Data Requirements for Applying Machine Learning to Energy Disaggregation
ENERGIES(2019)
摘要
Energy disaggregation, or nonintrusive load monitoring (NILM), is a technology for separating a household's aggregate electricity consumption information. Although this technology was developed in 1992, its practical usage and mass deployment have been rather limited, possibly because the commonly used datasets are not adequate for NILM research. In this study, we report the findings from a newly collected dataset that contains 10 Hz sampling data for 58 houses. The dataset not only contains the aggregate measurements, but also individual appliance measurements for three types of appliances. By applying three classification algorithms (vanilla DNN (Deep Neural Network), ML (Machine Learning) with feature engineering, and CNN (Convolutional Neural Network) with hyper-parameter tuning) and a recent regression algorithm (Subtask Gated Network) to the new dataset, we show that NILM performance can be significantly limited when the data sampling rate is too low or when the number of distinct houses in the dataset is too small. The well-known NILM datasets that are popular in the research community do not meet these requirements. Our results indicate that higher quality datasets should be used to expedite the progress of NILM research.
更多查看译文
关键词
energy disaggregation,nonintrusive load monitoring (NILM),machine learning,data requirements
AI 理解论文
溯源树
样例
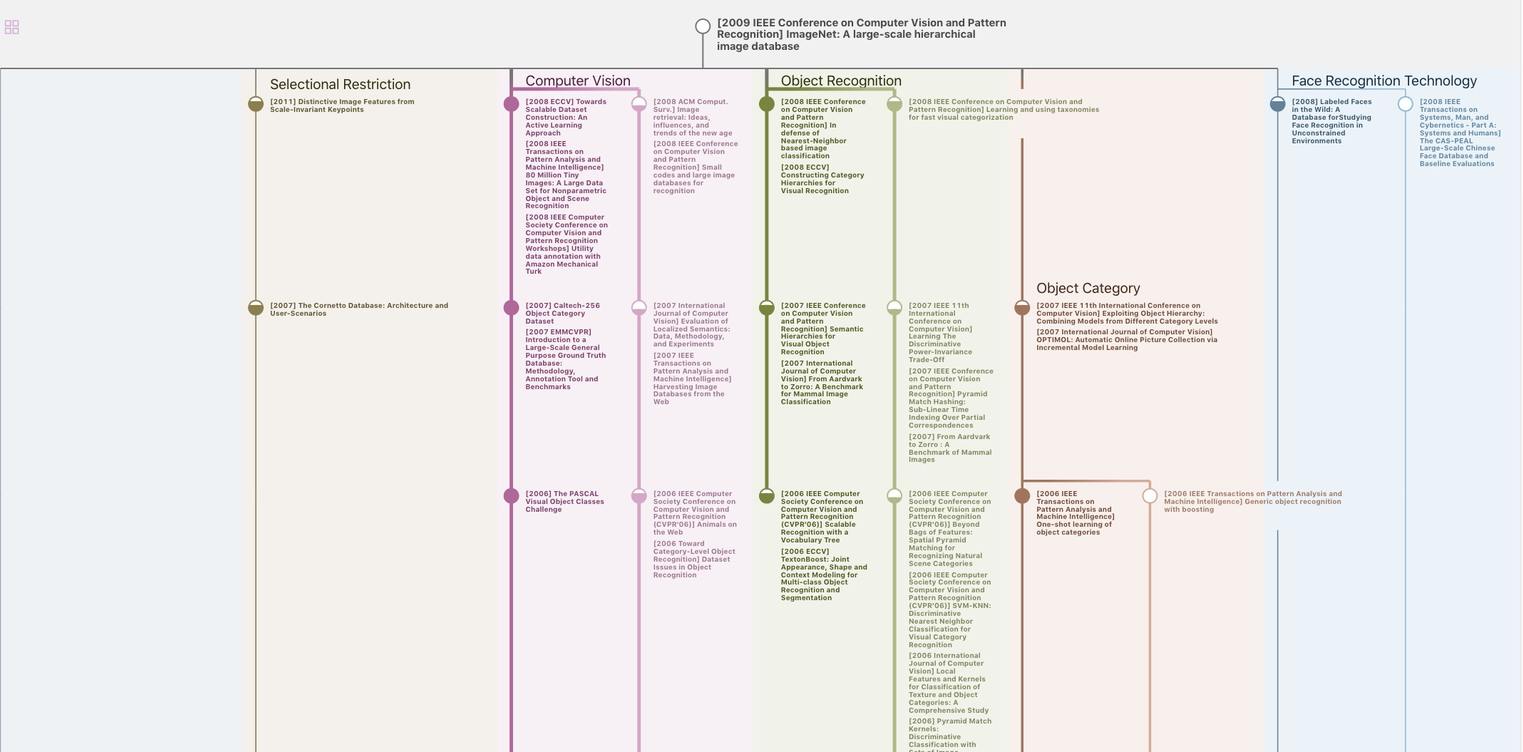
生成溯源树,研究论文发展脉络
Chat Paper
正在生成论文摘要