Reach-to-grasp motions: Towards a dynamic classification approach for upper-limp prosthesis
2019 9th International IEEE/EMBS Conference on Neural Engineering (NER)(2019)
摘要
During reach-to-grasp motions, the Electromyo-graphic (EMG) activity of the arm varies depending on motion stage. The variability of the EMG signals results in low classification accuracy during the reaching phase, delaying the activation of the prosthesis. To increase the efficiency of the pattern-recognition system, we investigate the muscle activity of four individuals with below-elbow amputation performing reach-to-grasp motions and segment the arm-motion into three phases with respect to the extension of the arm. Furthermore, we model the dynamic muscle contractions of each class with Gaussian distributions over the different phases and the overall motion. We quantify of the overlap among the classes with the Hellinger distance and notice larger values and, thus, smaller overlaps among the classes with the segmentation to motion phases. A Linear Discriminant Analysis classifier with phase segmentation affects positively the classification accuracy by 6 - 10% on average.
更多查看译文
关键词
reach-to-grasp motions,dynamic classification approach,upper-limp prosthesis,motion stage,EMG signals results,low classification accuracy,reaching phase,muscle activity,arm-motion,motion phases,electromyographic activity
AI 理解论文
溯源树
样例
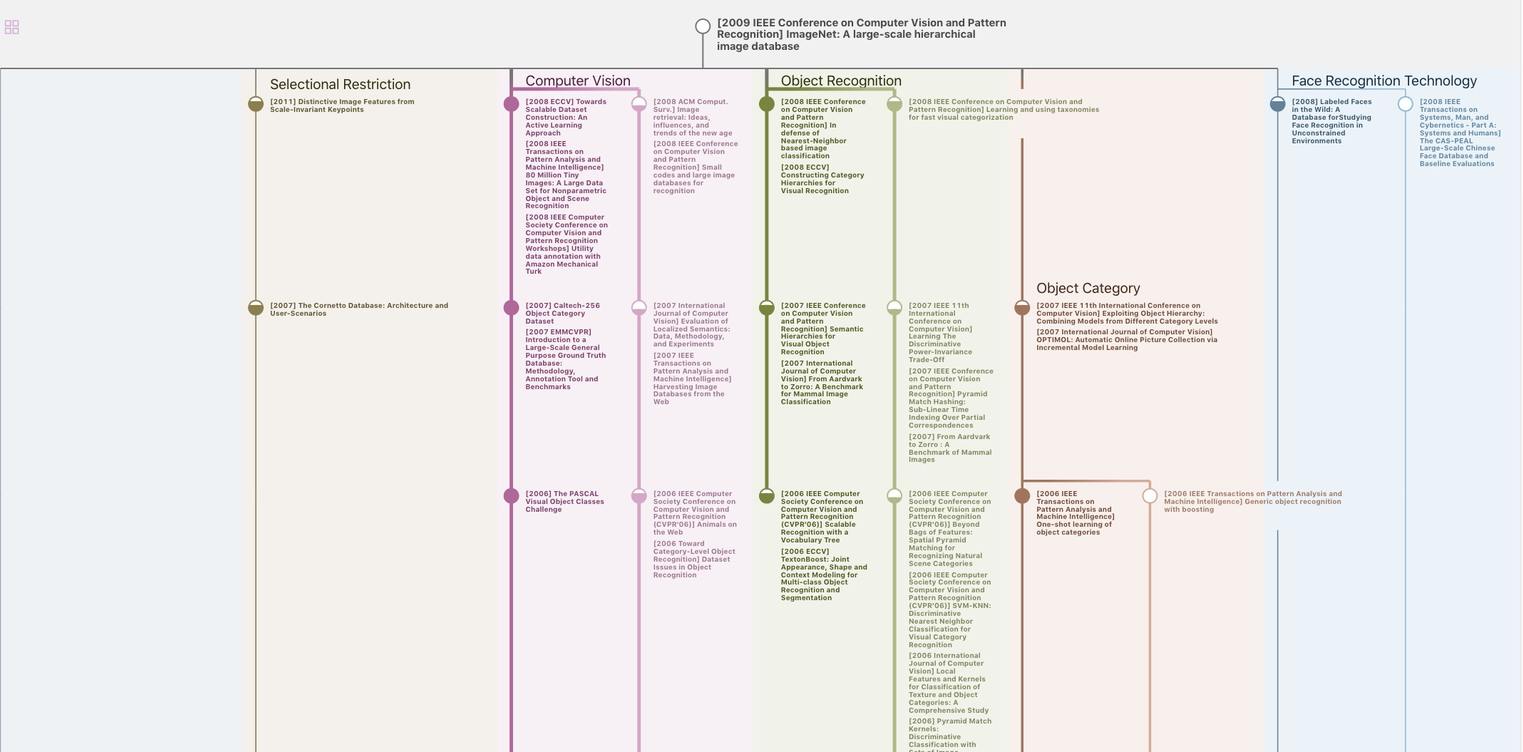
生成溯源树,研究论文发展脉络
Chat Paper
正在生成论文摘要