Weekly cycle assessment of PM mass concentrations and sources, and impacts on temperature and wind speed in Southern Italy
Atmospheric Research(2019)
摘要
A methodology to detect the weekly cycle impact of the particulate matter (PM), and PM sources on the near surface temperature and wind speed is discussed in the paper. Chemically-speciated PM10 and PM2.5 samples are analyzed to detect the weekly cycle of both the PM mass concentrations and the PM sources identified by the Positive Matrix Factorization technique. The average percent departure (APD) of the PM mass concentration from the mean value calculated for each day of the week shows that a positive (higher values during midweek) and a negative (higher values during weekend) weekly cycle characterizes the PM10 and PM2.5 mass concentrations in Autumn-Winter (AW, September–February) and Spring-Summer (SS, March–August), respectively. The westerly transport of pollution seems to have a role on the negative PM weekly cycle found in SS. The analysis of the six identified aerosol sources indicates that in SS the mixed anthropogenic and the reacted dust sources likely impact the PM10 negative weekly cycle and that the mixed anthropogenic source likely impacts the PM2.5 negative weekly cycle. The mixed anthropogenic and soil dust sources likely affect in AW the positive weekly cycle of the PM10 mass concentration. Both sources in addition to the reacted dust source seem to affect the PM2.5 mass concentration in AW. The APD analysis of the temperature (T) and wind speed (WS) at the surface from measurements co-located in space and time with the PM ones reveals that the WS and T values are characterized by a negative weekly cycle in AW. Conversely, in SS, the WS-APD value decreases on Sunday and the T-APD values increase in the second half of the week. These last results likely give evidence of the PM impact on the near-surface temperature and wind speed at the study site.
更多查看译文
关键词
Weekly cycles,PM mass concentration,Temperature and wind speed,Positive Matrix Factorization,Long-range transport
AI 理解论文
溯源树
样例
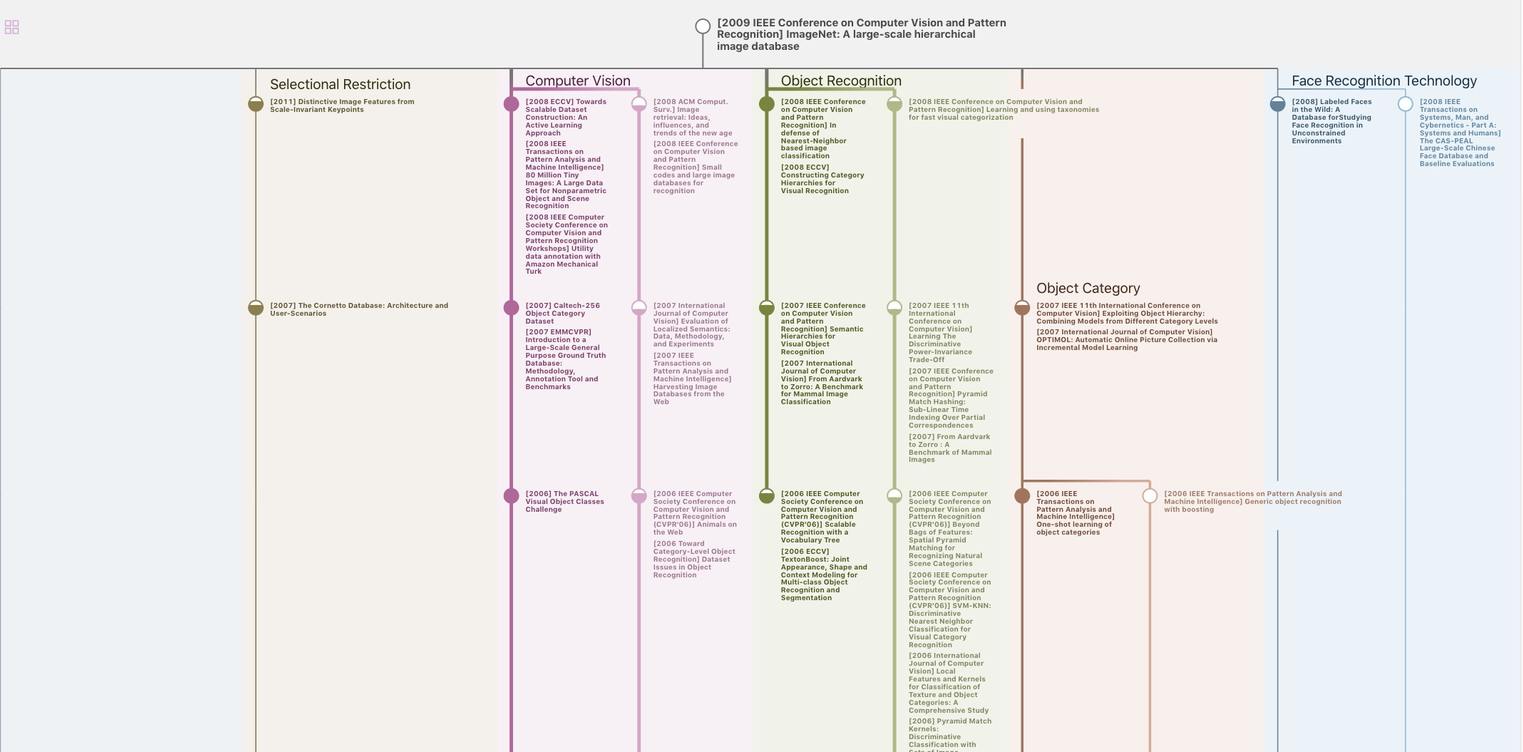
生成溯源树,研究论文发展脉络
Chat Paper
正在生成论文摘要