Automated Non-contact Detection of Head and Body Positions during Sleep
IEEE ACCESS(2019)
摘要
Obstructive sleep apnea (OSA) is a respiratory disorder characterized by interruption to breathing during sleep. Usually, the OSA is more severe in the supine sleeping position. Recent studies also demonstrated that the head position may play an important role in the pathophysiology of the OSA. Therefore, monitoring the sleeping body and the head position has high clinical importance to optimize the treatment of the OSA. In this paper, three machine learning approaches were used to detect the head position during sleep in infrared images. In the first two methods, supervised classifiers were trained to estimate the head position based on different feature sets extracted from infrared images. In the third method, three different convolutional neural network (CNN) structures (ResNet50, MobileNet, and Darknet19) were trained to detect the head position during sleep. To detect the body position, the same CNN architectures were trained on infrared images. Overnight sleeping data (sleep duration = 5 +/- 1 h) from 50 participants (age: 53 +/- 15 years, BMI: 29 +/- 6 kg/m2, and 30 men/20 women) with various levels of OSA severity as measured by the apnea-hypopnea index (AHI = 25 +/- 29 events/h and OSA severity: 12 normal, 13 mild, 11 moderate, and 14 severe) were collected for this paper. The models were trained on the data collected in one laboratory room from half of the participants and tested on the data from the other half collected in a different laboratory room. The best performing model (Darknet19) correctly estimated the lateral versus supine head position with 92% accuracy and 94% F1-Score and the lateral versus supine body position with 87% accuracy and 87% F1-Score.
更多查看译文
关键词
Computer vision,machine learning,position detection,sleep apnea,non-contact monitoring
AI 理解论文
溯源树
样例
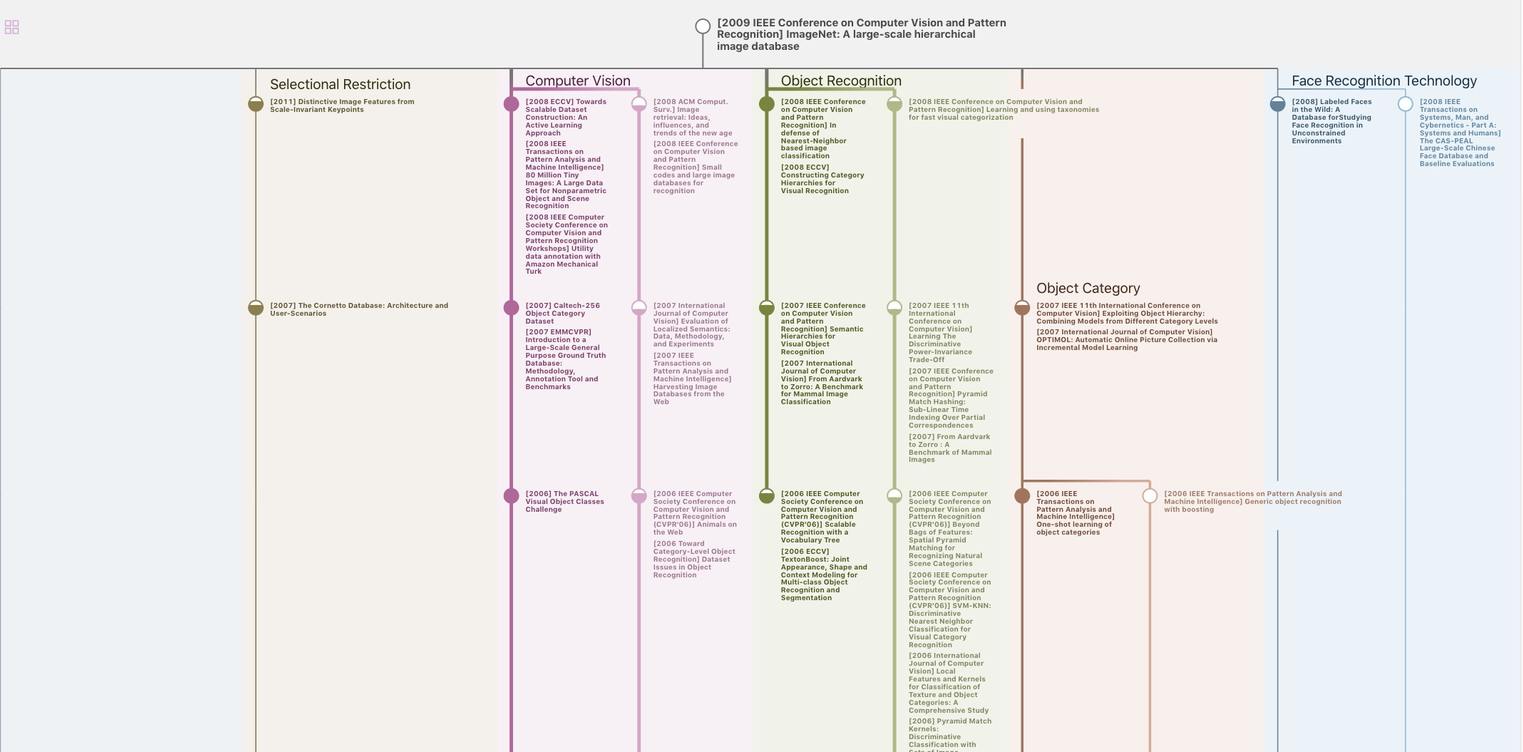
生成溯源树,研究论文发展脉络
Chat Paper
正在生成论文摘要