Automatic Hyperparameter Tuning Method For Local Outlier Factor, With Applications To Anomaly Detection
2019 IEEE International Conference on Big Data (Big Data)(2019)
摘要
Local outlier factor (LOF) is a state-of-the-art unsupervised machine learning model for anomaly detection in the Internet of Things (IoT). In recent years, there have been many important applications of LOF in large IoT systems, such as wireless transmission device system in high-speed trains, smart city vehicular social networks, industrial conditional monitoring systems, and network infrastructures for cyber security. LOF aims to forecast the imminent faults of an appliance in the IoT system, whose predictive performance greatly depends on the selection of its hyperparameters. The major challenge of hyperparameter tuning for unsupervised machine learning models including LOF in the context of IoT systems is that the incoming data may contain new types of anomaly that are unseen in the training data. In this paper, we propose a novel, heuristic methodology to tune the hyperparameters in IMF that accounts for this challenge. A tuned LOF model that uses the proposed method shows good predictive performance in both simulations and real data applications.
更多查看译文
关键词
local outlier factor,anomaly detection,hyperparameter tuning
AI 理解论文
溯源树
样例
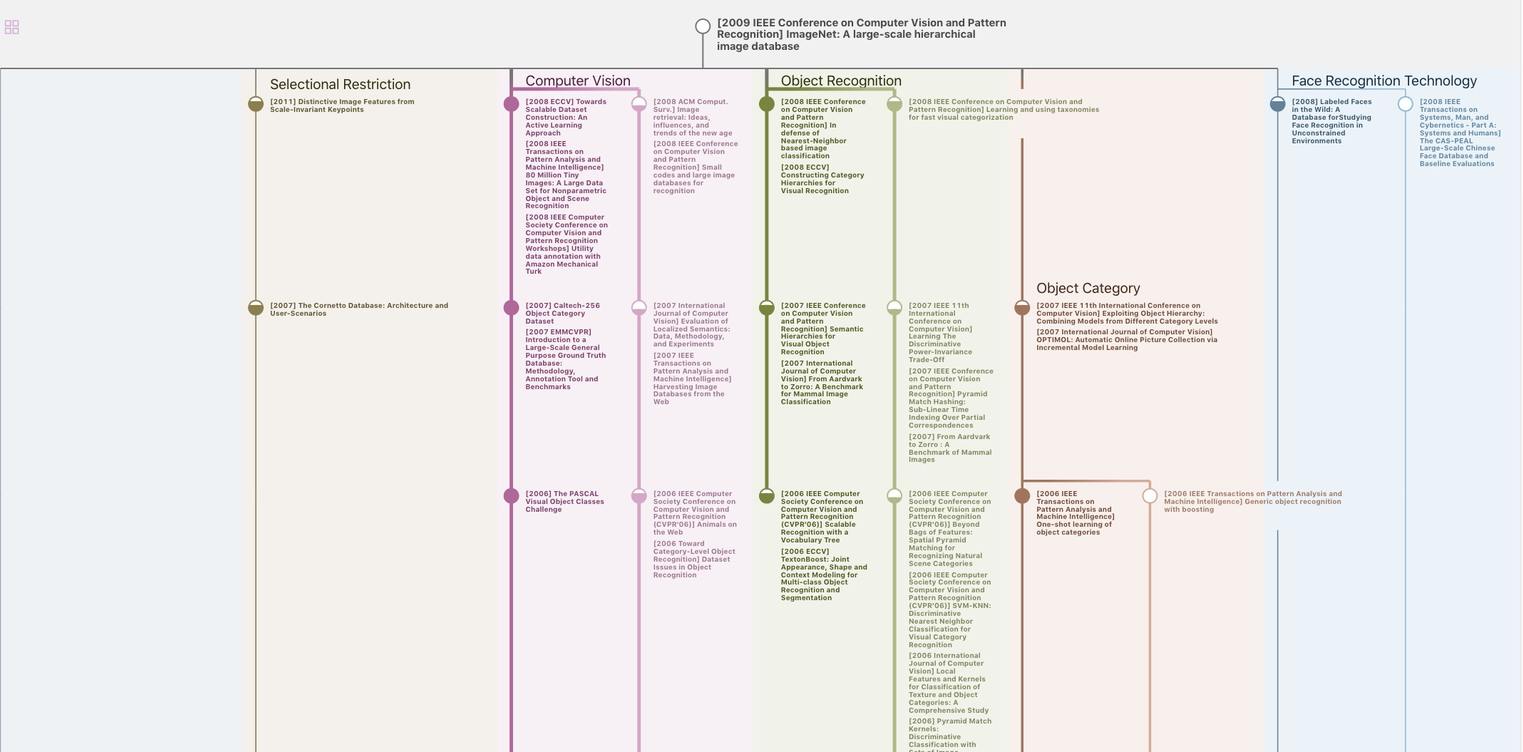
生成溯源树,研究论文发展脉络
Chat Paper
正在生成论文摘要