User preference modeling based on meta paths and diversity regularization in heterogeneous information networks
Knowledge-Based Systems(2019)
摘要
Recommendation methods based on heterogeneous information networks (HINs) have been attracting increased attention recently. Meta paths in HINs represent different types of semantic relationships. Meta path-based recommendation methods aim to use meta paths in HINs to evaluate the similarity or relevancy between nodes to make recommendations. In previous work, the meta paths have usually been selected manually (based on experience), and the path weight optimization methods usually suffer from overfitting. To solve these problems, we propose to automatically select and combine the meta paths through weight optimization. Diversity is introduced into the objective function as a regularization term to avoid overfitting. Inspired by the ambiguity decomposition theory in ensemble learning, we present a new diversity measure and use it to encourage diversity among meta paths to improve recommendation performance. Experimental results on item recommendation and tag recommendation tasks confirm the effectiveness of the proposed method compared with traditional collaborative filtering and state-of-the-art HIN-based recommendation methods.
更多查看译文
关键词
Recommender systems,Heterogeneous information network,Ensemble learning,Meta path,User preference
AI 理解论文
溯源树
样例
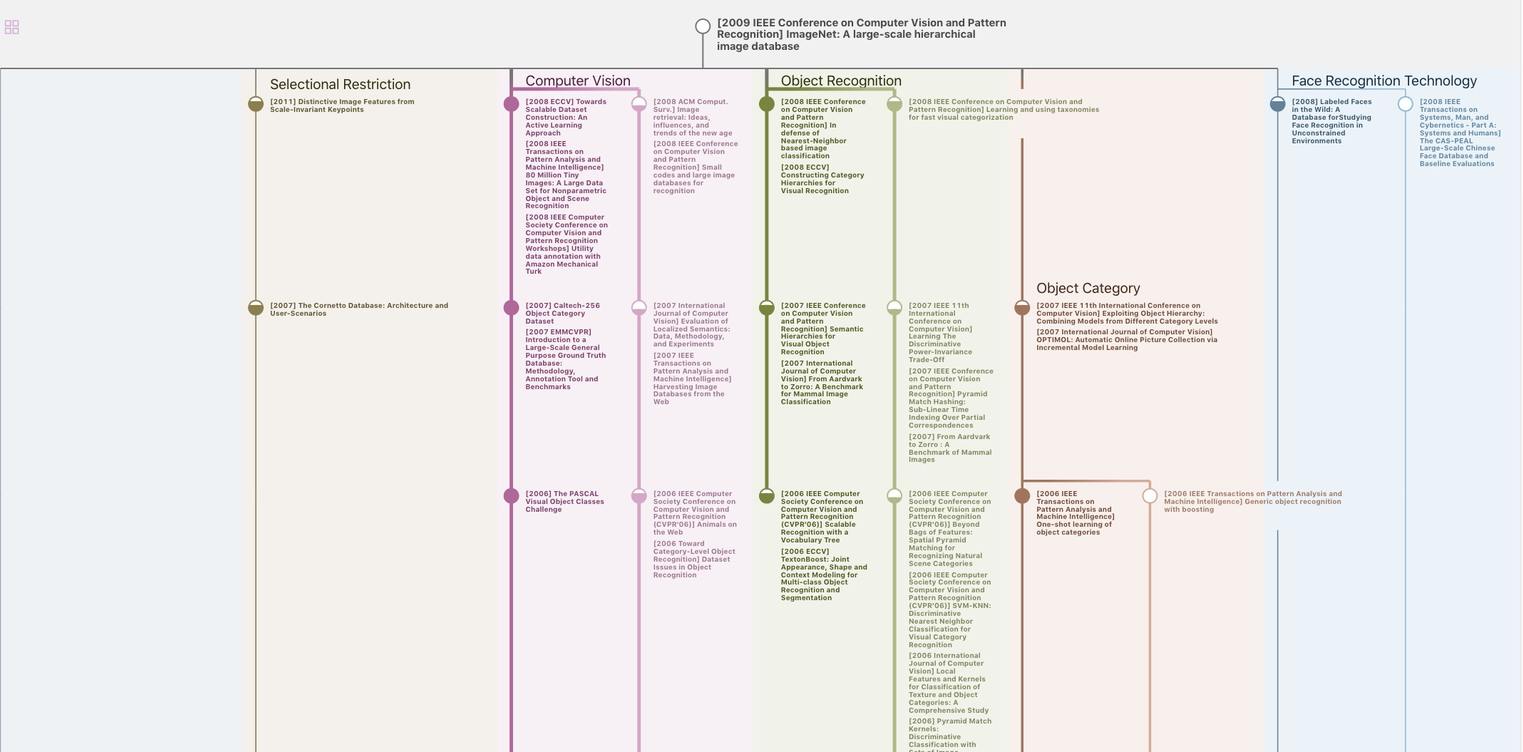
生成溯源树,研究论文发展脉络
Chat Paper
正在生成论文摘要