An Evaluation of Design-based Properties of Different Composite Estimators
arXiv: Methodology(2018)
摘要
For the last several decades, the US Census Bureau has been using the AK composite estimation method to produce statistics on employment from the Current Population Survey (CPS) data. The CPS uses a rotating design and AK estimators are linear combinations of monthly survey weighted averages (called month-in-sample estimates) in each rotation groups. Denoting by X the vector of month-in-sample estimates and by Σ its design based variance, the coefficients of the linear combination were optimized by the Census Bureau after substituting Σ by an estimate and under unrealistic stationarity assumptions. To show the limits of this approach, we compared the AK estimator with different competitors using three different synthetic populations that mimics the Current Population Survey (CPS) data and a simplified sample design that mimics the CPS design. In our simulation setup, empirically best estimators have larger mean square error than simple averages. In the real data analysis, the AK estimates are constantly below the survey-weighted estimates, indicating potential bias. Any attempt to improve on the estimated optimal estimator in either class would require a thorough investigation of the highly non-trivial problem of estimation of Σ for a complex setting like the CPS (we did not entertain this problem in this paper). A different approach is to use a variant of the regression composite estimator used by Statistics Canada. The regression composite estimator does not require estimation of Σ and is less sensitive to the rotation group bias in our simulations. Our study demonstrates that there is a great potential for improving the estimation of levels and month to month changes in the unemployment rates by using the regression composite estimator.
更多查看译文
关键词
calibration,estimated controls,longitudinal survey,labor force statistics
AI 理解论文
溯源树
样例
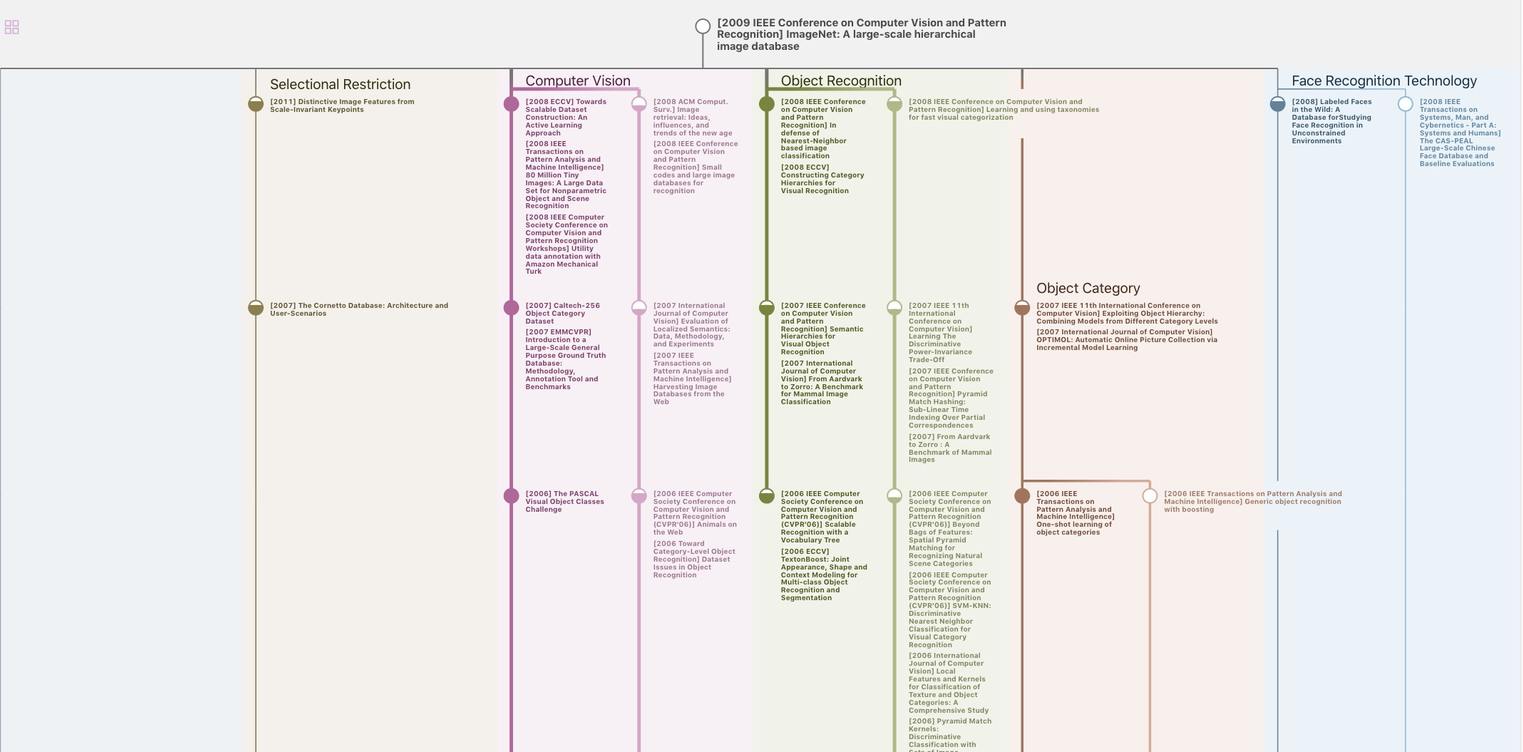
生成溯源树,研究论文发展脉络
Chat Paper
正在生成论文摘要