The Lipschitz Matrix: A Tool for Parameter Space Dimension Reduction.
arXiv: Numerical Analysis(2019)
摘要
Assuming a multivariate function is Lipschitz continuous is one way to arrive at the curse of dimensionality---the situation where the cost of tasks such as approximation, integration, and optimization grow exponentially in the input dimension of the function. Here we introduce the Lipschitz matrix, a generalization of the scalar Lipschitz constant that alters the norm in which the distance between points is measured. When this Lipschitz matrix is low-rank, we are able to avoid this curse by noting that the quantity of interest is a ridge function with a low-dimensional subspace (the range of the Lipschitz matrix) onto which we can restrict the input without loss. Even if the Lipschitz matrix is not low rank, it can still reduce the constant associated with these tasks compared the Lipschitz constant when the singular values of the Lipschitz matrix decay. In addition, the Lipschitz matrix has other uses: it can identify an active subspace for parameter space dimension reduction; it can define uncertainty and provide informative bounds in high-dimensional spaces; and it motivates a space filling design of experiments to minimize uncertainty. As there are a limited number of situations where the Lipschitz matrix can be identified analytically, we show that the Lipschitz matrix can be estimated by solving a semidefinite program using a finite number of function samples or gradients.
更多查看译文
AI 理解论文
溯源树
样例
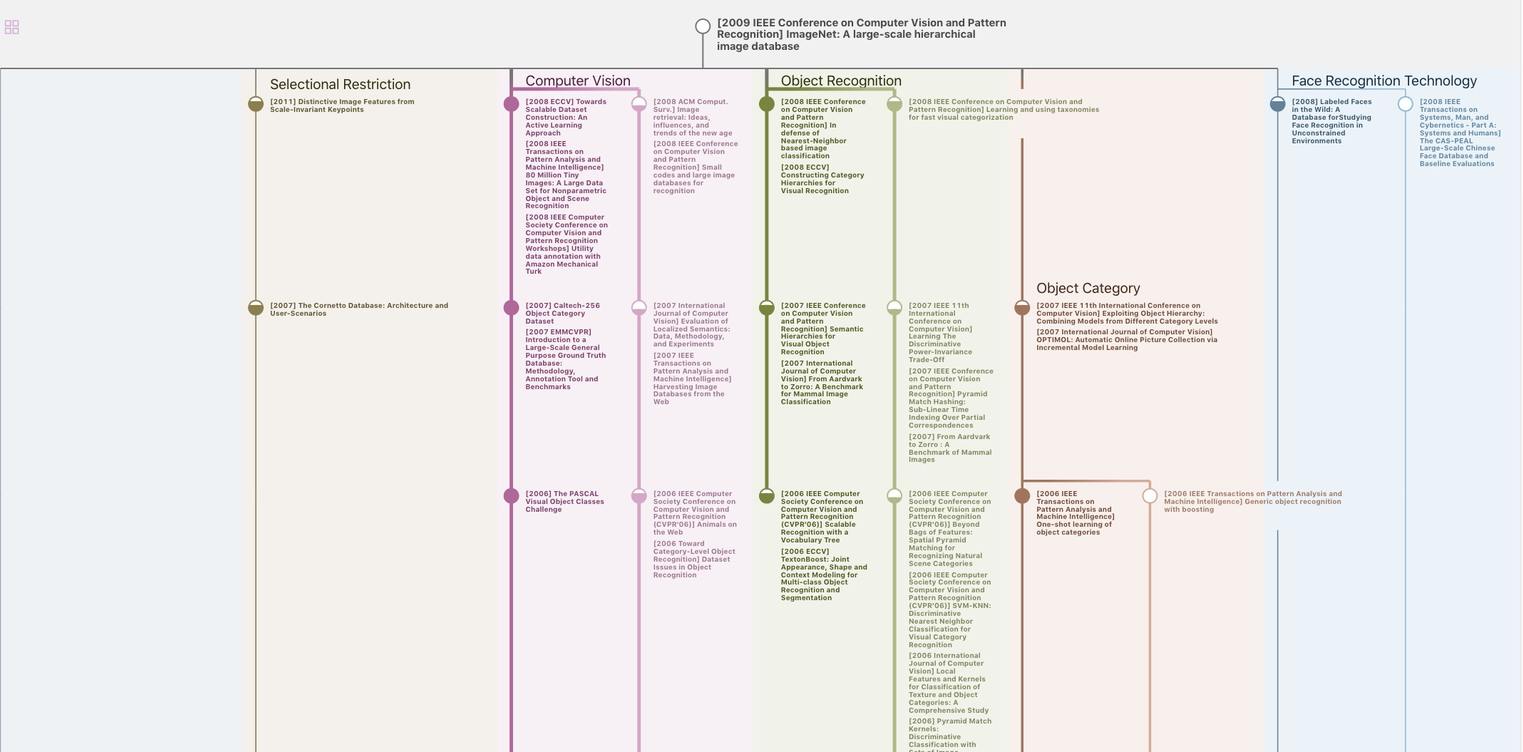
生成溯源树,研究论文发展脉络
Chat Paper
正在生成论文摘要