Deep convolutional BiLSTM fusion network for facial expression recognition
The Visual Computer(2019)
摘要
Deep learning algorithms have shown significant performance improvements for facial expression recognition (FER). Most deep learning-based methods, however, focus more attention on spatial appearance features for classification, discarding much useful temporal information. In this work, we present a novel framework that jointly learns spatial features and temporal dynamics for FER. Given the image sequence of an expression, spatial features are extracted from each frame using a deep network, while the temporal dynamics are modeled by a convolutional network, which takes a pair of consecutive frames as input. Finally, the framework accumulates clues from fused features by a BiLSTM network. In addition, the framework is end-to-end learnable, and thus temporal information can be adapted to complement spatial features. Experimental results on three benchmark databases, CK+, Oulu-CASIA and MMI, show that the proposed framework outperforms state-of-the-art methods.
更多查看译文
关键词
Facial expression recognition, Deep network, BiLSTM, Spatial–temporal features
AI 理解论文
溯源树
样例
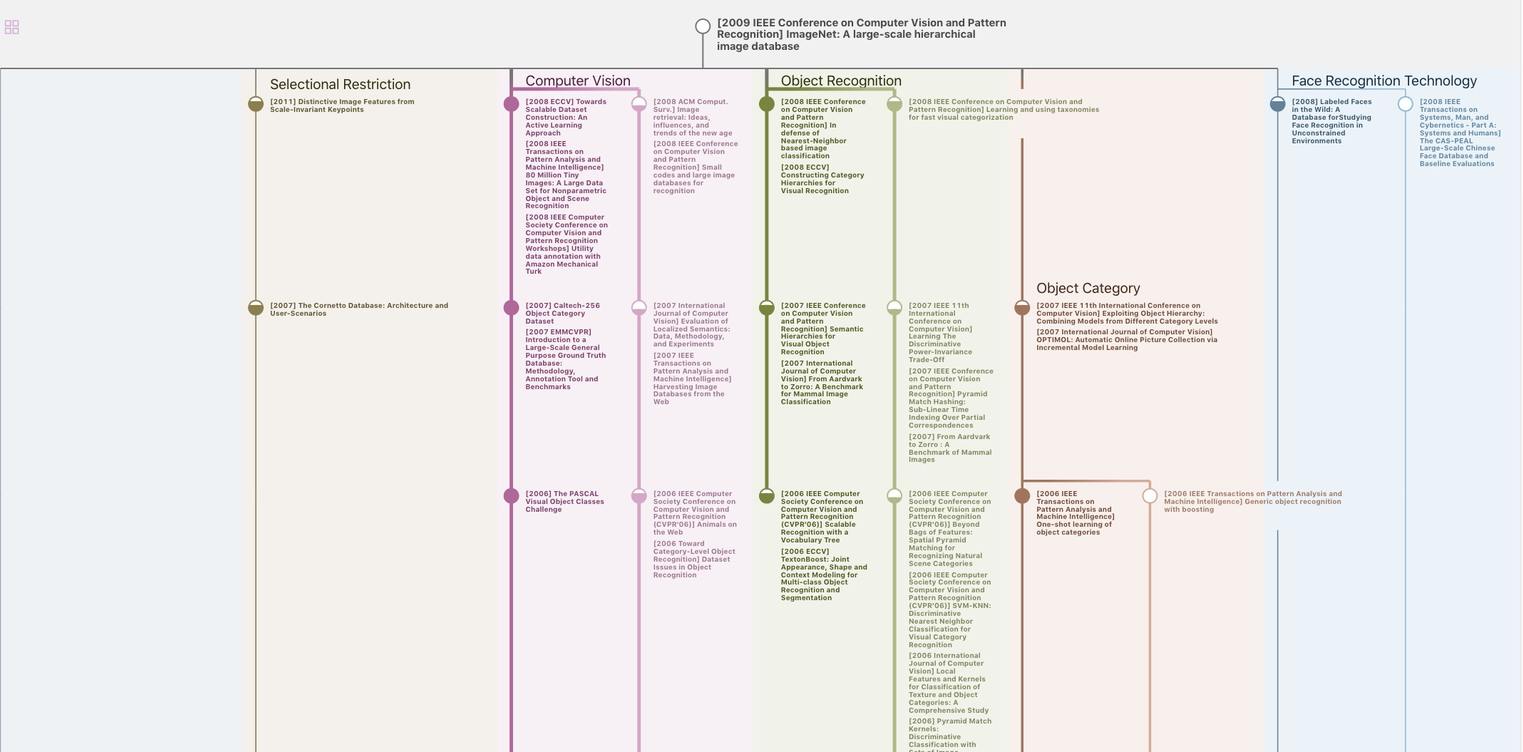
生成溯源树,研究论文发展脉络
Chat Paper
正在生成论文摘要