Learning to Learn: Hierarchical Meta-Critic Networks
IEEE ACCESS(2019)
摘要
In recent years, deep reinforcement learning methods have achieved impressive performance in many different fields, including playing games, robotics, and dialogue systems. However, there are still a lot of restrictions here, one of which is the demand for massive amounts of sampled data. In this paper, a hierarchical meta-learning method based on the actor-critic algorithm is proposed for sample efficient learning. This method provides the transferable knowledge that can efficiently train an actor on a new task with a few trials. Specifically, a global basic critic, meta critic, and task specified network are shared within a distribution of tasks and are capable of criticizing any actor trying to solve any specified task. The hierarchical framework is applied to a critic network in the actor-critic algorithm for distilling meta-knowledge above the task level and addressing distinct tasks. The proposed method is evaluated on multiple classic control tasks with reinforcement learning algorithms, including the start-of-the-art meta-learning methods. The experimental results statistically demonstrate that the proposed method achieves state-of-the-art performance and attains better results with more depth of meta critic network.
更多查看译文
关键词
Deep reinforcement learning,hierarchical framework,knowledge,meta-learning
AI 理解论文
溯源树
样例
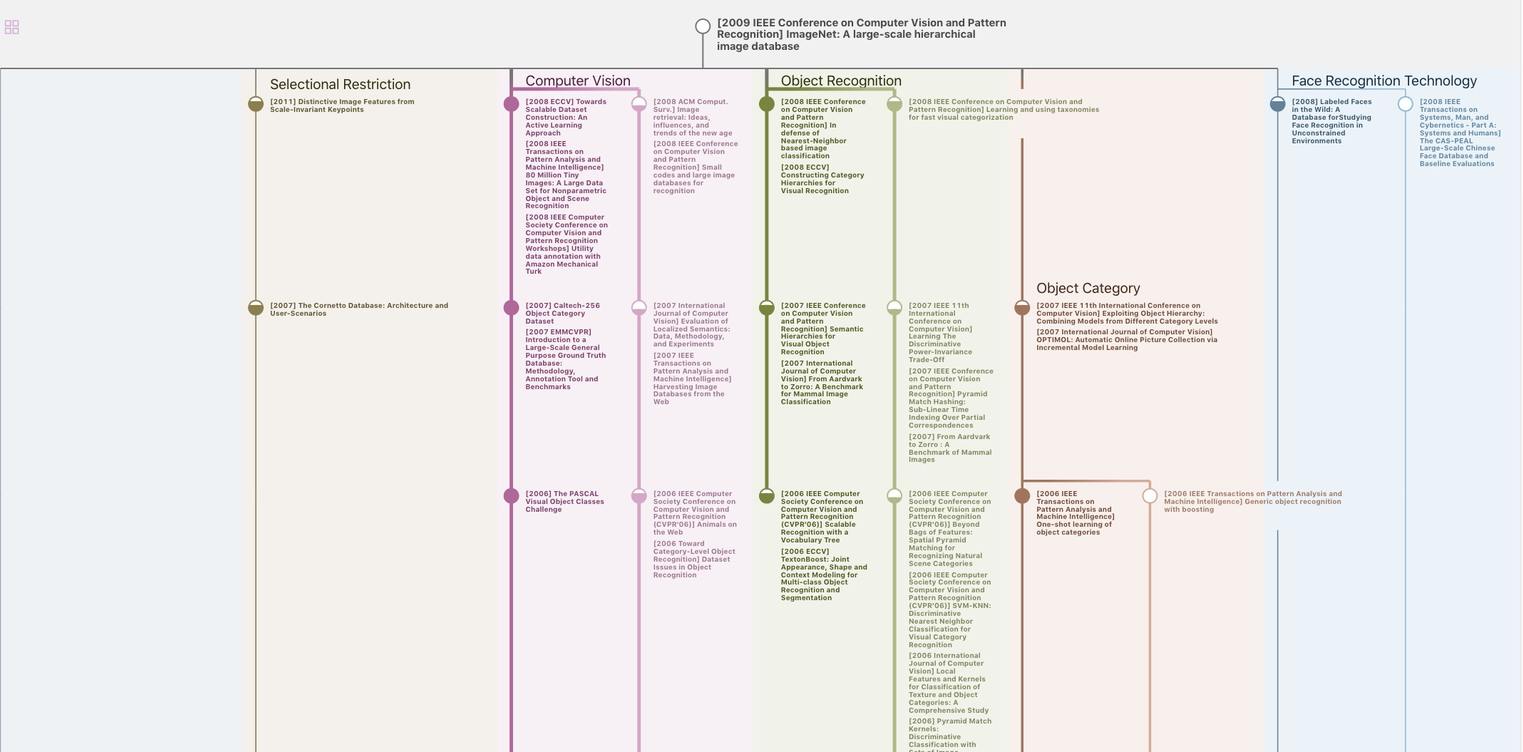
生成溯源树,研究论文发展脉络
Chat Paper
正在生成论文摘要