CSLI: Cost-Sensitive Collaborative Filtering with Local Information Embedding.
ROUGH SETS, IJCRS 2019(2019)
摘要
Mean absolute error and root mean square error are typically used to evaluate the accuracy of recommender system. However, these evaluation metrics implicitly mean that the cost of different wrong recommendation actions is the same. In this paper, we propose the cost-sensitive collaborative filtering with local information embedding (CSLI) algorithm to handle unequal misclassification costs. First, we employ a clustering algorithm to extract local rating information. Second, we design a collaborative filtering algorithm embedding local rating information to compute the prediction p. Third, we construct a 2 x 2 cost matrix by considering different misclassification costs. We employ the trichotomy method to obtain the recommendation threshold r(t) with the cost matrix. Finally, the recommendation actions are determined based on p and r(t). Combined with the cost matrix, we calculate the average misclassification cost and use it to evaluate the performance of the CSLI algorithm. Experimental results show that the proposed algorithm is lower than the state-of-the-art ones in term of average cost.
更多查看译文
关键词
Collaborative filtering, Cost-sensitive, Local rating information, Misclassification cost
AI 理解论文
溯源树
样例
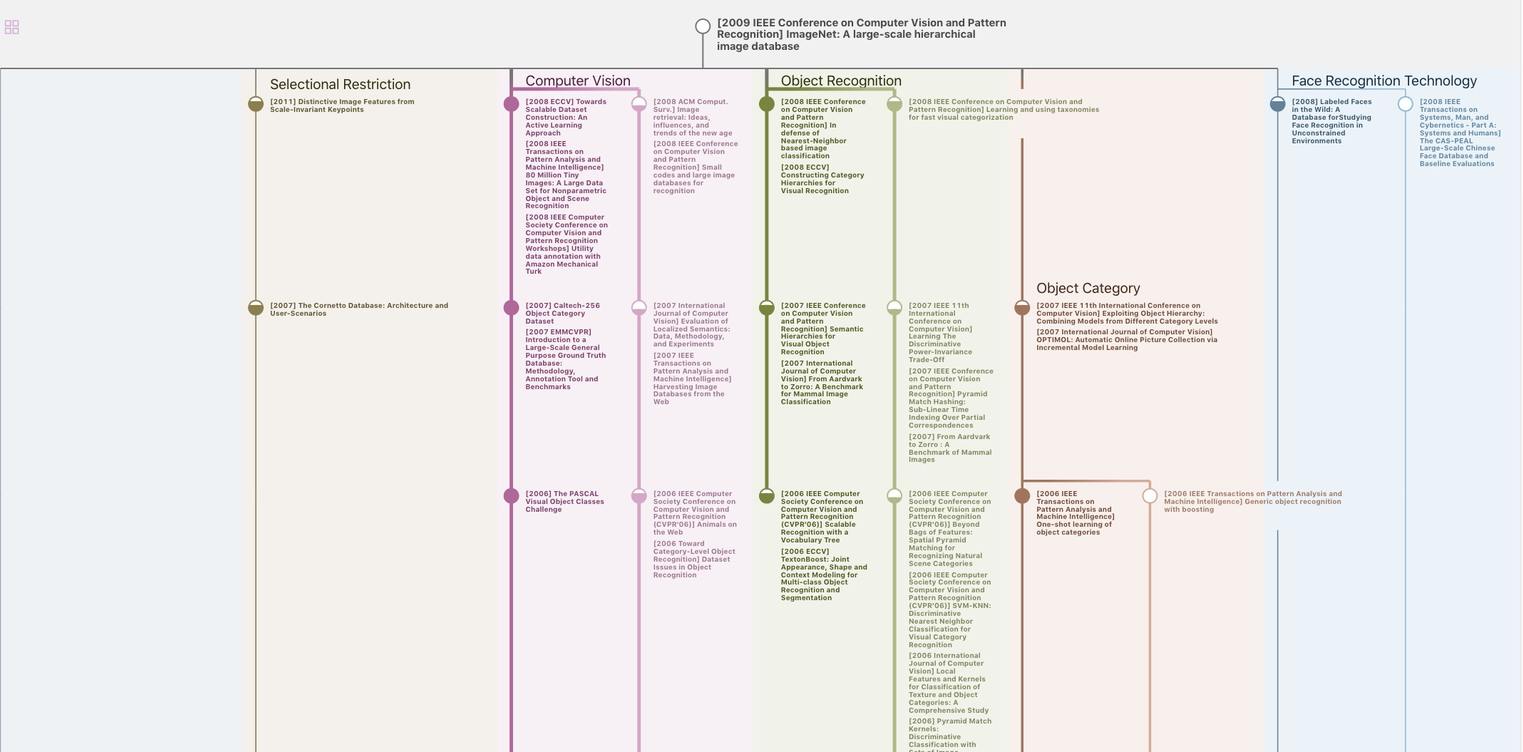
生成溯源树,研究论文发展脉络
Chat Paper
正在生成论文摘要