Mitigation of Adversarial Examples in RF Deep Classifiers Utilizing AutoEncoder Pre-training
2019 International Conference on Military Communications and Information Systems (ICMCIS)(2019)
摘要
Adversarial examples in machine learning for images are widely publicized and explored. Illustrations of misclassifications caused by these slightly perturbed inputs are abundant and commonly known (e.g., a picture of panda imperceptibly perturbed to fool the classifier into incorrectly labeling it as a gibbon). Similar attacks on deep learning (DL) for radio frequency (RF) signals and their mitigation strategies are scarcely addressed in the published work. Yet, RF adversarial examples (AdExs) with minimal waveform perturbations can cause drastic, targeted misclassification results, particularly against spectrum sensing/survey applications (e.g. BPSK is mistaken for 8-PSK). Our research on deep learning AdExs and proposed defense mechanisms are RF-centric, and incorporate physical-world, over-the-air (OTA) effects. We herein present defense mechanisms based on pre-training the target classifier using an autoencoder. Our results validate this approach as a viable mitigation method to subvert adversarial attacks against deep learning-based communications and radar sensing systems.
更多查看译文
关键词
radio frequency signals,minimal waveform perturbations,deep learning AdExs,defense mechanisms,RF-centric,viable mitigation method,adversarial attacks,deep learning-based communications,radar sensing systems,machine learning,RF deep classifiers,autoencoder pre-training,RF adversarial example mitigation strategy,over-the-air effects,target classifier pre-training,spectrum sensing
AI 理解论文
溯源树
样例
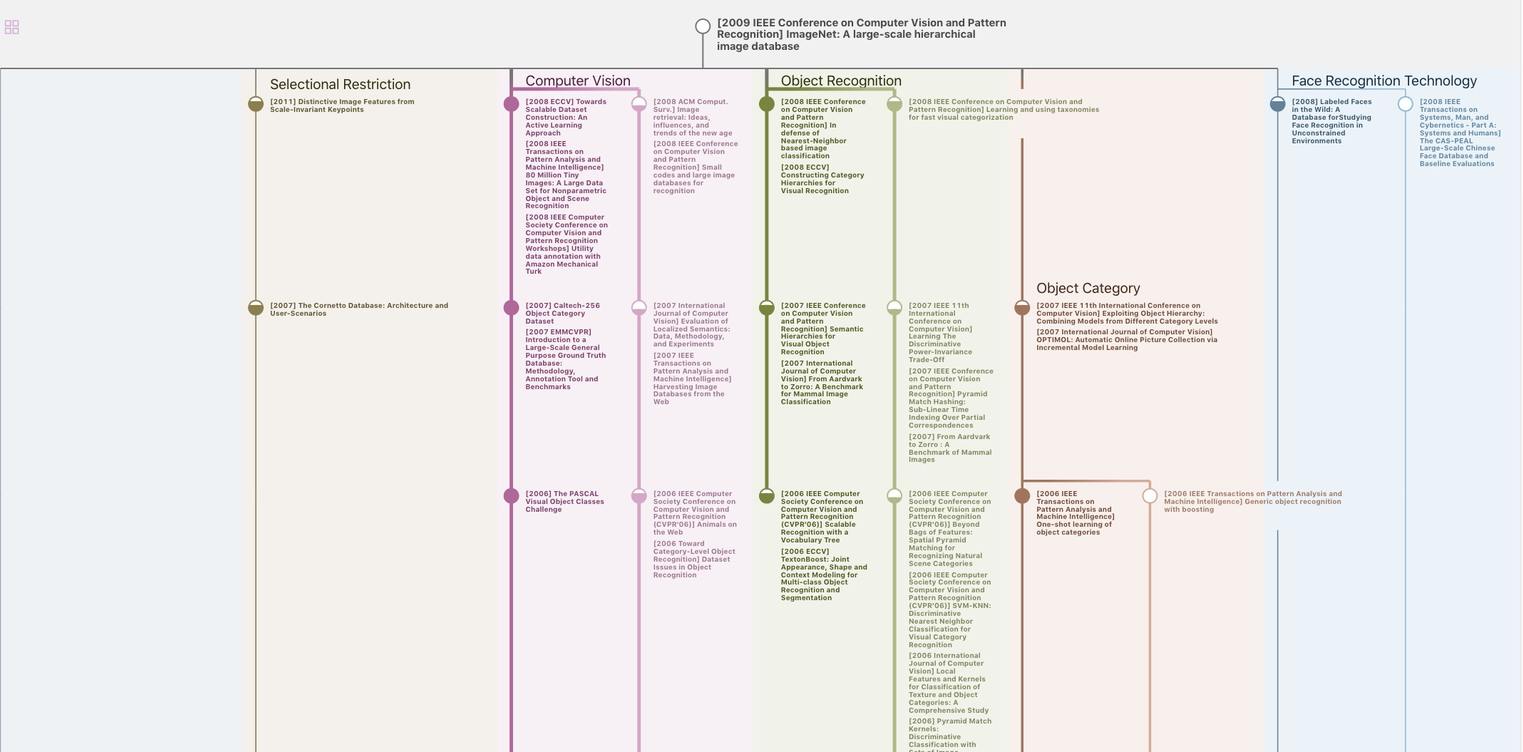
生成溯源树,研究论文发展脉络
Chat Paper
正在生成论文摘要