Batch virtual adversarial training for graph convolutional networks
arXiv: Learning(2023)
摘要
We present batch virtual adversarial training (BVAT), a novel regularization method for graph convolutional networks (GCNs). BVAT addresses the issue that GCNs do not ensure the smoothness of the model’s output distribution against local perturbations around the input node features. We propose two algorithms, sampling-based BVAT and optimization-based BVAT, which promote the output smoothness of GCN classifiers based on the generated virtual adversarial perturbations for either a subset of independent nodes or all nodes via an elaborate optimization process. Extensive experiments on three citation network datasets Cora, Citeseer and Pubmed and a knowledge graph dataset Nell validate the efficacy of the proposed method in semi-supervised node classification tasks.
更多查看译文
关键词
Virtual adversarial training,Graph convolutional networks,Semi-supervised node classification
AI 理解论文
溯源树
样例
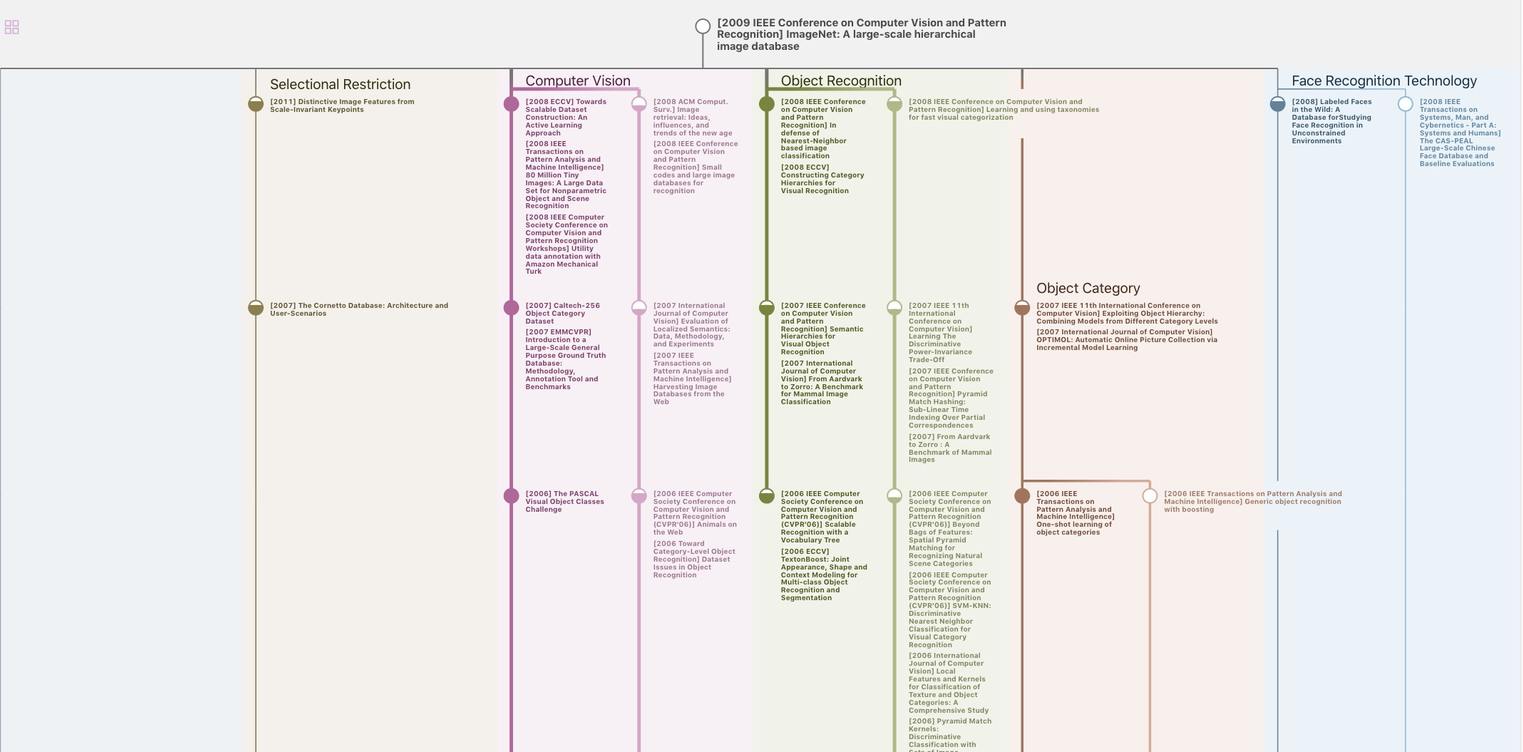
生成溯源树,研究论文发展脉络
Chat Paper
正在生成论文摘要