AggregationNet: Identifying Multiple Changes Based on Convolutional Neural Network in Bitemporal Optical Remote Sensing Images
pacific-asia conference on knowledge discovery and data mining(2019)
摘要
The detection of multiple changes (i.e., different change types) in bitemporal remote sensing images is a challenging task. Numerous methods focus on detecting the changing location while the detailed “from-to” change types are neglected. This paper presents a supervised framework named AggregationNet to identify the specific “from-to” change types. This AggregationNet takes two image patches as input and directly output the change types. The AggregationNet comprises a feature extraction part and a feature aggregation part. Deep “from-to” features are extracted by the feature extraction part which is a two-branch convolutional neural network. The feature aggregation part is adopted to explore the temporal correlation of the bitemporal image patches. A one-hot label map is proposed to facilitate AggregationNet. One element in the label map is set to 1 and others are set to 0. Different change types are represented by different locations of 1 in the one-hot label map. To verify the effectiveness of the proposed framework, we perform experiments on general optical remote sensing image classification datasets as well as change detection dataset. Extensive experimental results demonstrate the effectiveness of the proposed method.
更多查看译文
关键词
Multiple change detection, Remote sensing, Aggregation network
AI 理解论文
溯源树
样例
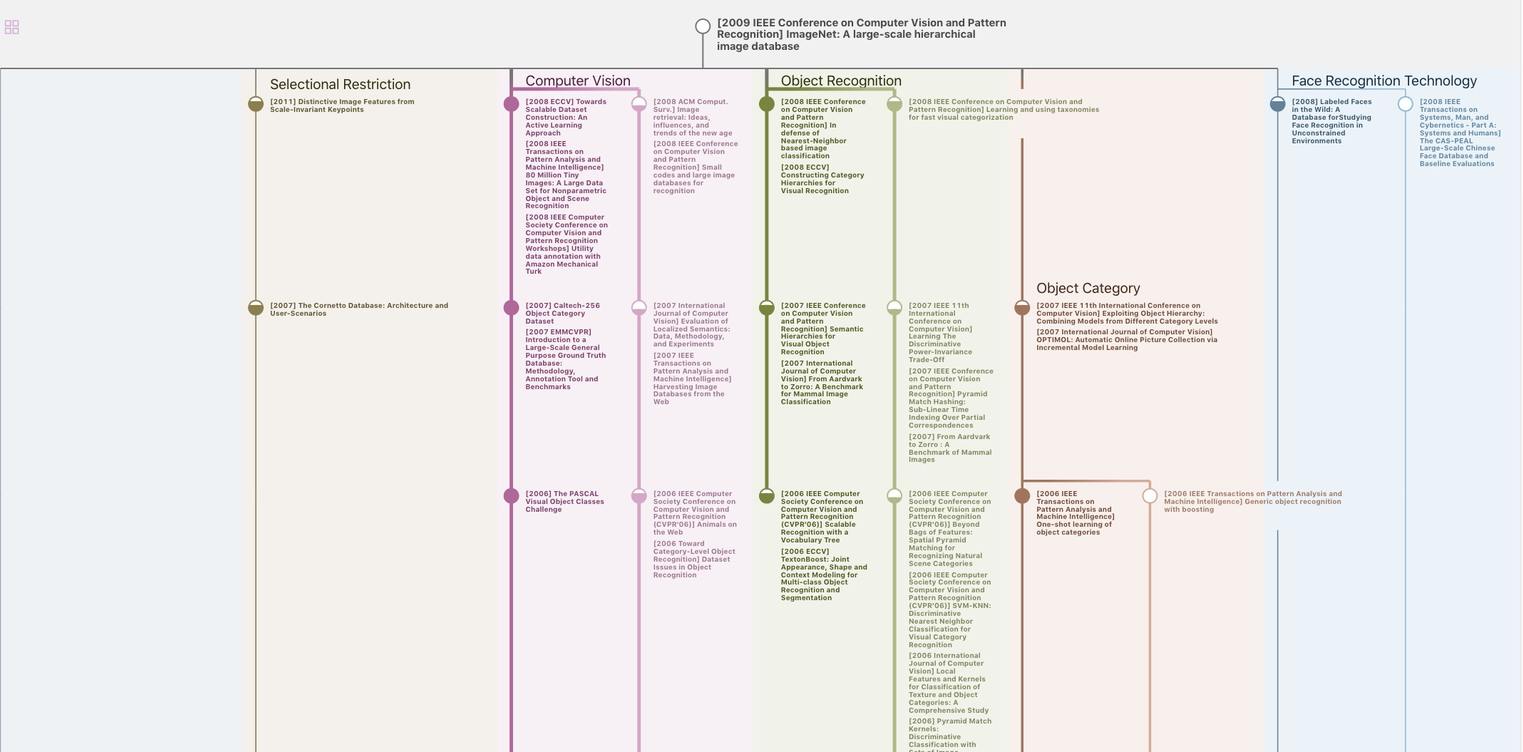
生成溯源树,研究论文发展脉络
Chat Paper
正在生成论文摘要