Learning Explainable Entity Resolution Algorithms for Small Business Data using SystemER
Proceedings of the 5th Workshop on Data Science for Macro-modeling with Financial and Economic Datasets(2019)
摘要
The 2019 FEIII CALI data challenge aims at linking different representations of the same real-world entities across multiple public datasets that collect identification and activity data about small to medium enterprises (SMEs) in California. We formalize this challenge as a learning-based entity resolution (ER) task, the goal of which is to learn a high-precision and high-recall pair-wise ER model that classifies small business entity pairs into matches and non-matches. Realistic ER tasks usually involve a pipeline of laborintensive and error-prone tasks, such as data preprocesing, gathering of training data, feature engineering, and model tuning. In this task, we apply an advanced human-in-the-loop system, named SystemER, to learn ER algorithms for SME entities. Powered by active learning and via a carefully designed user interface, SystemER can learn high-quality explainable ER algorithms with low human effort, while achieving high-accuracy on the datasets provided by the FEIII CALI data challenge.
更多查看译文
关键词
Entity resolution, SystemER, human-in-the-loop, small business
AI 理解论文
溯源树
样例
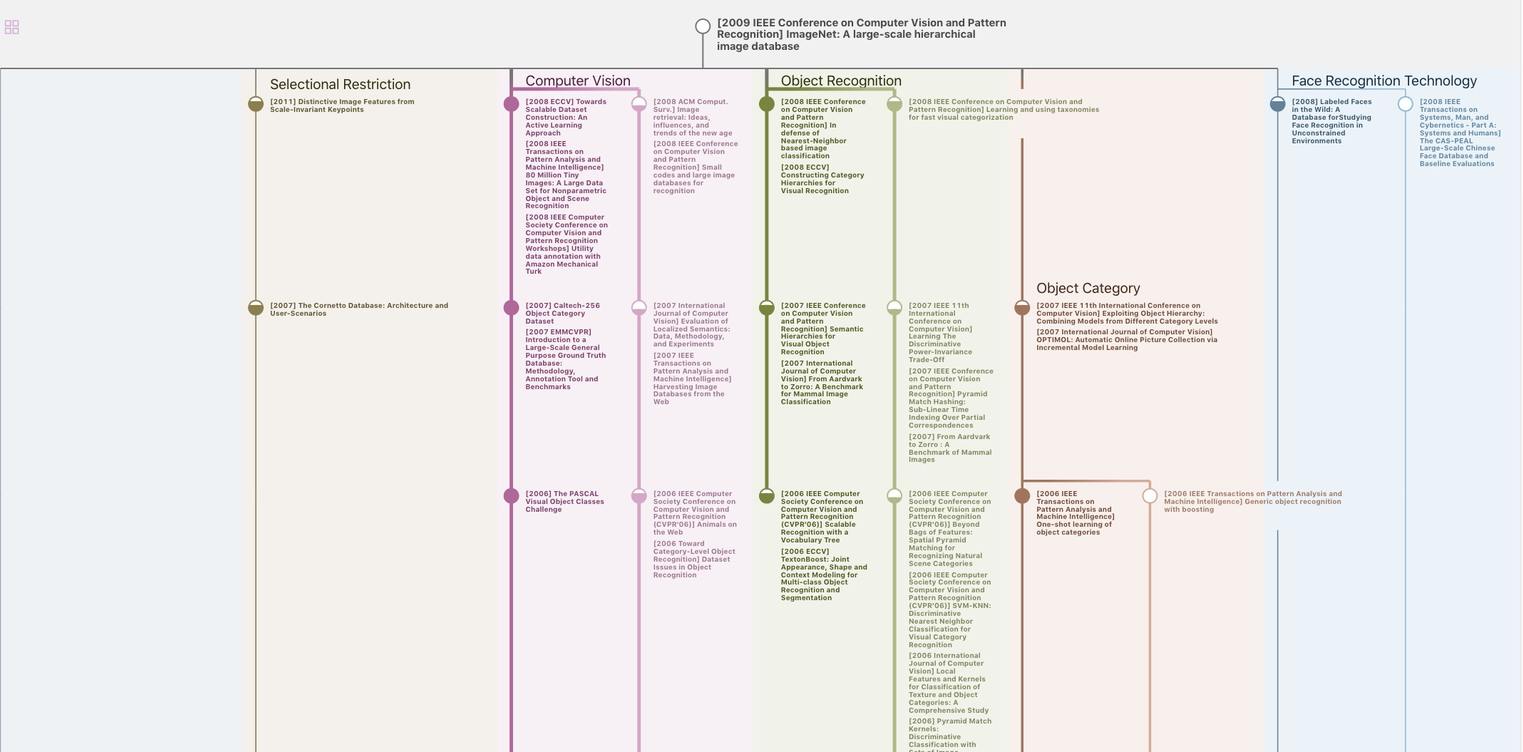
生成溯源树,研究论文发展脉络
Chat Paper
正在生成论文摘要