Rarely-switching linear bandits: optimization of causal effects for the real world.
arXiv: Machine Learning(2019)
摘要
Exploring the effect of policies in many real world scenarios is difficult, unethical, or expensive. After all, doctor guidelines, tax codes, and price lists can only be reprinted so often. We may thus want to only change a policy when it is probable that the change is beneficial. Fortunately, thresholds allow us to estimate treatment effects. Such estimates allows us to optimize the threshold. Here, based on the theory of linear contextual bandits, we present a conservative policy updating procedure which updates a deterministic policy only when needed. We extend the theory of linear bandits to this rarely-switching case, proving such procedures share the same regret, up to constant scaling, as the common LinUCB algorithm. However the algorithm makes far fewer changes to its policy. We provide simulations and an analysis of an infant health well-being causal inference dataset, showing the algorithm efficiently learns a good policy with few changes. Our approach allows efficiently solving problems where changes are to be avoided, with potential applications in economics, medicine and beyond.
更多查看译文
AI 理解论文
溯源树
样例
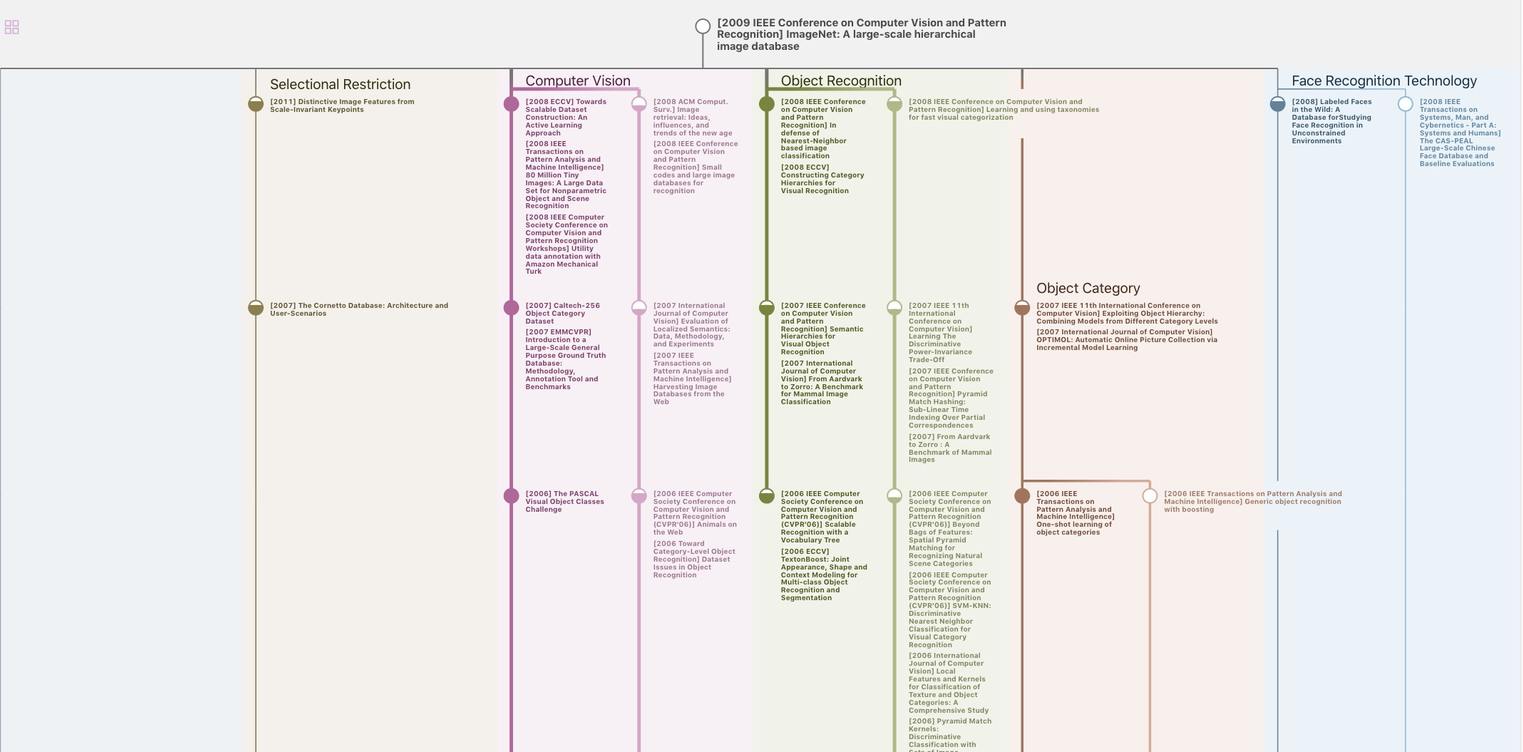
生成溯源树,研究论文发展脉络
Chat Paper
正在生成论文摘要