AI 理解论文
溯源树
样例
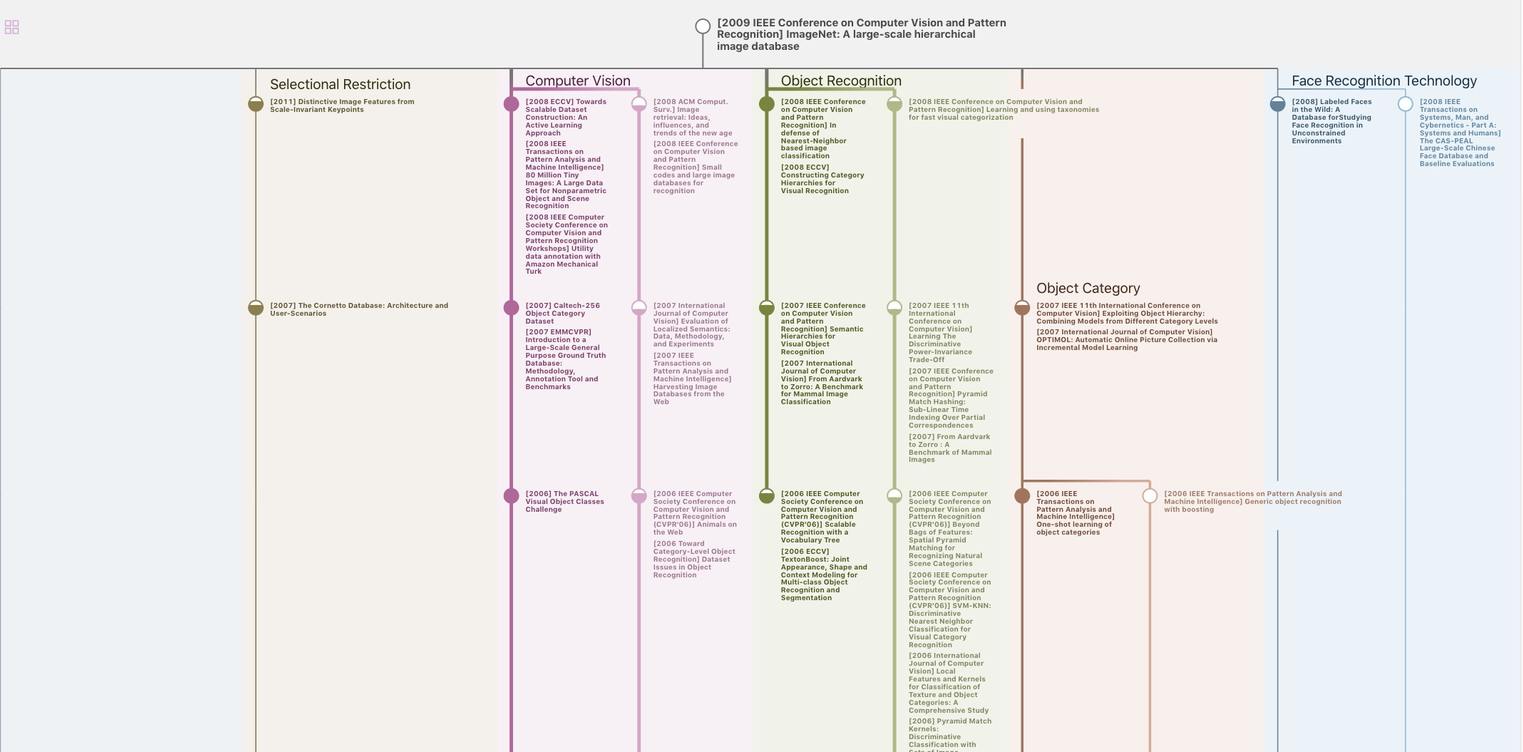
生成溯源树,研究论文发展脉络
Chat Paper
正在生成论文摘要