Walking without Friends: Publishing Anonymized Trajectory Dataset without Leaking Social Relationships
IEEE Transactions on Network and Service Management(2019)
摘要
Trajectory data has been widely collected via mobile devices and publicly released for academic research and commercial purposes. One primary concern of publishing such a dataset is the privacy issue. Previous protection schemes mainly focus on preventing re-identification attack, which utilizes the uniqueness of trajectories. However, the correlation between trajectories, which has not been given much attention to before, could also give rise to serious privacy leakage. Recent studies have proved that it is possible to identify social relationship, de-anonymize trajectories or even infer user’s locations by analyzing the correlation between users’ trajectories. We identify the serious privacy problem of social relationship leakage caused by what we call social relationship attack and aim to protect social relationship information, which cannot be protected by existing algorithms. We contribute to the design of a new privacy model and an effective system to deal with social relationship attack and re-identification attack simultaneously while maintaining high data utility. We propose a
SlidingWindow
algorithm to merge trajectories according to their
social-aware distance
, which concerns both the spatiotemporal distance and social proximity. Evaluations of two trajectory datasets under different scenarios demonstrate that our system provides more than 1.84 times privacy protection at the cost of only 2.5% data utility loss.
更多查看译文
关键词
Trajectory,Correlation,Privacy,Data privacy,Spatiotemporal phenomena,Publishing,Data models
AI 理解论文
溯源树
样例
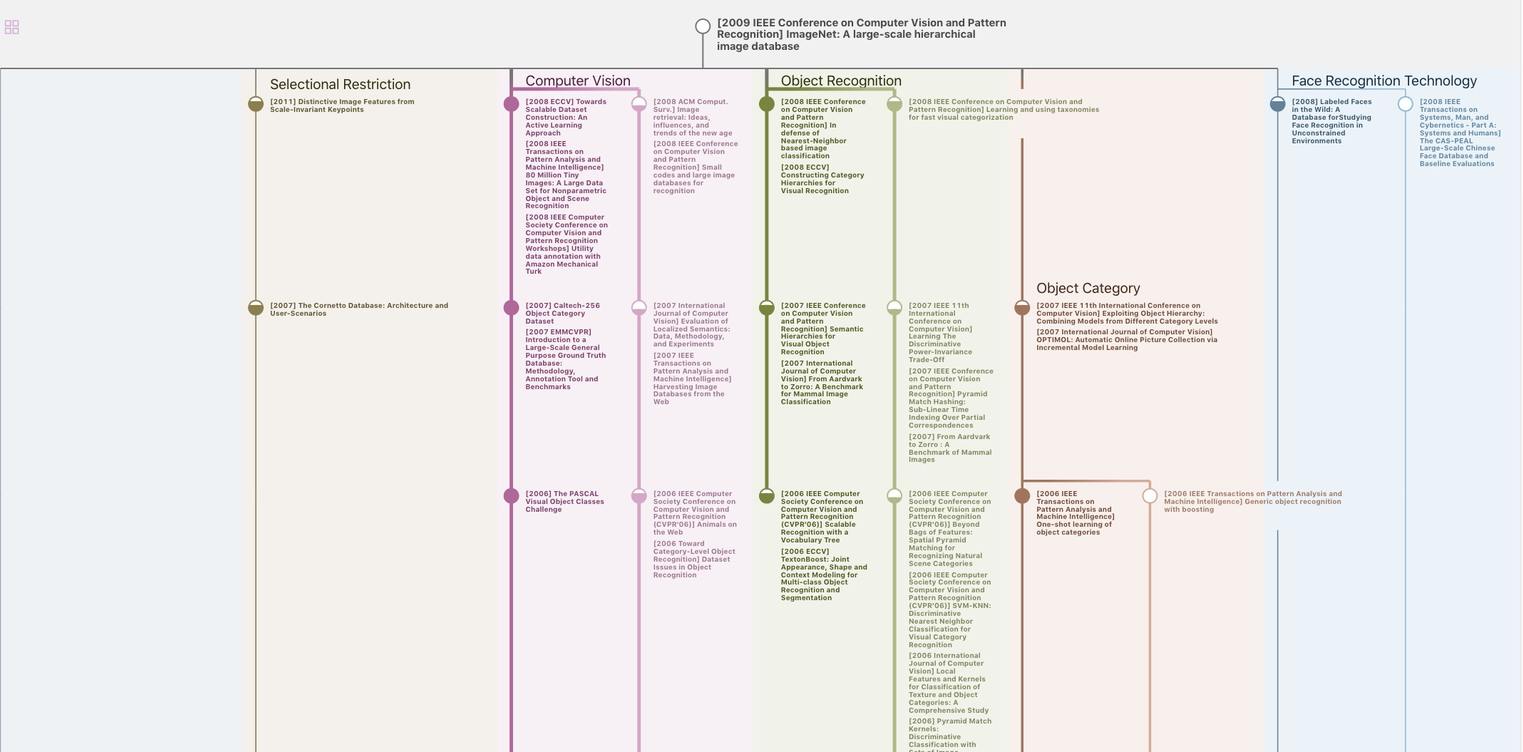
生成溯源树,研究论文发展脉络
Chat Paper
正在生成论文摘要