Scalable Detection of Crowd Motion Patterns
IEEE Transactions on Knowledge and Data Engineering(2020)
摘要
Studying the movements of crowds is important for understanding and predicting the behavior of large groups of people. When analyzing crowds, one is often interested in the long-term macro-level motions of the crowd as a whole, as opposed to the micro-level short-term movements of individuals. A high-level representation of these motions is thus desirable. In this work, we present a scalable method for detection of
crowd motion patterns
, i.e., spatial areas describing the dominant motions within crowds. For measuring crowd movements, we propose a fast, scalable, and low-cost method based on proximity graphs. For analyzing crowd movements, we utilize a three-stage pipeline: (1) represents the behavior of each person at each moment in time as a low-dimensional data point, (2) cluster these data points based on spatial relations, and (3) concatenate these clusters based on temporal relations. Experiments on synthetic datasets reveals our method can handle various scenarios including curved lanes and diverging flows. Evaluation on real-world datasets shows our method is able to extract useful motion patterns which could not be properly detected by existing methods. Overall, we see our work as an initial step towards rich pattern recognition.
更多查看译文
关键词
Sensors,Trajectory,Pipelines,Data collection,Cameras,Target tracking
AI 理解论文
溯源树
样例
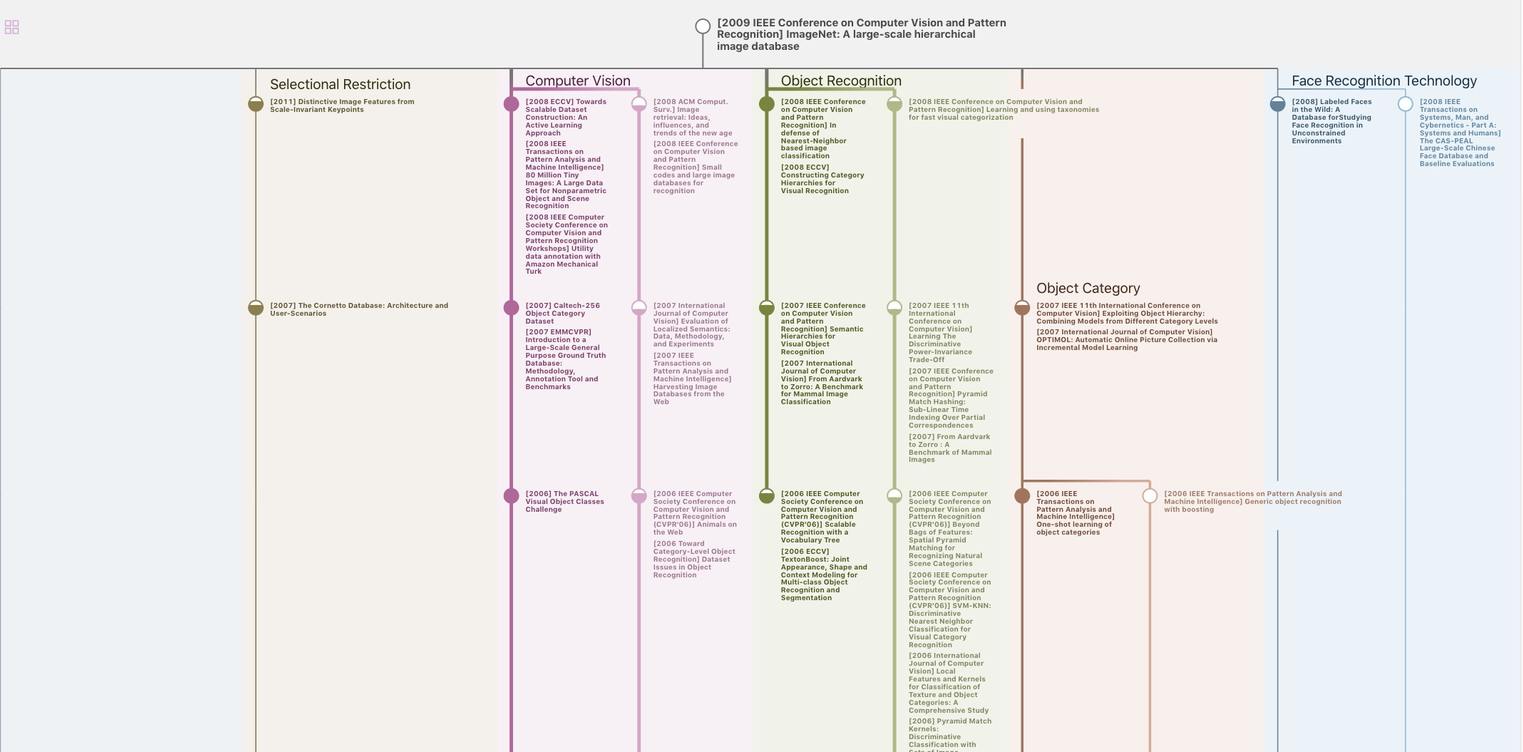
生成溯源树,研究论文发展脉络
Chat Paper
正在生成论文摘要