Smoking causes early biological aging: a deep neural network analysis of common blood test results
EUROPEAN RESPIRATORY JOURNAL(2018)
摘要
Introduction: Aging is a key determinant of death, disease, and health costs. Currently, there are no clinically available methods to measure the effects of smoking on biological aging. Aim: To assess differences in biological aging between smokers and nonsmokers using deep neural network modelling of common blood test results. Methods: With IRB approval 20 715 883 anonymized blood test results from 149 000 subjects (49 000 smokers) were used to select relevant feature spaces using multifactorial adaptive arbitrage models. Feature spaces were constructed using same day results and missing values imputed using applied linear regression. Age prediction was assessed using the Pearson correlation coefficient (r), the standard coefficient of determination (R2), and the mean absolute error (MAE) from Random Forest models of selected distinct feature spaces first training with nonsmokers, and then analyzing both smokers and nonsmokers. Results: The best model used 24 features (including smoking status) with r = 0.78, R2 = 0.60, ε-accuracy = 82%, and MAE = 5.42 years. Fasting glucose, gender and erythrocyte distribution width were most predictive of biological aging. Smoking status improved aging prediction, but was not amongst the top five predictors. Smokers biologically aged faster than nonsmokers regardless of cholesterol and fasting glucose levels. Female and male smokers aged twice and one and a half times faster than gender-matched nonsmokers. Increased biological aging compared to nonsmokers plateaued after age 55 years. Conclusion: Smoking increased biological aging up to age 55 years, more so in women, and regardless of fasting blood glucose or serum cholesterol values.
更多查看译文
关键词
early biological aging,smoking,deep neural network analysis,deep neural network
AI 理解论文
溯源树
样例
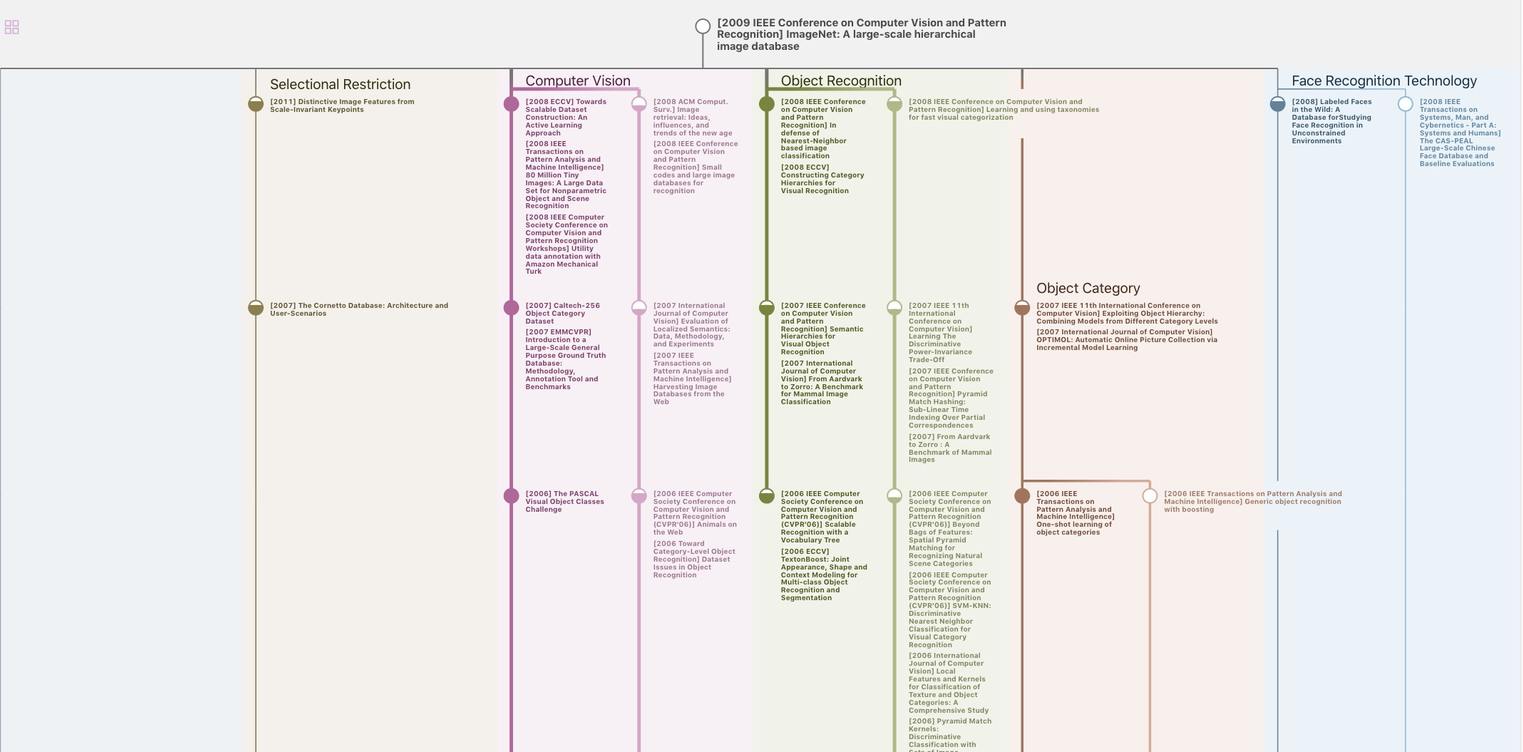
生成溯源树,研究论文发展脉络
Chat Paper
正在生成论文摘要