Tradeoffs in the Efficient Detection of Sign Language Content in Video Sharing Sites
ACM Transactions on Accessible Computing (TACCESS)(2019)
摘要
Video sharing sites have become keepers of de-facto digital libraries of sign language content, being used to store videos including the experiences, knowledge, and opinions of many in the deaf or hard of hearing community. Due to limitations of term-based search over metadata, these videos can be difficult to find, reducing their value to the community. Another result is that community members frequently engage in a push-style delivery of content (e.g., emailing or posting links to videos for others in the sign language community) rather than having access be based on the information needs of community members. In prior work, we have shown the potential to detect sign language content using features derived from the video content rather than relying on metadata. Our prior technique was developed with a focus on accuracy of results and are quite computationally expensive, making it unrealistic to apply them on a corpus the size of YouTube or other large video sharing sites. Here, we describe and examine the performance of optimizations that reduce the cost of face detection and the length of video segments processed. We show that optimizations can reduce the computation time required by 96%, while losing only 1% in F1 score. Further, a keyframe-based approach is examined that removes the need to process continuous video. This approach achieves comparable recall but lower precision than the above techniques. Merging the advantages of the optimizations, we also present a staged classifier, where the keyframe approach is used to reduce the number of non-sign language videos fully processed. An analysis of the staged classifier shows a further reduction in average computation time per video while achieving similar quality of results.
更多查看译文
关键词
ASL, Sign language, metadata extraction, video analysis, video sharing
AI 理解论文
溯源树
样例
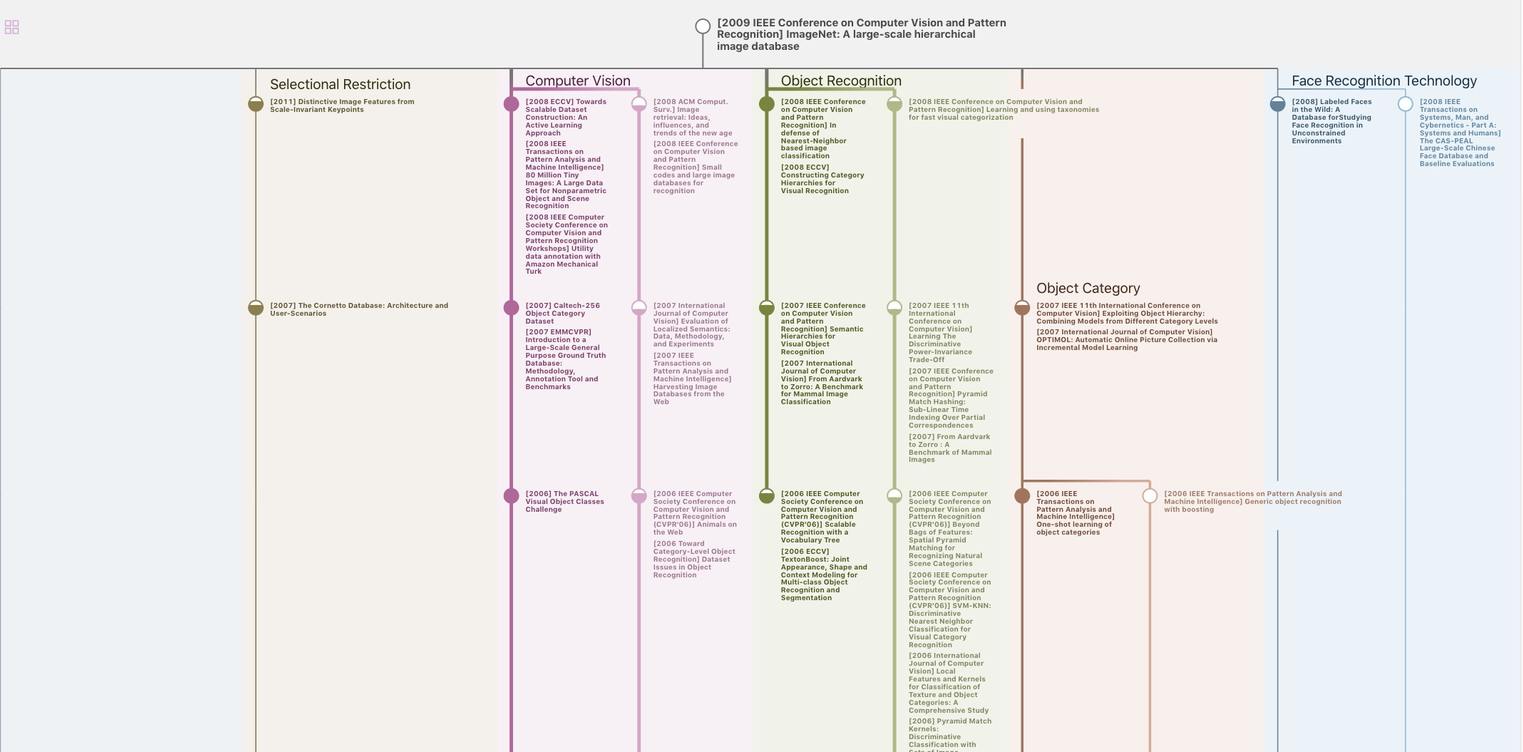
生成溯源树,研究论文发展脉络
Chat Paper
正在生成论文摘要