Network embedding with class discriminability
Proceedings of the ACM Turing Celebration Conference - China(2019)
摘要
Network embedding, which learns low-dimensional representations from networks for network information preservation, has gained considerable attention in recent years. Network embedding has been shown to outperform many traditional node representation learning methods on the tasks such as clustering, classification and visualization. However, focusing on preserving the proximity between nodes, most previous works ignore many prominent features of networks. Some recent methods contrive to preserve cluster (class) structure in network by making representations closer to each other for nodes in the same class and enhancing the discriminability of cluster structure, which can result in better performance on various tasks. In this paper, we propose a simple and general model which can be extended to most network embedding models, especially NMF-based methods, to make the representations more discriminative for preserving cluster structure and therefore improve their results. In the experiments, we employ our model to extend an original and basic algorithm dating back to the early 2000s as a simple example. Comparison results with state-of-the-art algorithms have confirmed the effectiveness of our model.
更多查看译文
关键词
class discriminability, dimension reduction, feature learning, network embedding
AI 理解论文
溯源树
样例
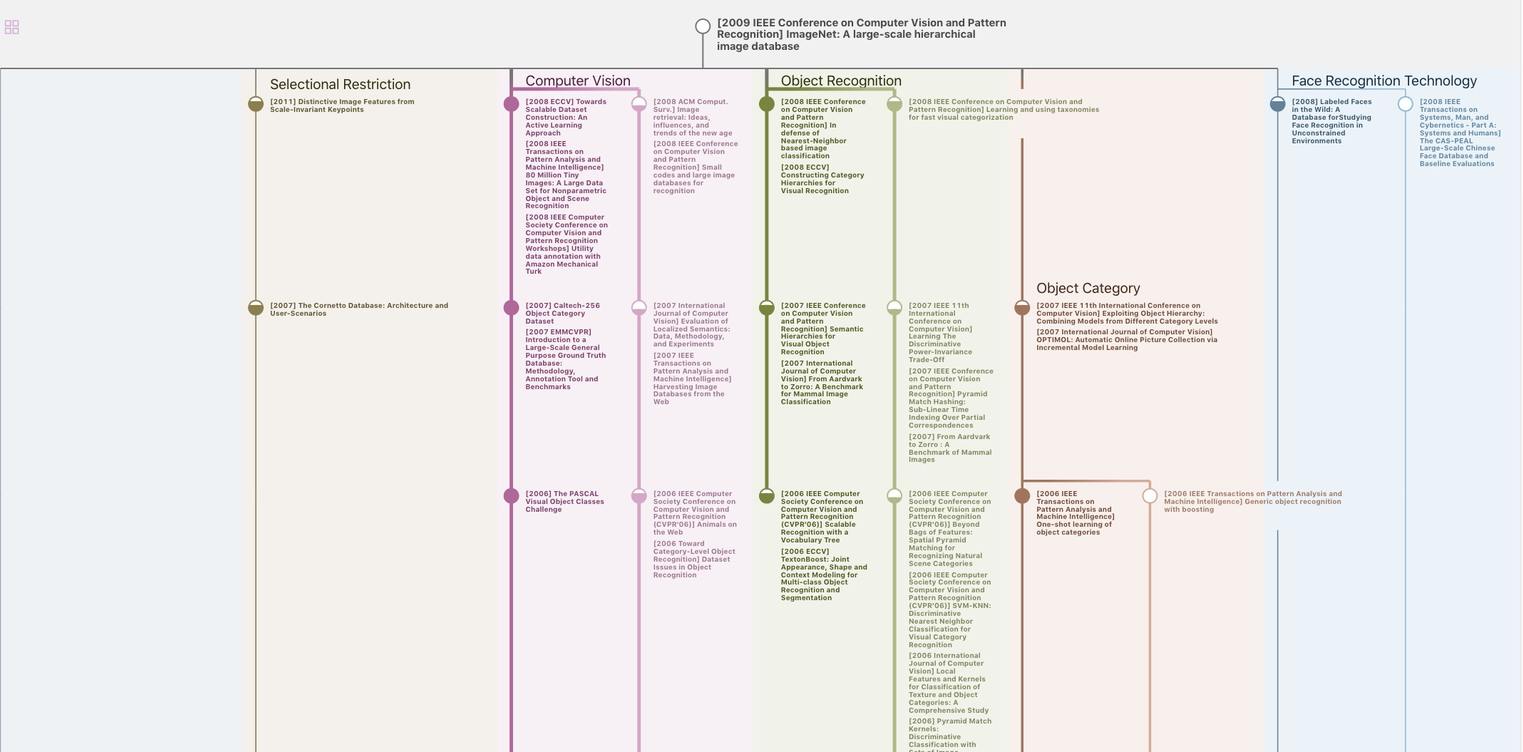
生成溯源树,研究论文发展脉络
Chat Paper
正在生成论文摘要