Multi-level Similarity Perception Network for Person Re-identification
ACM Transactions on Multimedia Computing, Communications, and Applications (TOMM)(2019)
摘要
In this article, we propose a novel deep Siamese architecture based on a convolutional neural network (CNN) and multi-level similarity perception for the person re-identification (re-ID) problem. According to the distinct characteristics of diverse feature maps, we effectively apply different similarity constraints to both low-level and high-level feature maps during training stage. Due to the introduction of appropriate similarity comparison mechanisms at different levels, the proposed approach can adaptively learn discriminative local and global feature representations, respectively, while the former is more sensitive in localizing part-level prominent patterns relevant to re-identifying people across cameras. Meanwhile, a novel strong activation pooling strategy is utilized on the last convolutional layer for abstract local-feature aggregation to pursue more representative feature representations. Based on this, we propose final feature embedding by simultaneously encoding original global features and discriminative local features. In addition, our framework has two other benefits: First, classification constraints can be easily incorporated into the framework, forming a unified multi-task network with similarity constraints. Second, as similarity-comparable information has been encoded in the network’s learning parameters via back-propagation, pairwise input is not necessary at test time. That means we can extract features of each gallery image and build an index in an off-line manner, which is essential for large-scale real-world applications. Experimental results on multiple challenging benchmarks demonstrate that our method achieves splendid performance compared with the current state-of-the-art approaches.
更多查看译文
关键词
CNN, Person re-identification, deep Siamese architecture, multi-level similarity perception
AI 理解论文
溯源树
样例
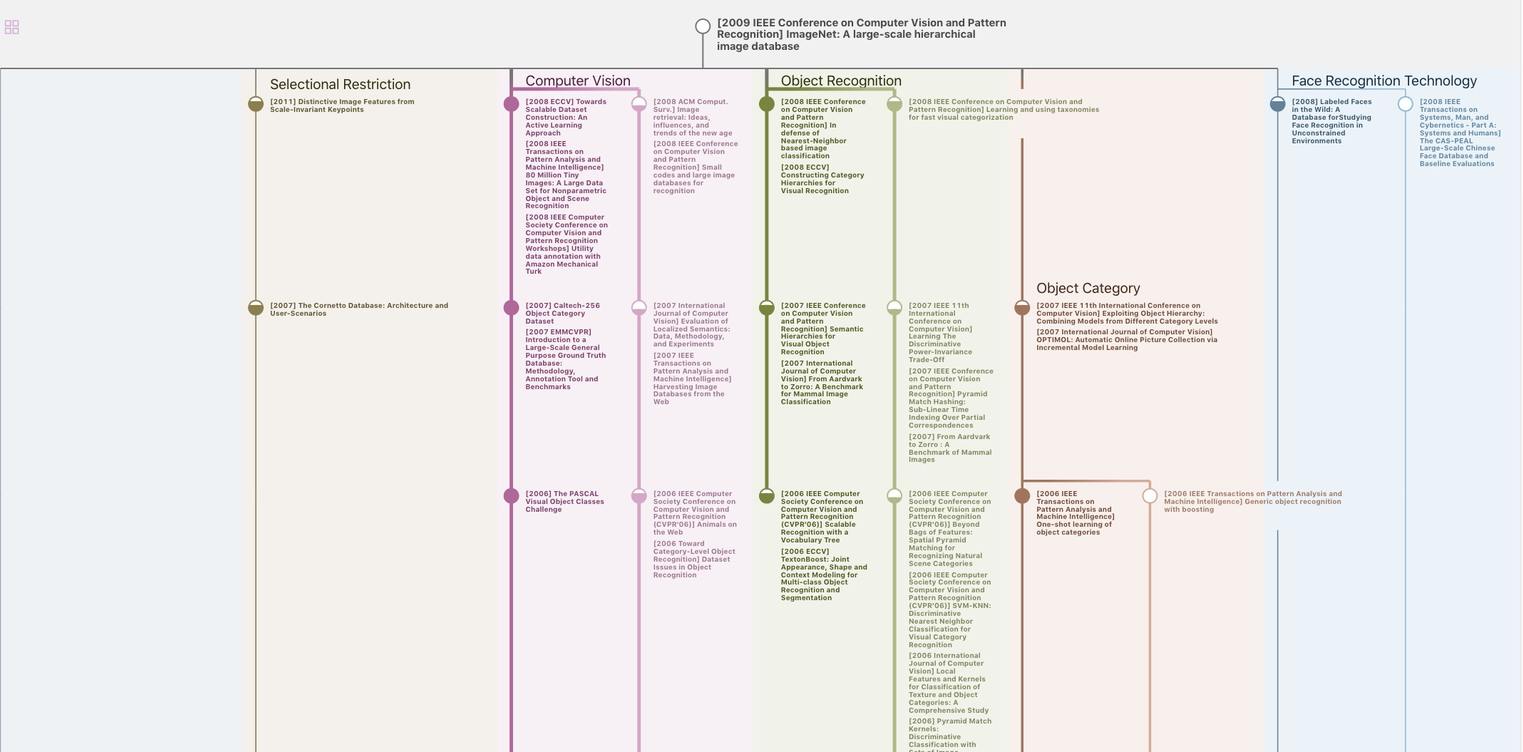
生成溯源树,研究论文发展脉络
Chat Paper
正在生成论文摘要