Multi-view Learning for Early Prognosis of Academic Performance: A Case Study
IEEE Transactions on Learning Technologies(2019)
摘要
Educational data mining has gained a lot of attention among scientists in recent years and constitutes an efficient tool for unraveling the concealed knowledge in educational data. Recently, semisupervised learning methods have been gradually implemented in the educational process demonstrating their usability and effectiveness. Cotraining is a representative semisupervised method aiming to exploit both labeled and unlabeled examples, provided that each example is described by two features views. Nevertheless, it is yet to be used in various scientific fields, among which the educational field as well, since the assumption about the existence of two feature views cannot be easily put into practice. Within this context, the main purpose of this study is to evaluate the efficiency of a proposed cotraining method for early prognosis of undergraduate students’ performance in the final examinations of a distance course based on a plethora of attributes which are naturally divided into two distinct views, since they are originated from different sources. More specifically, the first view consists of attributes regarding students’ characteristics and academic achievements which are manually filled out by their tutors, whereas the second one consists of attributes tracking students’ online activity in the course learning management system and which are automatically recorded by the system. The experimental results demonstrate the superiority of the proposed cotraining method as opposed to state-of-the-art semisupervised and supervised methods.
更多查看译文
关键词
Predictive models,Prognostics and health management,Data mining,Tools,Task analysis,Training,Radio frequency
AI 理解论文
溯源树
样例
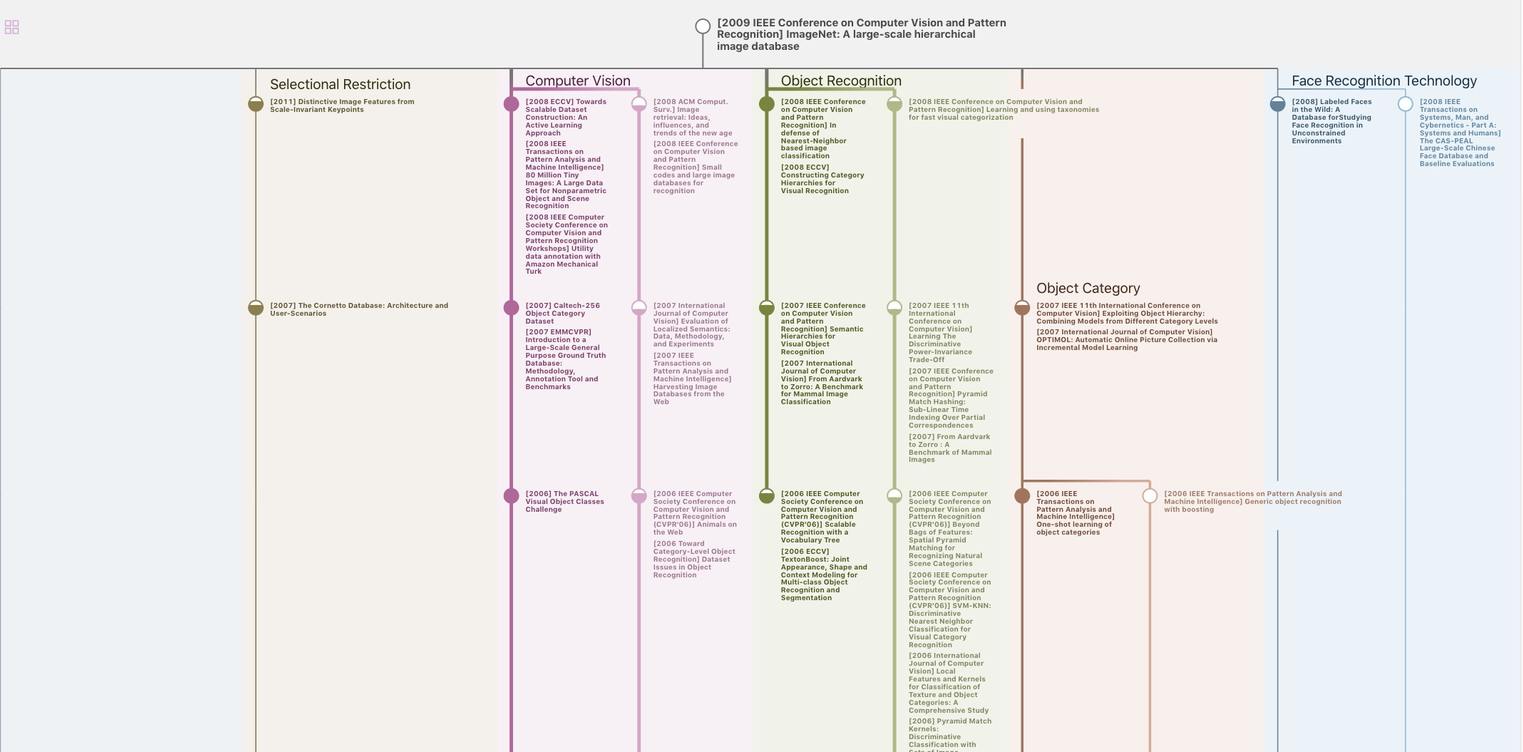
生成溯源树,研究论文发展脉络
Chat Paper
正在生成论文摘要