Identification of specificity TCR groups of tumor antigen specific T-cells
Cancer immunology research(2019)
摘要
A major breakthrough in the treatment of advanced melanoma has been the development and FDA approval of immune checkpoint inhibitors. Approximately 20% of the patients who received anti-CTLA-4 or anti-PD-1 therapy have long-term remissions. At the core of the clinical success lies the fact that cancer patients bear T lymphocytes that recognize tumor-specific antigens. It has been proposed that efficacy of immune checkpoint inhibitors is attributable, at least in part, to diversification of T-cell receptor (TCR) repertoire in peripheral circulation. However, current TCR repertoire analyses mostly rely on counting unique TCR sequences, which does not consider the degenerate nature of TCR recognition, that is, many different TCRs can recognize the same antigen peptide and the same TCR can recognize different antigen peptides. This will create roadblocks to identify recurrent TCR clones that likely recognize the same clinically relevant tumor antigen. To understand how TCR sequences encode its specificity, our lab sequenced several thousands of TCRs from T-cells enriched by peptide-major histocompatibility complex multimer (pMHC)-guided cell sorting. We established a TCR repertoire database that contains our own sequences and published TCRs with known specificity. We used this database as the training dataset for a machine learning algorithm that classified TCRs (mainly TCR beta) based on their similarity and specificity. Although the orginal algorithm—termed Grouping of lymphocyte interactions by paratope hotspots(GLIPH)—was proficient in identifying TCR clusters in a small dataset such as those generated by single-cell TCR sequences, it failed to perform reliably when applied to larger data set generated by high-throughput bulk TCR beta sequences. Most importantly, the reference sequences used by GLIPH 1.0 are insufficient when the targeted TCR dataset is much larger. The new version of GLIPH (GLIPH 2.0) was significantly improved in performance and reliability. As a proof of concept, we applied GLIPH 2.0 to TCR-sequences generated from peripheral T-cells of a cohort of melanoma patients. Each of these patients received one infusion of poly-clonal CTLs specific for HLA-A2/MART1. These autologous MART-specific CTLs were generated by priming with MART1 peptide-pulsed dendritic cells and enriched by pMHC-tetramer-guided cell sorting. MART1 peptide stimulation promotes expansion of CTLs based on TCR clonality. However, the overlap of sequences among these polyclonal CTLs is marginal. These TCR sequences were then analyzed by GLIPH and we found that MART1-specific CTLs showed significant enrichment of CDR3 motifs. As a control, we analyzed peripheral TCR repertoire from a group HLA-A2+ healthy donors and found no significant convergence in TCR sequences. This proves that GLIPH 2.0 can reliably identify TCR convergence and antigen specificity CD3 motifs from large-scale TCR beta repertoires. This algorithm can further facilitate the identification of recurrent tumor antigens in melanoma patients receiving T-cell-based immunotherapy. Citation Format: Liang Chen, Chunlin Wang, Mark Davis. Identification of specificity TCR groups of tumor antigen specific T-cells [abstract]. In: Proceedings of the Fourth CRI-CIMT-EATI-AACR International Cancer Immunotherapy Conference: Translating Science into Survival; Sept 30-Oct 3, 2018; New York, NY. Philadelphia (PA): AACR; Cancer Immunol Res 2019;7(2 Suppl):Abstract nr PR14.
更多查看译文
AI 理解论文
溯源树
样例
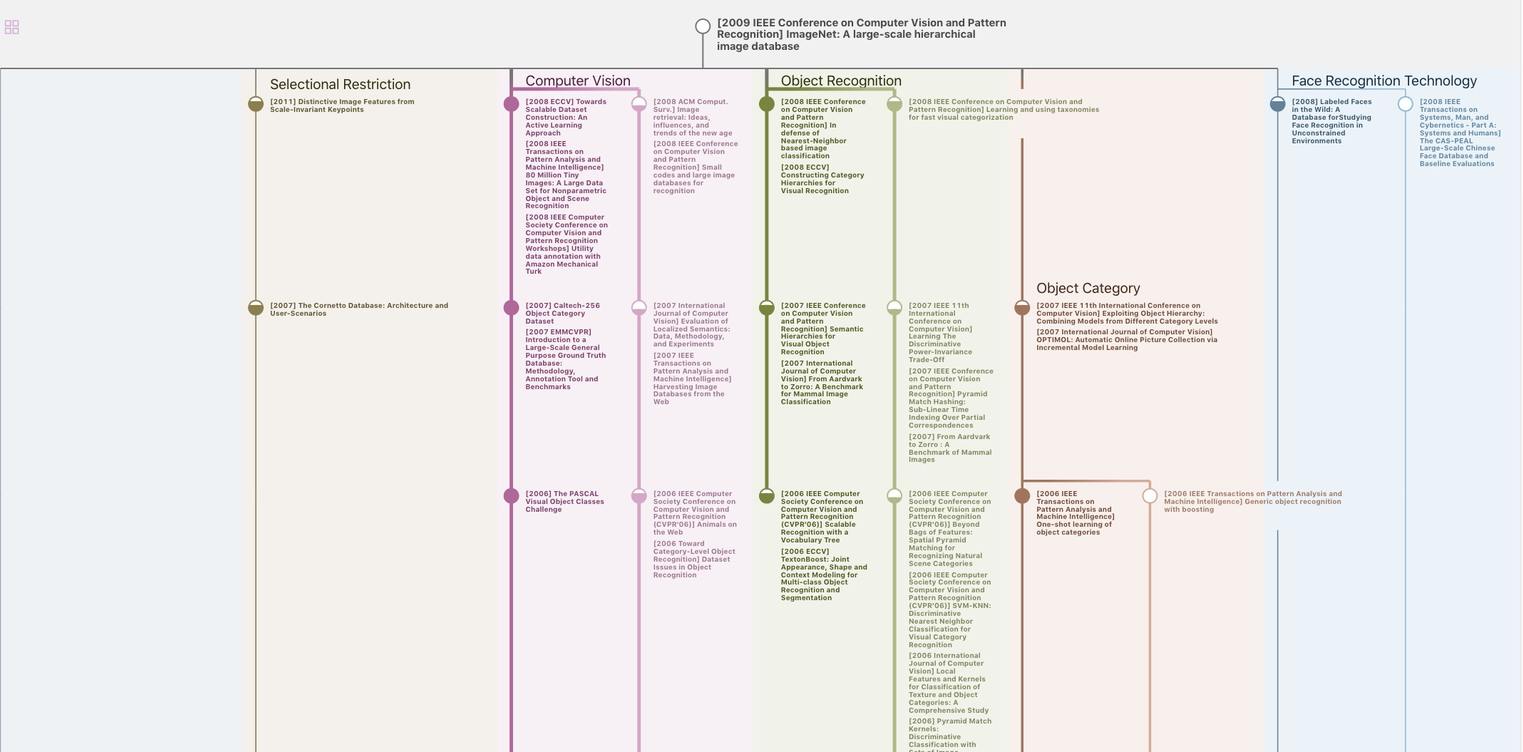
生成溯源树,研究论文发展脉络
Chat Paper
正在生成论文摘要