Large Scale Product Categorization using Structured and Unstructured Attributes.
arXiv: Information Retrieval(2019)
摘要
Product categorization using text data for eCommerce is a very challenging extreme classification problem with several thousands of classes and several millions of products to classify. Even though multi-class text classification is a well studied problem both in academia and industry, most approaches either deal with treating product content as a single pile of text, or only consider a few product attributes for modelling purposes. Given the variety of products sold on popular eCommerce platforms, it is hard to consider all available product attributes as part of the modeling exercise, considering that products possess their own unique set of attributes based on category. In this paper, we compare hierarchical models to flat models and show that in specific cases, flat models perform better. We explore two Deep Learning based models that extract features from individual pieces of unstructured data from each product and then combine them to create a product signature. We also propose a novel idea of using structured attributes and their values together in an unstructured fashion along with convolutional filters such that the ordering of the attributes and the differing attributes by product categories no longer becomes a modelling challenge. This approach is also more robust to the presence of faulty product attribute names and values and can elegantly generalize to use both closed list and open list attributes.
更多查看译文
关键词
large scale product categorization,unstructured attributes,structured
AI 理解论文
溯源树
样例
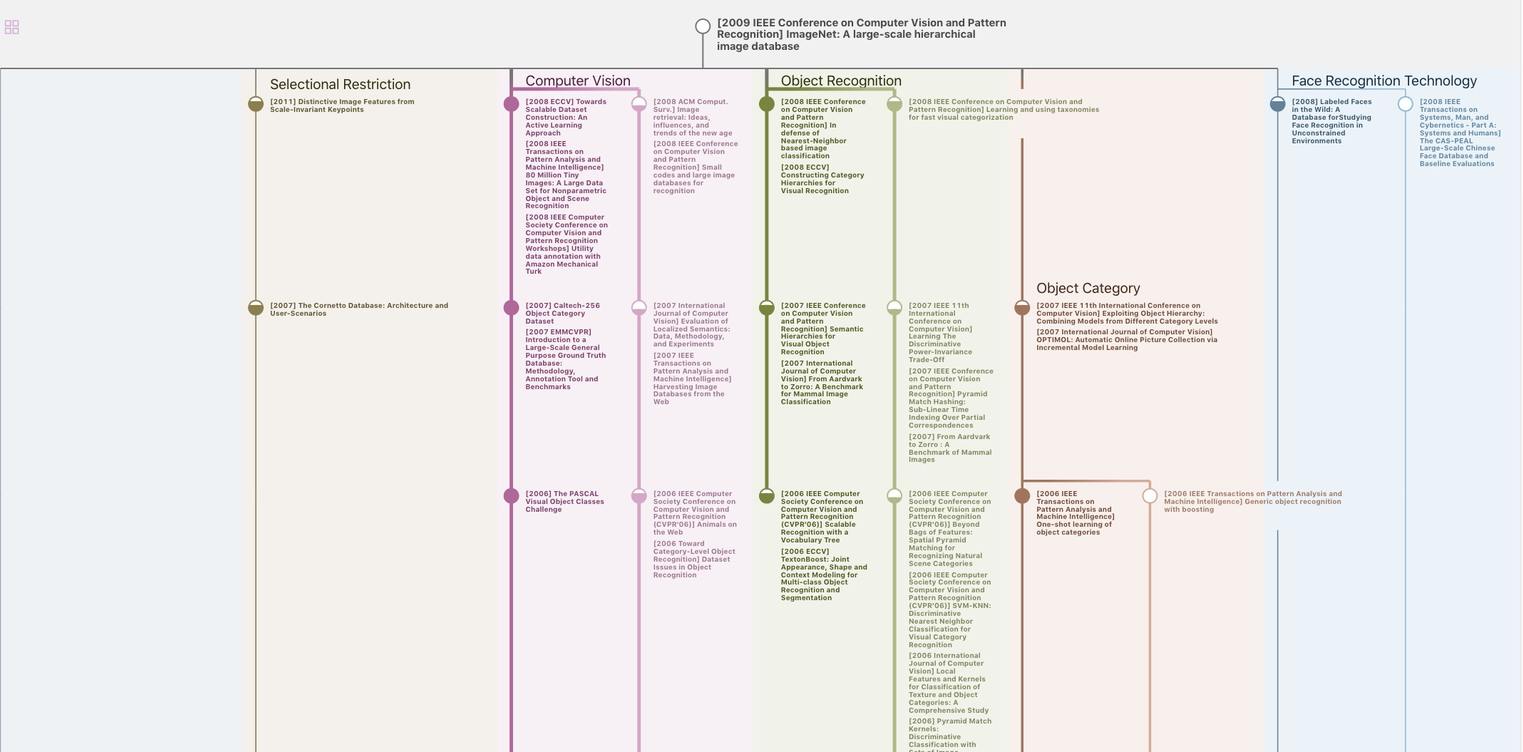
生成溯源树,研究论文发展脉络
Chat Paper
正在生成论文摘要