Improving Nuclei Classification Performance in H&E Stained Tissue Images Using Fully Convolutional Regression Network and Convolutional Neural Network
2018 IEEE Applied Imagery Pattern Recognition Workshop (AIPR)(2018)
摘要
Detection and classification of nuclei in histopathology images is an important step in the research of understanding tumor microenvironment and evaluating cancer progression and prognosis. The task is challenging due to imaging factors such as varying cell morphologies, batch-to-batch variations in staining, and sample preparation. We present a two-stage deep learning pipeline that combines a Fully Convolutional Regression Network (FCRN) that performs nuclei localization with a Convolution Neural Network (CNN) that performs nuclei classification. Instead of using hand-crafted features, the system learns the visual features needed for detection and classification of nuclei making the process robust to the aforementioned variations. The performance of the proposed system has been quantitatively evaluated on images of hematoxylin and eosin (H&E) stained colon cancer tissues and compared to the previous studies using the same data set. The proposed deep learning system produces promising results for detection and classification of nuclei in histopathology images.
更多查看译文
关键词
deep learning,fully convolutional regression network,nucleus classification,nucleus detection,histopathology image analysis.
AI 理解论文
溯源树
样例
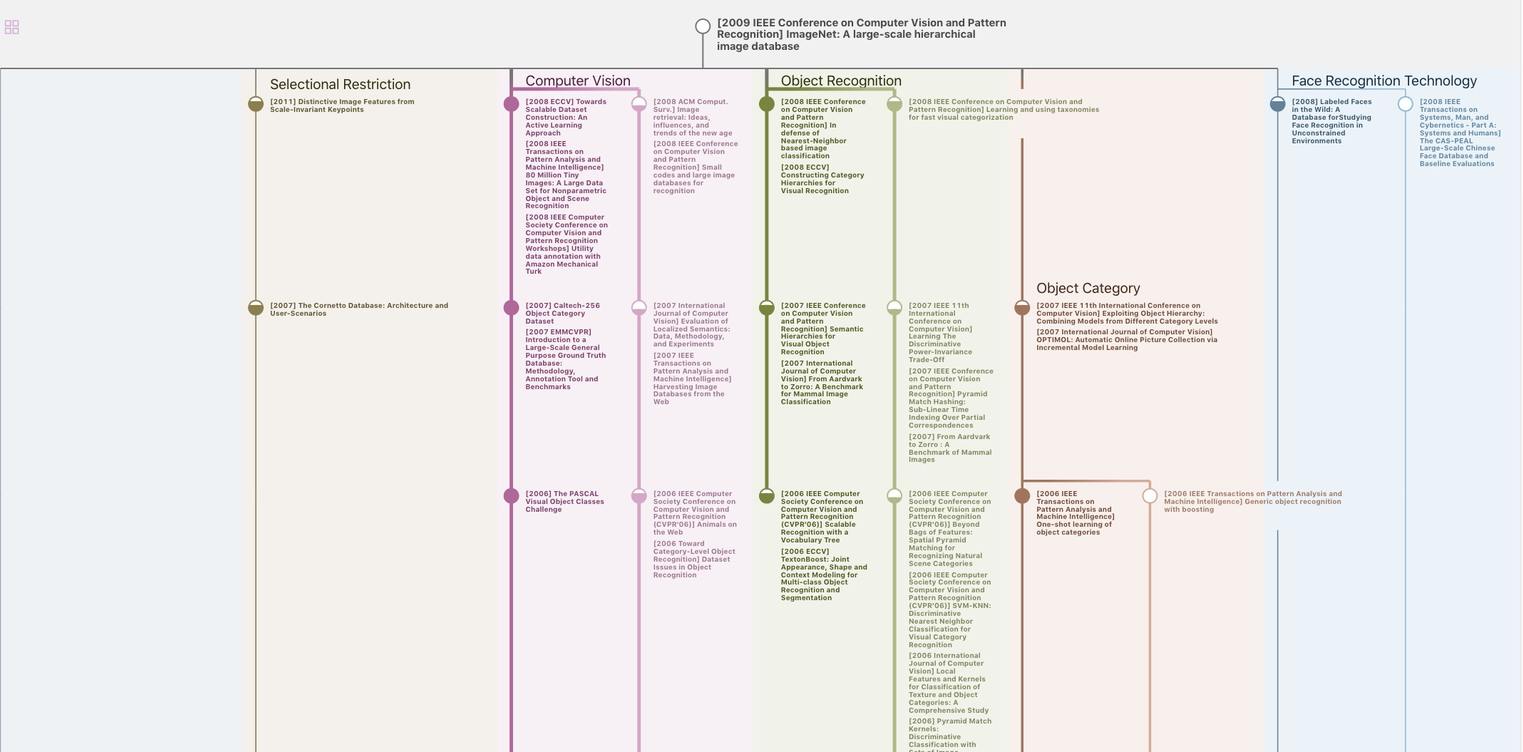
生成溯源树,研究论文发展脉络
Chat Paper
正在生成论文摘要