Edge guided compressive sensing for image reconstruction based on two-stage l0 minimization
Journal of Visual Communication and Image Representation(2019)
摘要
In compressive sensing framework, the results of image reconstruction are sometimes not accurate enough due to the downsampled measurements, especially when the sampling rate is relatively small. This paper proposes a novel edge guided compressive sensing (EGCS) algorithm for natural image reconstruction based on two-stage l0 minimization, aiming to improve the reconstruction performance. Firstly, wavelet transform is utilized to provide sparsity and multiple sampling scheme is employed to acquire the down-sampled measurements. Then, in the first stage, we design an edge-preserving smoothing method by l0 gradient minimization to extract the important edge prior accurately, which can not only contribute a lot to improve the reconstruction accuracy of image structures but also reduce the computational complexity remarkably. Also, the use of multiple sampling scheme is beneficial to enhancing the guidance accuracy of multiple edge prior. In the second stage, under the guidance of multiple edge prior, the intelligent searching strategies are designed by taking advantages of intelligent optimization algorithms in solving combinatorial optimization problems and utilizing the superior performance of greedy algorithm on reconstruction speed, which is conductive to solve the joint sparse reconstruction based on l0 minimization essentially. The better reconstruction performance can be achieved based on the fact that it is more likely to find the global optimal solution accurately by the designed intelligent searching strategies, especially when the sampling rate is relatively small. Experimental results on natural image reconstruction demonstrate that our proposed method EGCS is superior to the state-of-the-art reconstruction algorithms, and can well preserve the important image structures at the same time.
更多查看译文
关键词
Compressive sensing,Image reconstruction,l0 minimization,Edge prior,Multiple sampling scheme
AI 理解论文
溯源树
样例
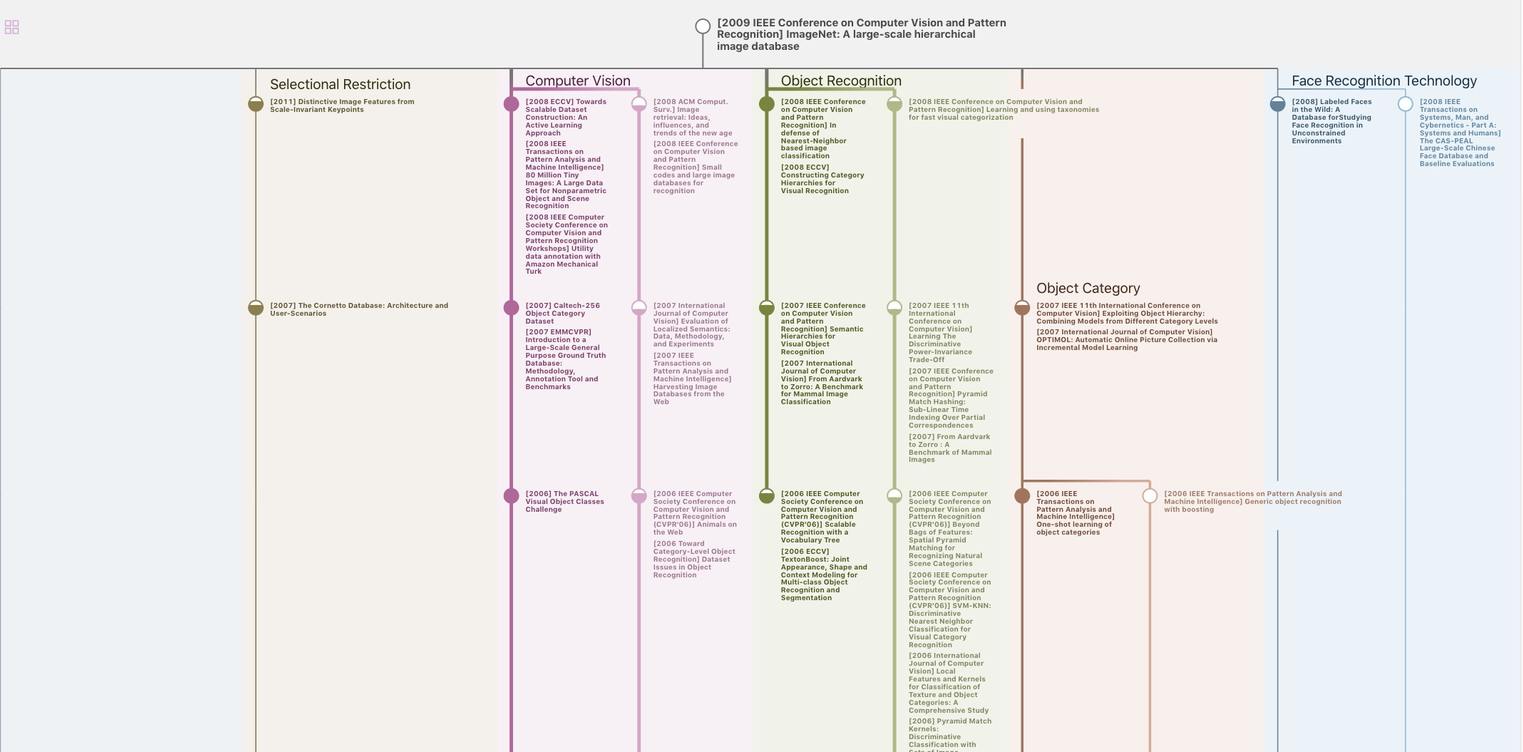
生成溯源树,研究论文发展脉络
Chat Paper
正在生成论文摘要