Fault detection based on robust characteristic dimensionality reduction
Control Engineering Practice(2019)
摘要
In this paper, a novel fault detection method is developed based on robust characteristic dimensionality reduction (RCDR). The time-constrained sparse representation (TCSR) method is firstly introduced by considering the space and time characteristics of industrial process monitoring data simultaneously. It can remove space-related outliers, time-related outliers and noises by solving an optimization problem. Then, a new RCDR method is proposed, which fully utilizes the constructed robust adjacency graph and considers the data characteristics. Its scatter matrices are specially designed by consideration of the data characteristics of fault detection. The within-class scatter matrix only characterizes normal data set with a classic covariance matrix, while the inter-class scatter matrix characterizes the separability between normal data and fault data through a pre-defined scatter matrix. It is worth mentioning that our method does not make Gaussian assumptions about the distribution of the fault data, and the number of projection directions is not limited as well. The TCSR is also embedded into our proposed dimensionality reduction method, enabling it to handle the fault detection problem under strong disturbances. Simulations on Tennessee Eastman process (TEP) and a case study of electric multiple unit (EMU) braking system of high-speed trains fully demonstrate the effectiveness and applicability of our proposed fault detection method.
更多查看译文
关键词
Fault detection,Time-constrained sparse representation (TCSR),Robust characteristic dimensionality reduction (RCDR),Tennessee eastman process (TEP),Electric multiple unit (EMU) braking system
AI 理解论文
溯源树
样例
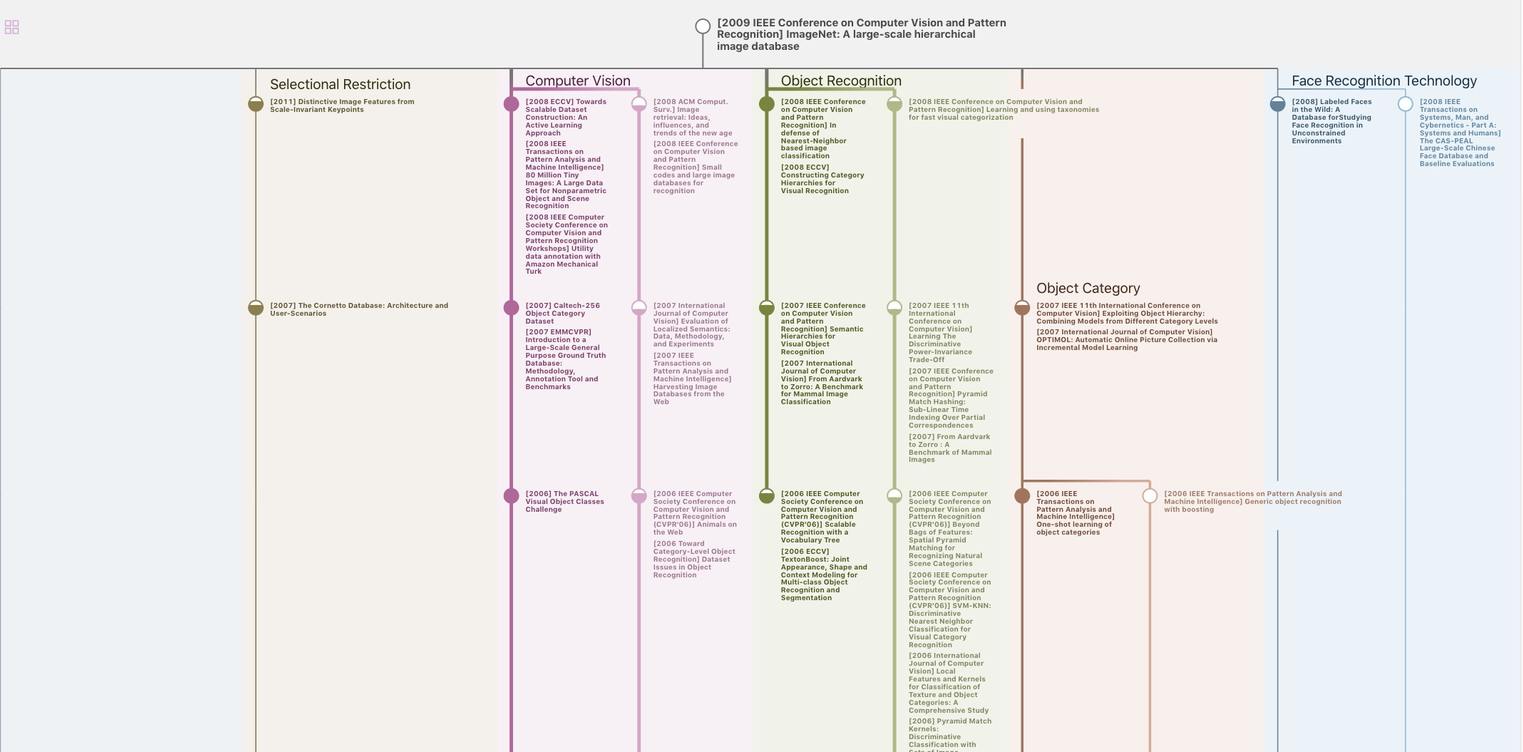
生成溯源树,研究论文发展脉络
Chat Paper
正在生成论文摘要