Mean-dependent nonstationary spatial models
arXiv: Methodology(2019)
摘要
Nonstationarity is a major challenge in analyzing spatial data. For example, daily precipitation measurements may have increased variability and decreased spatial smoothness in areas with high mean rainfall. Common nonstationary covariance models introduce parameters specific to each location, giving a highly-parameterized model which is difficult to fit. We develop a nonstationary spatial model that uses the mean to determine the covariance in a region, resulting in a far simpler, albeit more specialized, model. We explore inferential and predictive properties of the model under various simulated data situations. We show that this model in certain circumstances improves predictions compared to a standard stationary spatial model. We further propose a computationally efficient approximation that has comparable predictive accuracy. We also develop a test for nonstationary data and show it reliably identifies nonstationarity. We apply these methods to daily precipitation in Puerto Rico.
更多查看译文
AI 理解论文
溯源树
样例
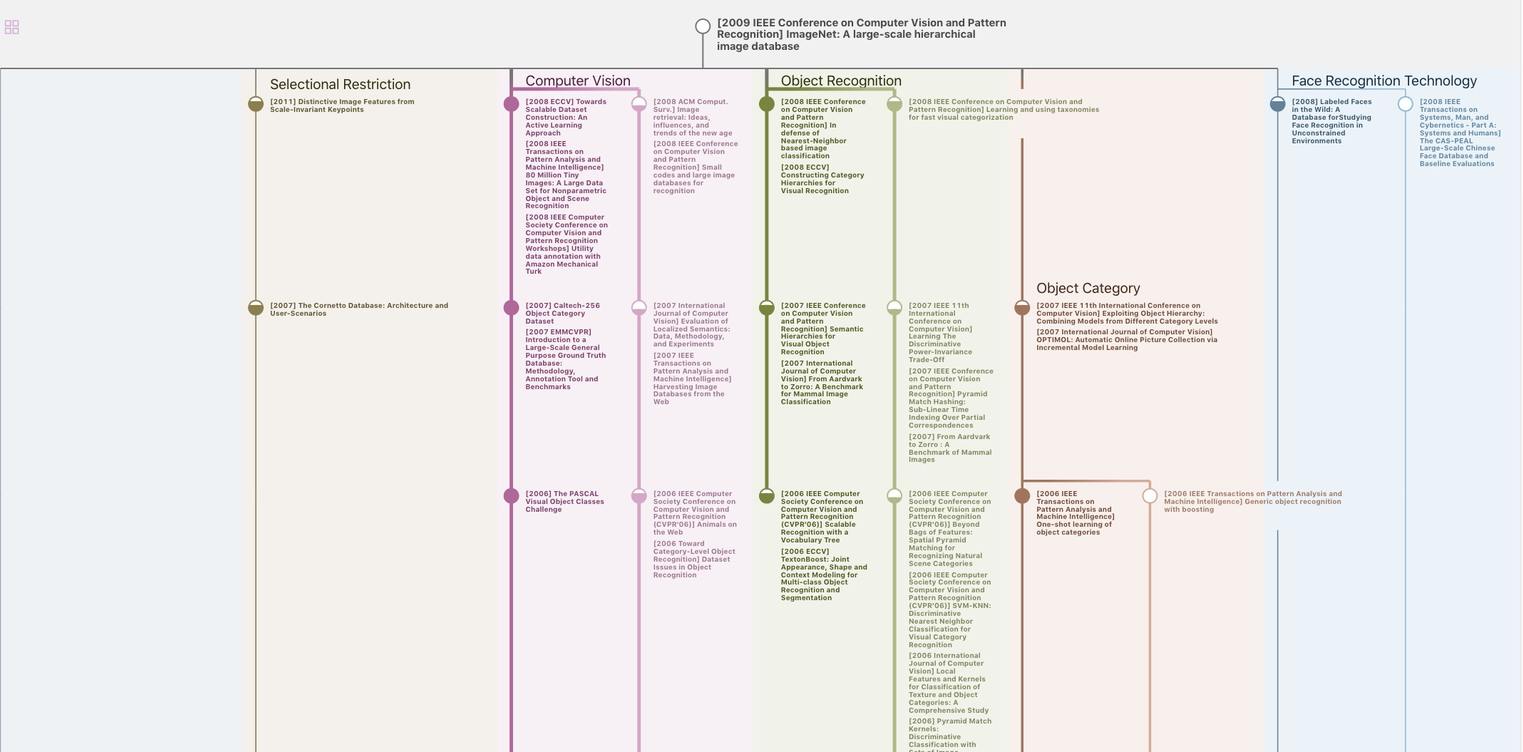
生成溯源树,研究论文发展脉络
Chat Paper
正在生成论文摘要