Automatic Hyperparameter Optimization for Transfer Learning on Medical Image Datasets Using Bayesian Optimization
2019 13th International Symposium on Medical Information and Communication Technology (ISMICT)(2019)
摘要
In the medical field of gastroenterology, deep learning is being explored and utilized in computer-aided diagnosis (CAD) systems. These systems assist physicians in the diagnosis of diseases and anomalies using visual data from endoscopic examinations. Deep learning has proven effective in the field [15]-[21], [23]. However, hyperparameter optimization is usually performed manually, taking a long time and with a chance of not finding the best parameters for classification accuracy. Using transfer learning, we aim for high accuracy in anomaly detection, and this paper describes a system for automatic hyperparameter optimization of convolutional neural network (CNN) models in Keras [4]. The presented system utilizes Bayesian optimization and is used to present experiments with three optimization strategies automatically optimizing hyperparameters for CNN models on two gastrointestinal datasets. Between the strategies, one was successful in achieving a high validation accuracy, while the others were considered failures. Compared to the best approaches in related work, our best models were around 10% better. With these experiments, we demonstrated that automatic hyperparameter optimization is an effective strategy for increasing performance in transfer learning.
更多查看译文
关键词
hyperparameter optimization,bayesian optimization,keras,medical images,gastrointestinal tract,gpyopt
AI 理解论文
溯源树
样例
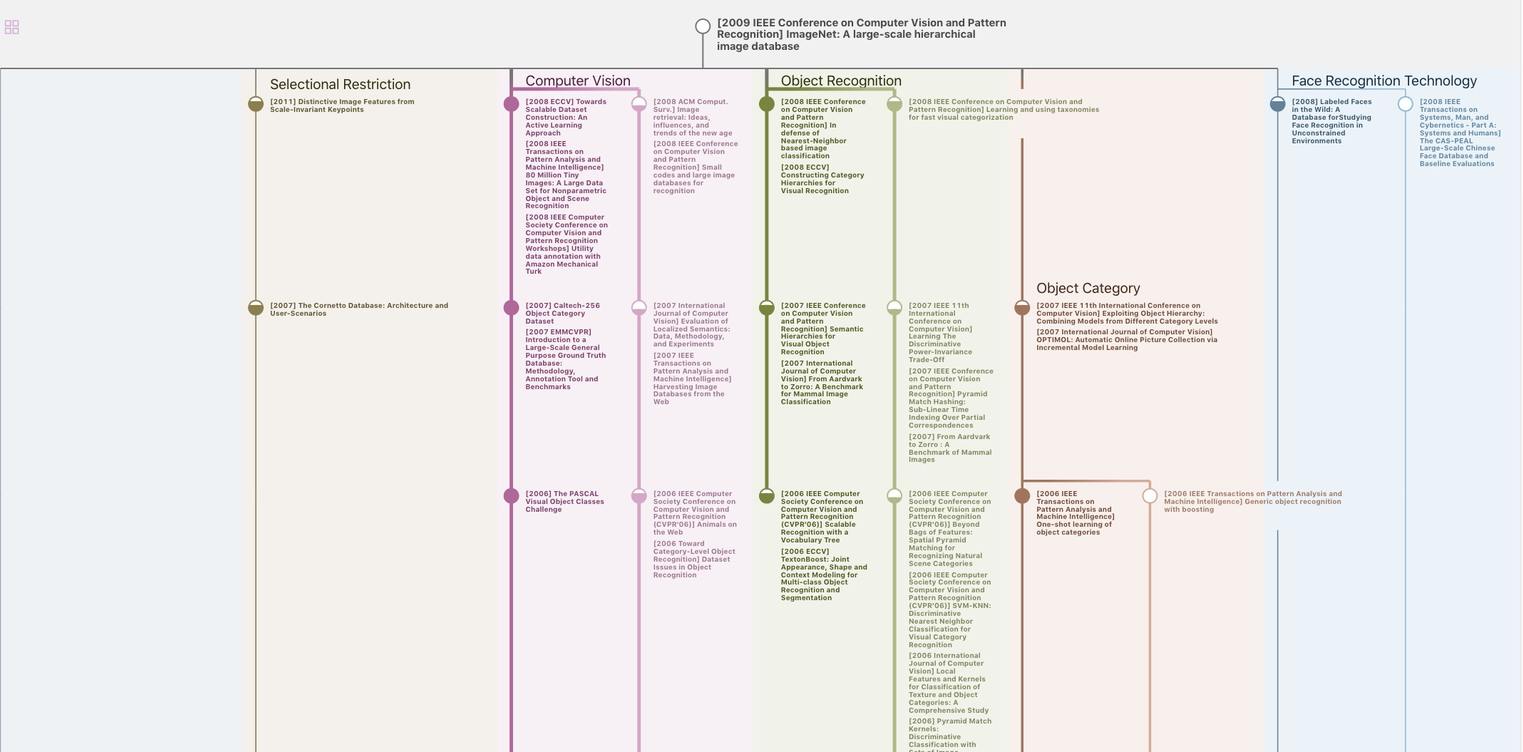
生成溯源树,研究论文发展脉络
Chat Paper
正在生成论文摘要