One minute is enough - Early Prediction of Student Success and Event-level Difficulty during Novice Programming Tasks.
EDM(2019)
摘要
Early prediction of student difficulty during long-duration learning activities allows a tutoring system to intervene by providing needed support, such as a hint, or by alerting an instructor. To be effective, these predictions must come early and be highly accurate, but such predictions are difficult for open-ended programming problems. In this work, Recent Temporal Patterns (RTPs) are used in conjunction with Support Vector Machine and Logistic Regression to build robust yet interpretable models for early predictions. We performed two tasks: to predict student success and difficulty during one, open-ended novice programming task of drawing a square-shaped spiral. We compared RTP against several machine learning models ranging from the classic to the more recent deep learning models such as Long Short Term Memory to predict whether students would be able to complete the programming task. Our results show that RTP-based models outperformed all others, and could successfully classify students after just one minute of a 20minute exercise (students can spend more than 1 hour on it). To determine when a system might intervene to prevent incompleteness or eventual dropout, we applied RTP at regular intervals to predict whether a student would make progress within the next five minutes, reflecting that they may be having difficulty. RTP successfully classified these students needing interventions over 85% of the time, with increased accuracy using data-driven program features. These results contribute significantly to the potential to build a fully data-driven tutoring system for novice programming.
更多查看译文
关键词
programming,student success,early prediction,event-level
AI 理解论文
溯源树
样例
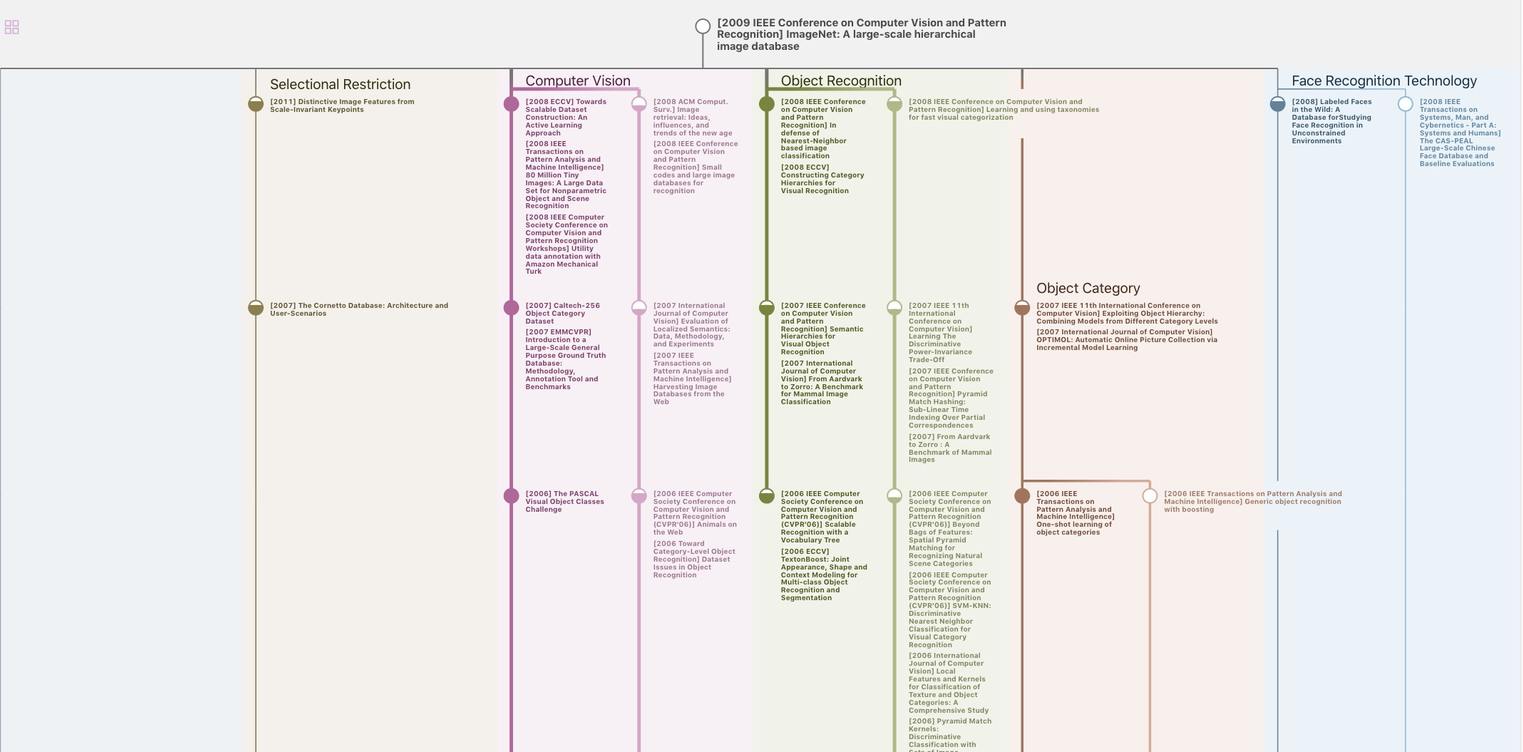
生成溯源树,研究论文发展脉络
Chat Paper
正在生成论文摘要