From Tv-L-1 To Gated Recurrent Nets
2019 IEEE INTERNATIONAL CONFERENCE ON ACOUSTICS, SPEECH AND SIGNAL PROCESSING (ICASSP)(2019)
Abstract
TV-L-1 is a classical diffusion-reaction model for low-level vision tasks, which can be solved by a duality based iterative algorithm. Considering the recent success of end-to-end learned representations, we propose a TV-LSTM network to unfold the duality based iterations into long short-term memory (LSTM) cells. To provide a trainable network, we relax the difference operators in the gate and cell update of TV-LSTM to trainable parameters. Then, the proposed end-to-end trainable TV-LSTMs can be naturally connected with various task-specific networks, e.g., optical flow estimation and image decomposition. Extensive experiments on optical flow estimation and structure + texture decomposition have demonstrated the effectiveness and efficiency of the proposed method.
MoreTranslated text
Key words
total variation, optical flow, recurrent neural network, image decomposition
AI Read Science
Must-Reading Tree
Example
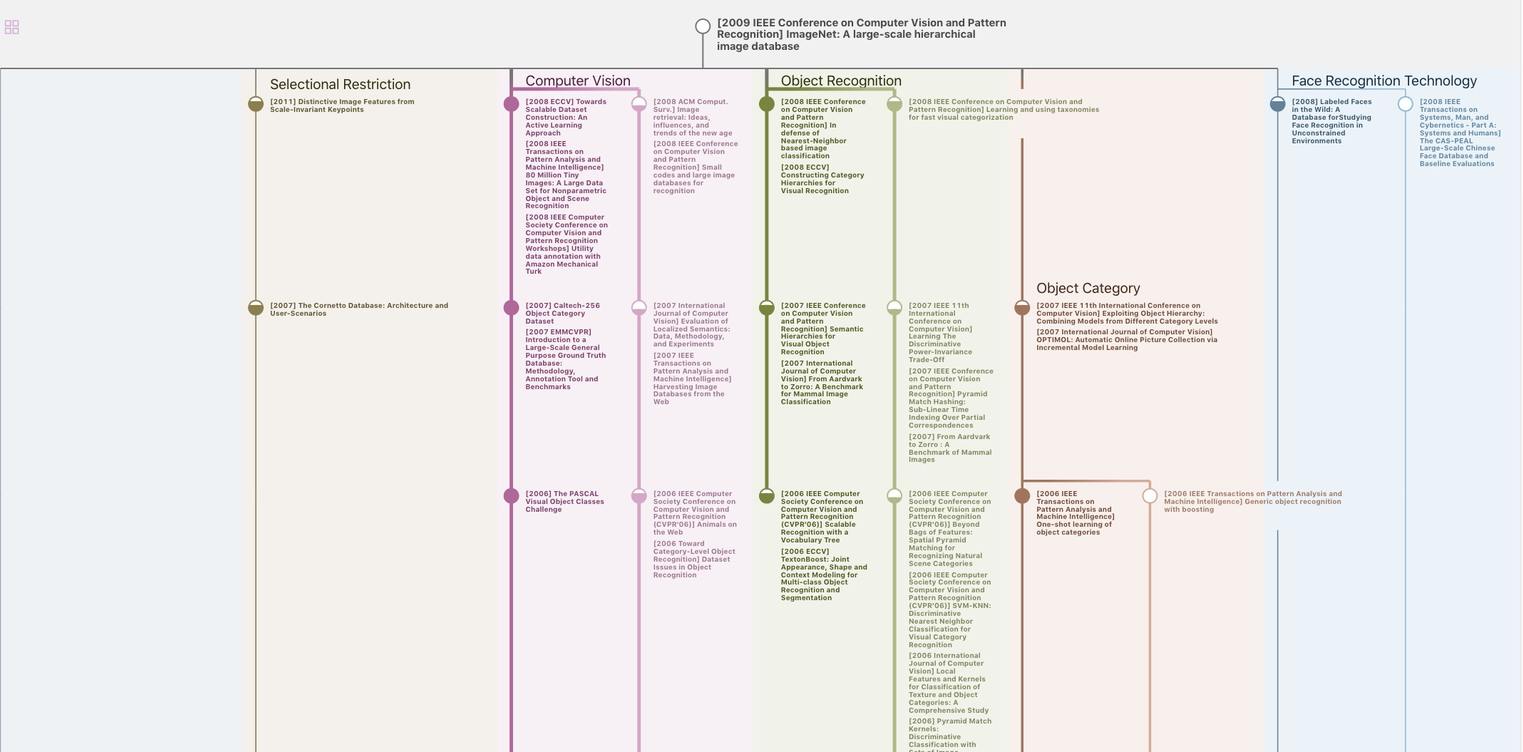
Generate MRT to find the research sequence of this paper
Chat Paper
Summary is being generated by the instructions you defined